Underwater Multitarget Tracking With Sonar Images Using Thresholded Sequential Monte Carlo Probability Hypothesis Density Algorithm
IEEE GEOSCIENCE AND REMOTE SENSING LETTERS(2022)
摘要
This letter presents a multitarget tracking algorithm-thresholded sequential Monte Carlo probability hypothesis density (TH-SMC-PHD) algorithm. The TH-SMC-PHD aims to overcome the problem that underwater multitarget tracking is prone to missing tracking on sonar images, resulting in the breakage of trajectories. First, cell average (CA)-constant false alarm rate (CFAR) and K-means are employed to detect potential underwater targets from sonar images, respectively. Then, the TH-SMC-PHD is applied to the detection results for tracking, using a continuously lost frame threshold to reduce the missing tracking rate (MR) and the minimum-sampling-variance (MSV) resampling to improve tracking accuracy. An actual underwater multitarget tracking experiment using a forward-looking sonar was contracted in a water tank to evaluate the tracking performance. Compared with the other two PHD tracking algorithms, the results demonstrate that the proposed algorithm achieves high-precision, non-fracture, non-missing tracking of three targets. In addition, the TH-SMC-PHD is more stable and less affected by different detection algorithms, which has the potential for practical applications.
更多查看译文
关键词
Target tracking,Sonar detection,Object detection,Detection algorithms,Prediction algorithms,Particle measurements,Atmospheric measurements,Sonar images,thresholded sequential Monte Carlo probability hypothesis density (TH-SMC-PHD) algorithm,underwater multitarget tracking
AI 理解论文
溯源树
样例
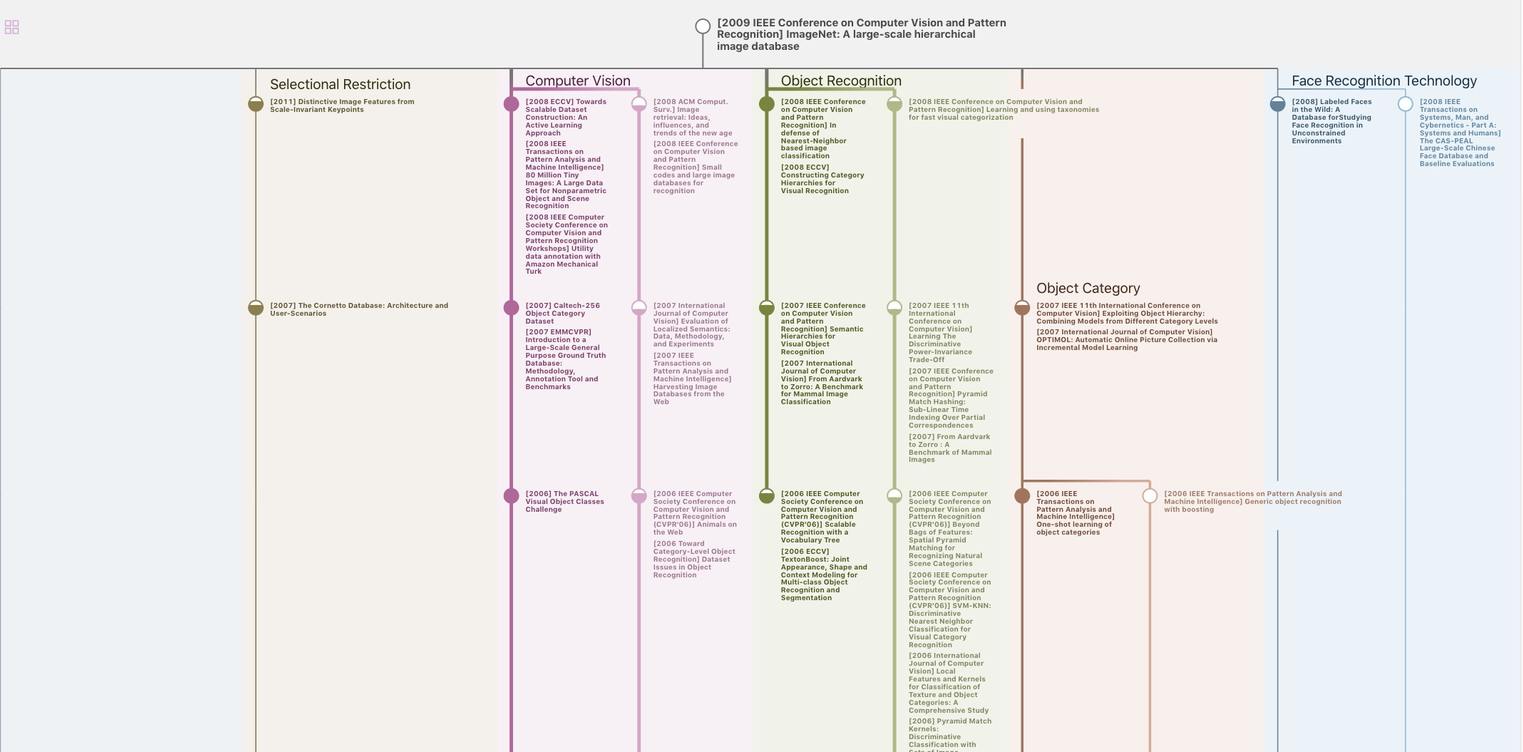
生成溯源树,研究论文发展脉络
Chat Paper
正在生成论文摘要