Real-world Underwater Image Enhancement via Degradation-aware Dynamic Network.
Pacific Rim International Conference on Artificial Intelligence (PRICAI)(2022)
摘要
Underwater degradations are various and sometimes scene-dependent such as different kinds of color distortion and different extent of fuzziness, attributed to complicated underwater environments. Recently methods produce promising restoration results, however, they are time consuming and introduce suboptimal results when facing complicated scenes. Therefore, the two main challenges are how to maintain fast and generic processing when tackling complicated scenes. To address these problems, we develop a degradation-aware dynamic network named DD-Net by introducing the Koschmieder's light scattering model. Specifically, to handle various color distortions, we propose a scene-adaptive convolution, the basic element of which is no longer a float point number, but a function. The function outputs scene-adaptive parameters of the convolutions by taking the estimated scene factor of the underwater image as input, accordingly. In addition, to handle different extents of fuzziness degradation, we proposed a novel haze-adaptive regularization. The regularization uses medium transmission maps to guide the model to implicitly assign different attention to regions suffering from various degrees of degradation. By fully utilizing the global information (i.e., the global background light) and the local information (i.e., medium transmission map), our proposed method outperforms previous methods in terms of quantitative metrics. More importantly, our model is implemented in a lightweight manner, providing the possibility of real-time restoration.
更多查看译文
关键词
Uderwater image enhancement,Degradation-aware,Dynamic neural network
AI 理解论文
溯源树
样例
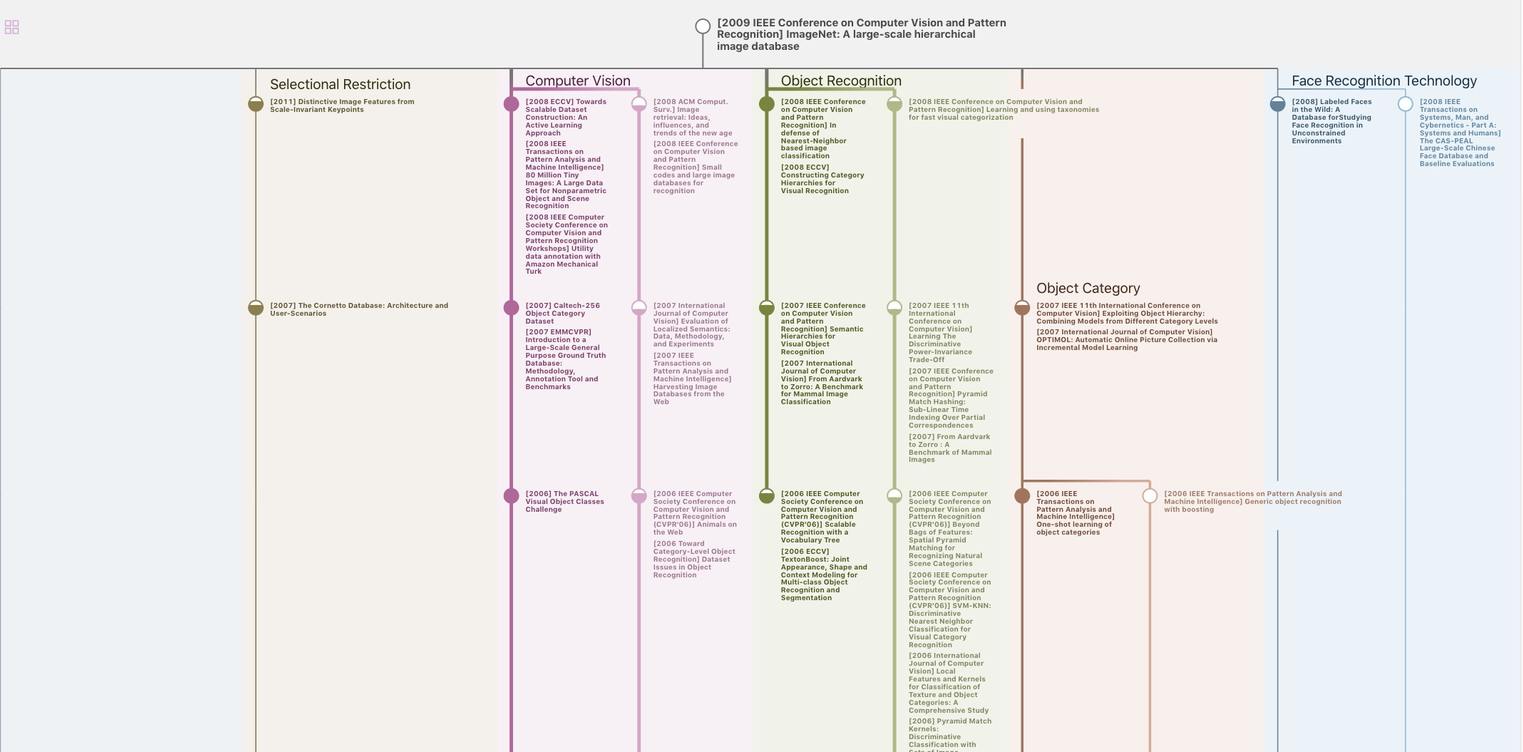
生成溯源树,研究论文发展脉络
Chat Paper
正在生成论文摘要