Unsupervised Adaptation of Spiking Networks in a Gradual Changing Environment
2022 IEEE High Performance Extreme Computing Conference (HPEC)(2022)
摘要
Spiking neural networks(SNNs) have drawn broad research interests in recent years due to their high energy efficiency and biologically-plausibility. They have proven to be competitive in many machine learning tasks. Similar to all Artificial Neural Network(ANNs) machine learning models, the SNNs rely on the assumption that the training and testing data are drawn from the same distribution. As the environment changes gradually, the input distribution will shift over time, and the performance of SNNs turns out to be brittle. To this end, we propose a unified framework that can adapt non-stationary streaming data by exploiting unlabeled intermediate domain, and fits with the in-hardware SNN learning algorithm Error-modulated STDP. Specifically, we propose a unique self-training framework to generate pseudo labels to retrain the model for intermediate and target domains. In addition, we develop an online-normalization method with an auxiliary neuron to normalize the output of the hidden layers. By combining the normalization with self-training, our approach gains average classification improvements over 10% on MNIST, NMINST, and two other datasets.
更多查看译文
关键词
Spiking Neural Networks,in-hardware learning,domain adaptation
AI 理解论文
溯源树
样例
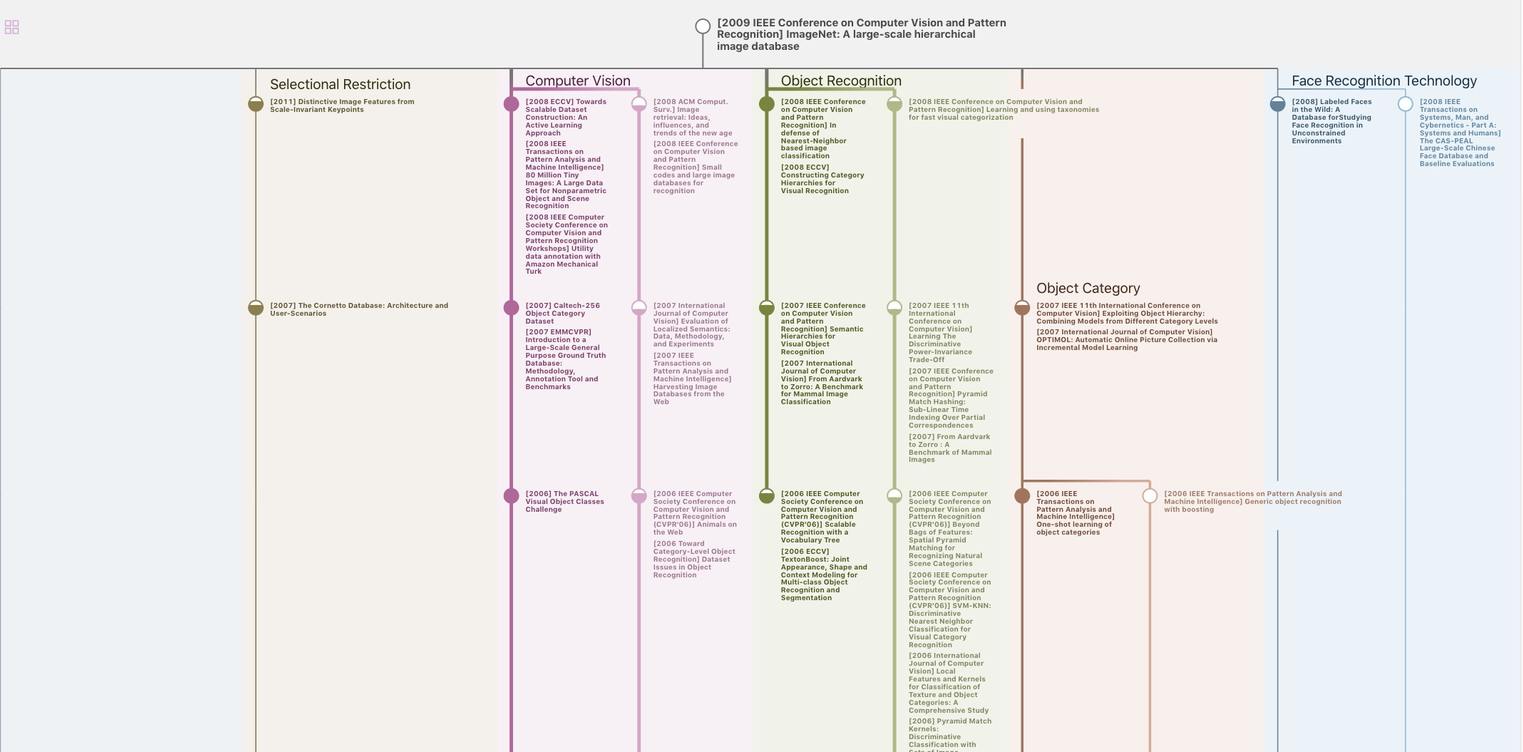
生成溯源树,研究论文发展脉络
Chat Paper
正在生成论文摘要