DDMA: Discrepancy-Driven Multi-agent Reinforcement Learning.
Pacific Rim International Conference on Artificial Intelligence (PRICAI)(2022)
摘要
Multi-agent reinforcement learning algorithms depend on quantities of interactions with the environment and other agents to derive an approximately optimal policy. However, these algorithms may struggle in the complex interactive relationships between agents and tend to explore the whole observation space aimlessly, which results in high learning complexity. Motivated by the occasional and local interactions between multiple agents in most real-world scenarios, in this paper, we propose a general framework named Discrepancy-Driven Multi-Agent reinforcement learning (DDMA) to help overcome this limitation. In this framework, we first parse the semantic components of each agent's observation and introduce a proliferative network to directly initialize the multi-agent policy with the corresponding single-agent optimal policy, which bypasses the misalignment of observation spaces in different scenarios. Then we model the occasional interactions among agents based on the discrepancy between these two policies, and conduct more focused exploration on these areas where agents interact frequently. With the direct initialization and the focused multi-agent policy learning, our framework can help accelerate the learning process and promote the asymptotic performance significantly. Experimental results on a toy example and several classic benchmarks demonstrate that our framework can obtain superior performance compared to baseline methods.
更多查看译文
关键词
Multi agent,Reinforcement learning,Exploration
AI 理解论文
溯源树
样例
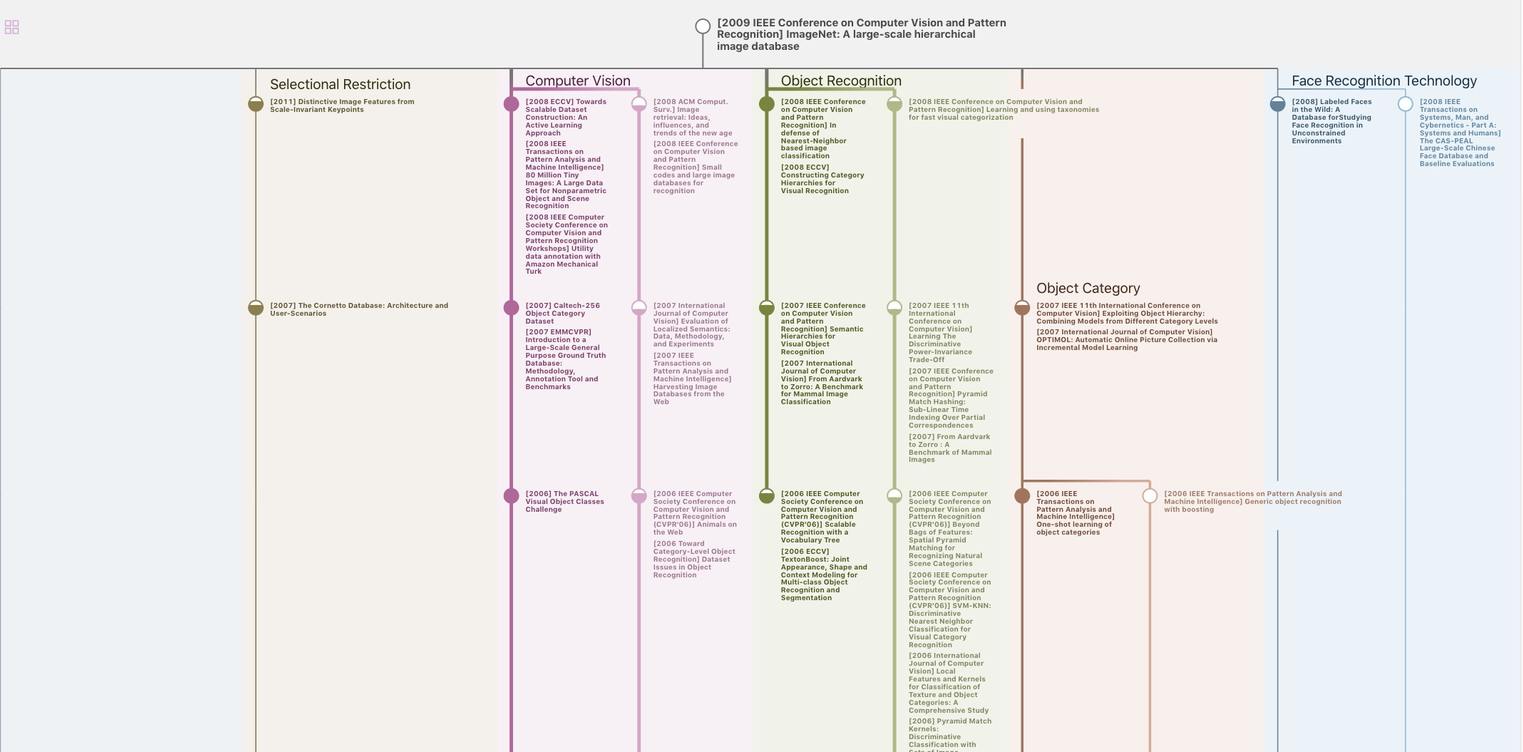
生成溯源树,研究论文发展脉络
Chat Paper
正在生成论文摘要