Stateful Neural Networks for Intermittent Systems
IEEE Transactions on Computer-Aided Design of Integrated Circuits and Systems(2022)
摘要
Deep neural network (DNN) inference on intermittently powered battery-less devices has the potential to unlock new possibilities for sustainable and intelligent edge applications. Existing intermittent inference approaches preserve progress information separate from the computed output features during inference. However, we observe that even in highly specialized approaches, the additional overhead incurred for inference progress preservation still accounts for a significant portion of the inference latency. This work proposes the concept of stateful neural networks, which enables a DNN to indicate the inference progress itself. Our runtime middleware embeds state information into the DNN such that the computed and preserved output features intrinsically contain progress indicators, avoiding the need to preserve them separately. The specific position and representation of the embedded states jointly ensure both output features and states are not corrupted while maintaining model accuracy, and the embedded states allow the latest output feature to be determined, enabling correct inference recovery upon power resumption. Evaluations were conducted on different Texas Instruments devices under varied intermittent power strengths and network models. Compared to the state-of-the-art, our approach can speed up intermittent inference by 1.3 to 5 times, achieving higher performance when executing modern convolutional networks with weaker power.
更多查看译文
关键词
Battery-less devices,deep neural networks (DNNs),energy harvesting,intermittent systems,stateful neural networks
AI 理解论文
溯源树
样例
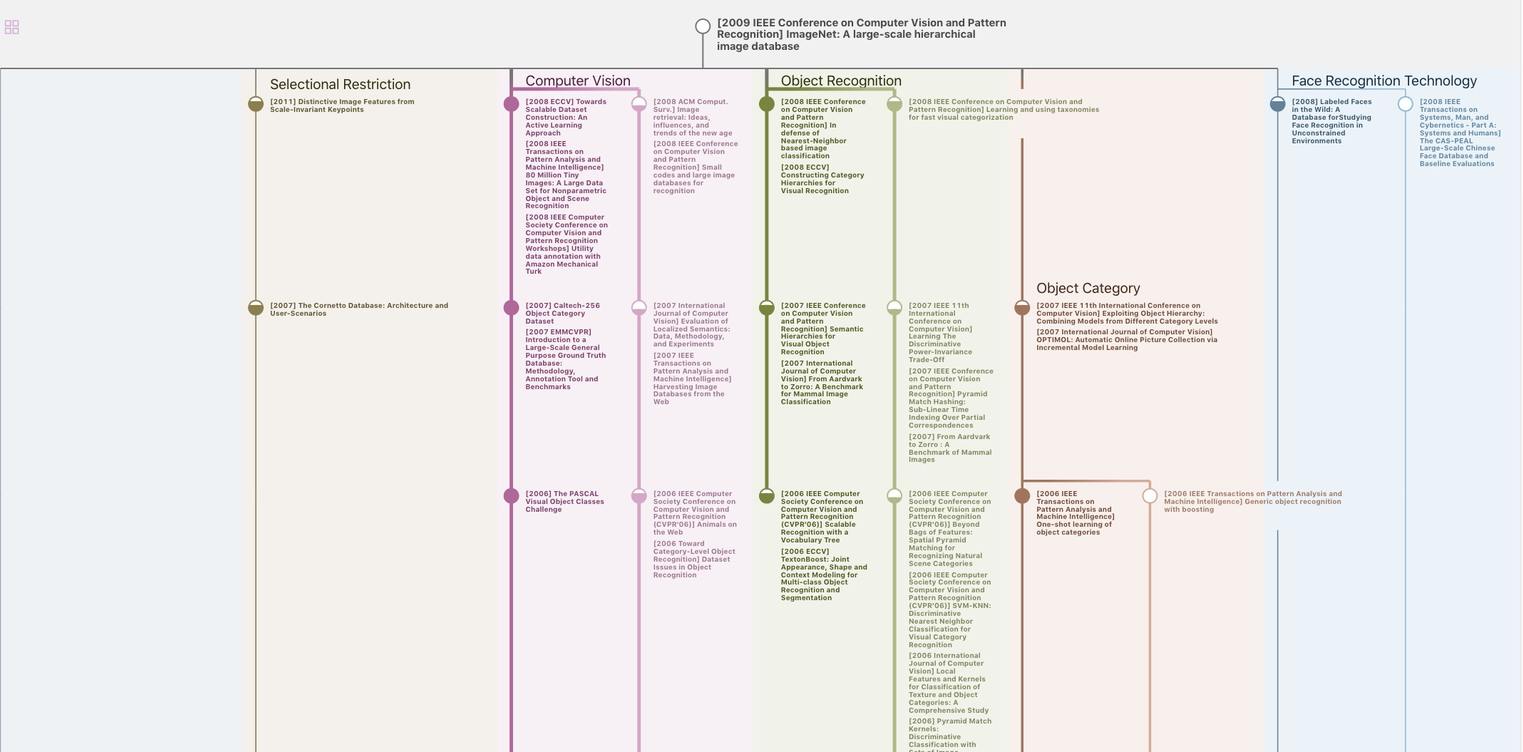
生成溯源树,研究论文发展脉络
Chat Paper
正在生成论文摘要