Dimensionality reduction of local structure in glassy binary mixtures.
The Journal of chemical physics(2022)
摘要
We consider unsupervised learning methods for characterizing the disordered microscopic structure of supercooled liquids and glasses. Specifically, we perform dimensionality reduction of smooth structural descriptors that describe radial and bond-orientational correlations and assess the ability of the method to grasp the essential structural features of glassy binary mixtures. In several cases, a few collective variables account for the bulk of the structural fluctuations within the first coordination shell and also display a clear connection with the fluctuations of particle mobility. Fine-grained descriptors that characterize the radial dependence of bond-orientational order better capture the structural fluctuations relevant for particle mobility but are also more difficult to parameterize and to interpret. We also find that principal component analysis of bond-orientational order parameters provides identical results to neural network autoencoders while having the advantage of being easily interpretable. Overall, our results indicate that glassy binary mixtures have a broad spectrum of structural features. In the temperature range we investigate, some mixtures display well-defined locally favored structures, which are reflected in bimodal distributions of the structural variables identified by dimensionality reduction.
更多查看译文
关键词
binary mixtures,dimensionality reduction,glassy
AI 理解论文
溯源树
样例
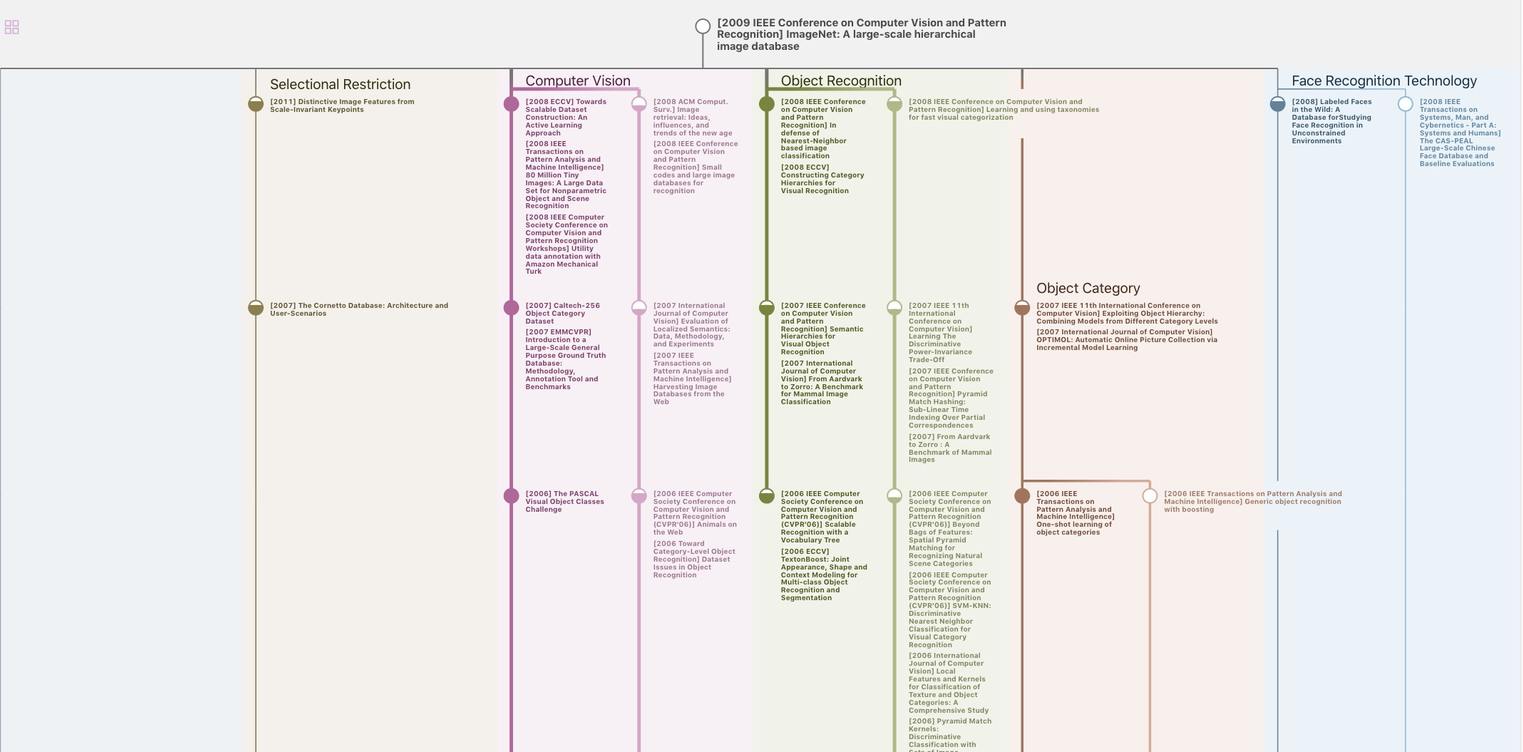
生成溯源树,研究论文发展脉络
Chat Paper
正在生成论文摘要