Online Detection and Classification of State Transitions of Multivariate Shock and Vibration Data
2022 IEEE High Performance Extreme Computing Conference (HPEC)(2022)
摘要
The US Department of Energy's (DOE) Los Alamos National Laboratory (LANL) is interested in automatic anomaly detection and classification applied to highly instrumented flight shock and vibration data for the purpose of providing insight into operational safety. For example, the safe and secure transport of materials and devices during a variety of conditions is particularly of interest. In this work, we apply well-known Machine Learning (ML) techniques to a publicly available motor vibration data set that serves as a proxy to the actual LANL data. We successfully train a random forest to classify anomalous motor states using the signal data set, and use this model to simulate real-time anomaly detection and event classification on multi-variate time series data [1], [2]. Furthermore, we perform an extensive suite of computational studies on a large cluster computer to determine optimal parametric settings for our framework and evaluate the cost-benefit of these parameters.
更多查看译文
关键词
machine learning,signal processing,change point detection
AI 理解论文
溯源树
样例
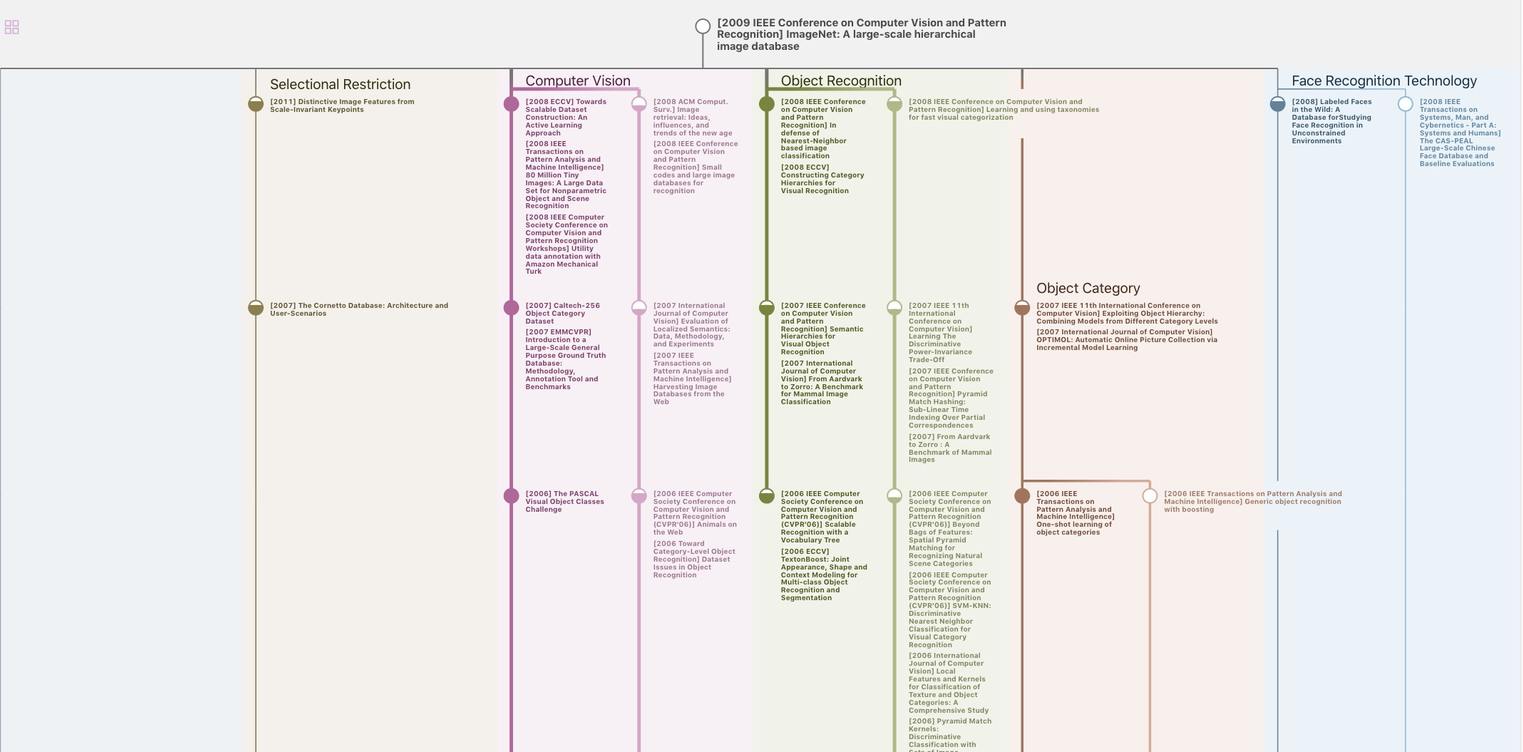
生成溯源树,研究论文发展脉络
Chat Paper
正在生成论文摘要