Increasing Patient Specificity of the Recurrent Neural Network Based Insulin Sensitivity Prediction by Transfer Learning
2022 IEEE 26th International Conference on Intelligent Engineering Systems (INES)(2022)
摘要
Insulin therapy is a frequently applied treatment in intensive care to normalize the patient's blood glucose level increased by stress-induced hyperglycaemia. This therapy is generally referred to as Tight Glycaemic Control (TGC). The STAR (Stochastic-TARgeted) protocol is a TGC which uses the patient's insulin sensitivity (SI) as a key parameter to describe the patient's actual state. Prediction of the future patient's state, i.e. prediction of the patient's future SI value, is a crucial step of the protocol currently implemented by using the so-called Intensive Care INsulin Glucose (ICING) model of the human glucose-insulin system and an associated stochastic model. In our previous studies, we have shown that the Recurrent Neural Network (RNN) models are efficient alternative methods of SI prediction. In this paper, we suggest applying the so-called transfer learning technique to further enhance the accuracy of the SI prediction by using the SI history of the current patient. The paper presents the proposed methodology for applying transfer learning in SI prediction and the evaluation of the method's accuracy by comparing the outcomes with the currently applied solution. Insilico validation using real patients' data is involved in this validation.
更多查看译文
关键词
machine learning,artificial intelligence,mixture density network,deep neural network,insulin sensitivity,tight glycaemic control,intensive care,STAR protocol,validation,in-silico validation
AI 理解论文
溯源树
样例
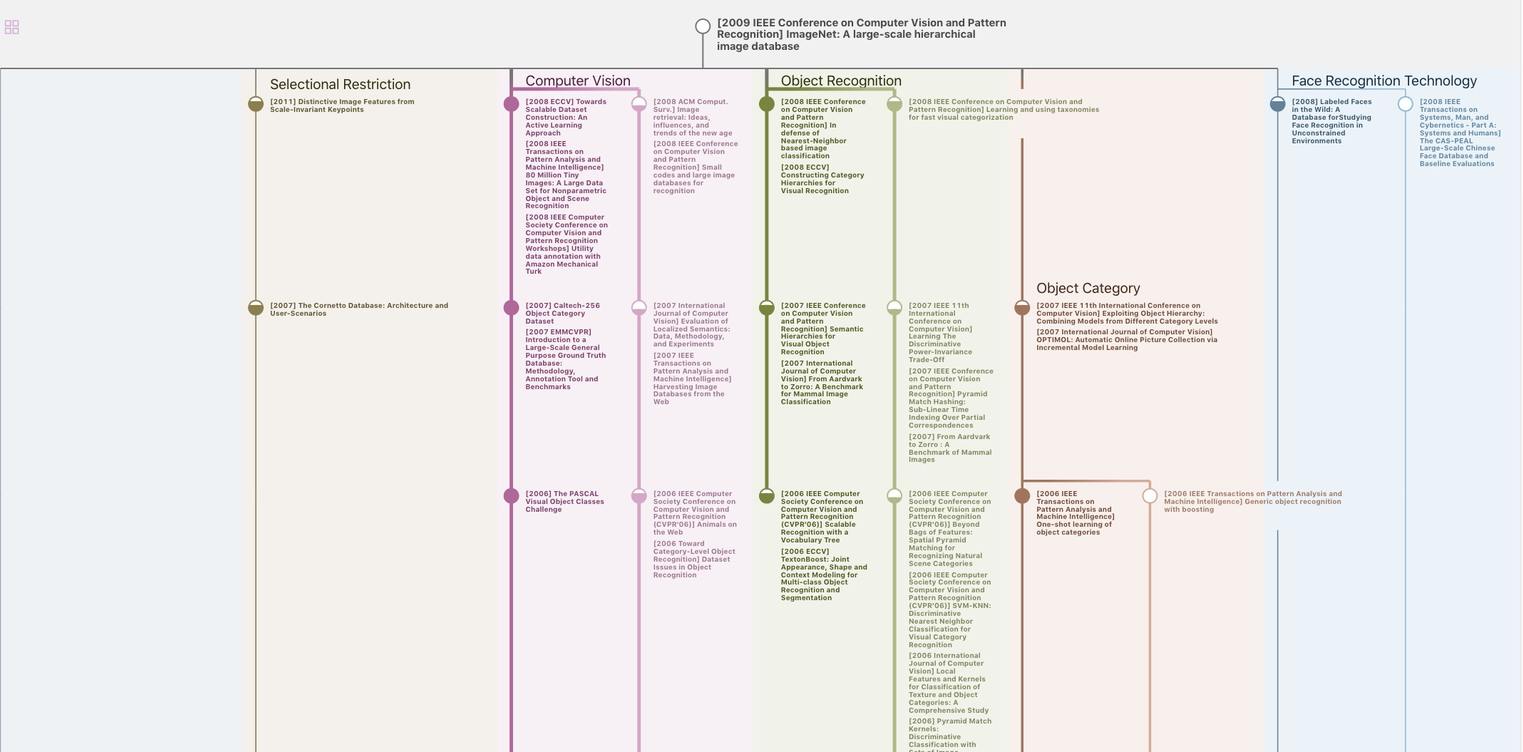
生成溯源树,研究论文发展脉络
Chat Paper
正在生成论文摘要