CooccurrenceAffinity: An R package for computing a novel metric of affinity in co-occurrence data that corrects for pervasive errors in traditional indices
biorxiv(2022)
摘要
Analysis of co-occurrence data in ecology and biogeography with traditional indices has led to many problems. In our recent study (Mainali, Slud, Singer, & Fagan, 2022), we revealed the source of the problem that makes the traditional indices fundamentally flawed and completely unreliable, and we further developed a novel metric of association, alpha, with complete formulation of the null distribution for estimating the mechanism of affinity. We also developed the maximum likelihood estimate (MLE) of alpha in our prior study. Here, we introduce the CooccurrenceAffinity R package that computes alpha MLE. We provide functions to perform the analysis based on a 2x2 contingency table of occurrence/co-occurrence counts as well as a mxn presence-absence matrix (e.g., species by site matrix). The flexibility of the function allows a user to compute the alpha MLE for entity pairs on matrix columns based on presence-absence states recorded in the matrix rows, or for entities on matrix rows based on presence-absence recorded in columns. We also provide functions for plotting the computed indices. As novel components of this software paper not reported in the original study, we present theoretical discussions about median interval and four types of confidence intervals; we further develop functions (a) to compute those intervals, (b) to evaluate their true coverage probability of enclosing the population parameter, and (c) to generate figures. CooccurrenceAffinity is a practical and efficient R package with user-friendly functions for end-to-end analysis and plotting of co-occurrence data in various formats, making it possible to compute the recently developed metric of alpha MLE as well as its median and confidence intervals introduced in this paper. The package supplements its main output of the novel metric of association with the three most common traditional indices of association in co-occurrence data: Jaccard, Sorensen-Dice, and Simpson.
### Competing Interest Statement
The authors have declared no competing interest.
更多查看译文
关键词
cooccurrenceaffinity,novel metric,indices,co-occurrence
AI 理解论文
溯源树
样例
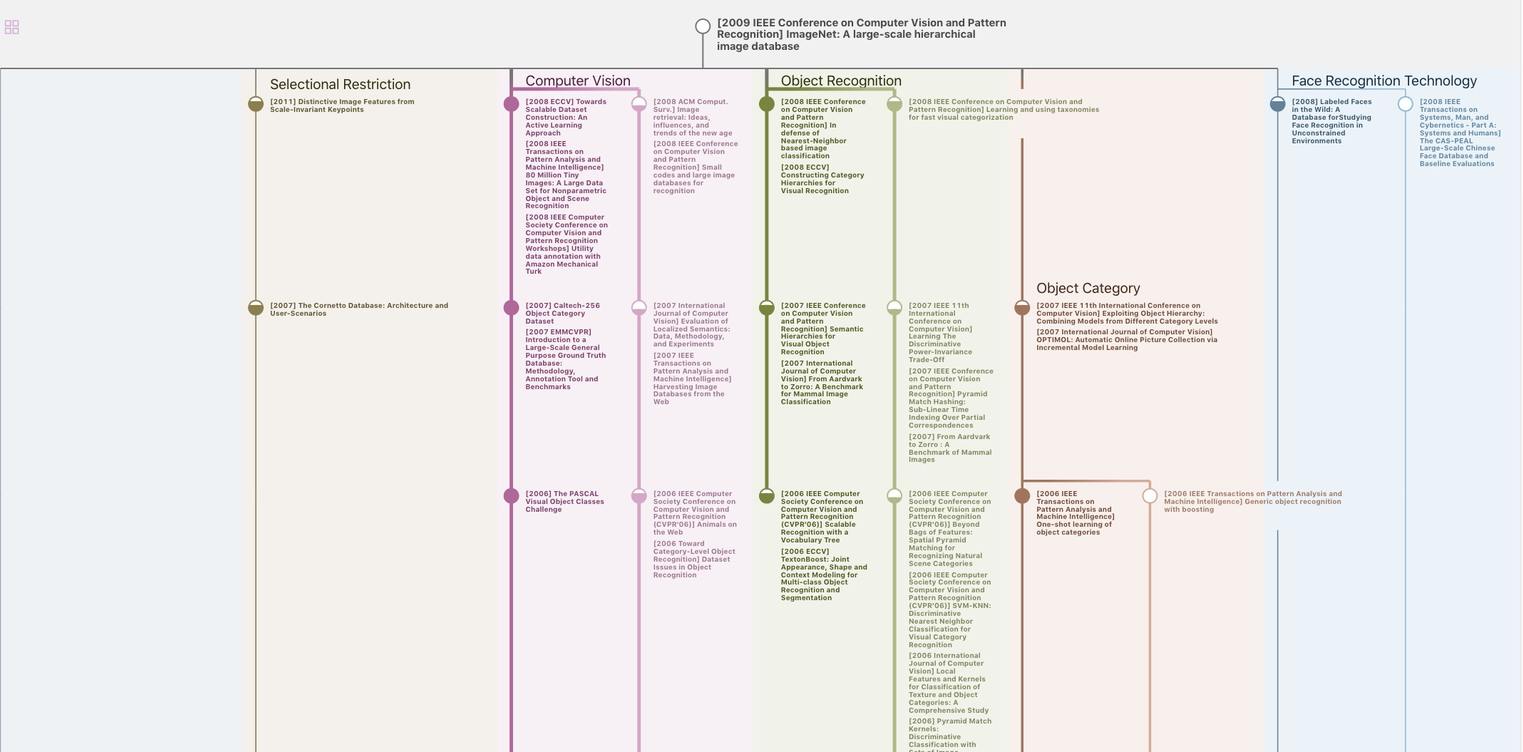
生成溯源树,研究论文发展脉络
Chat Paper
正在生成论文摘要