FedMint: Intelligent Bilateral Client Selection in Federated Learning With Newcomer IoT Devices
IEEE INTERNET OF THINGS JOURNAL(2023)
摘要
Federated learning (FL) is a novel distributed privacy-preserving learning paradigm, which enables the collaboration among several participants (e.g., Internet of Things (IoT) devices) for the training of machine learning models. However, selecting the participants that would contribute to this collaborative training is highly challenging. Adopting a random selection strategy would entail substantial problems due to the heterogeneity in terms of data quality, and computational and communication resources across the participants. Although several approaches have been proposed in the literature to overcome the problem of random selection, most of these approaches follow a unilateral selection strategy. In fact, they base their selection strategy on only the federated server's side, while overlooking the interests of the client devices in the process. To overcome this problem, we present in this article FedMint, an intelligent client selection approach for FL on IoT devices using game theory and bootstrapping mechanism. Our solution involves the design of: 1) preference functions for the client IoT devices and federated servers to allow them to rank each other according to several factors, such as accuracy and price; 2) intelligent matching algorithms that take into account the preferences of both parties in their design; and 3) bootstrapping technique that capitalizes on the collaboration of multiple federated servers in order to assign initial accuracy value for the newly connected IoT devices. We compare our approach against the VanillaFL selection process as well as other state-of-the-art approach and showcase the superiority of our proposal.
更多查看译文
关键词
Bootstrapping,client selection,federated learning (FL),game theory,incentive mechanism,Internet of Things (IoT),newcomer client,pricing
AI 理解论文
溯源树
样例
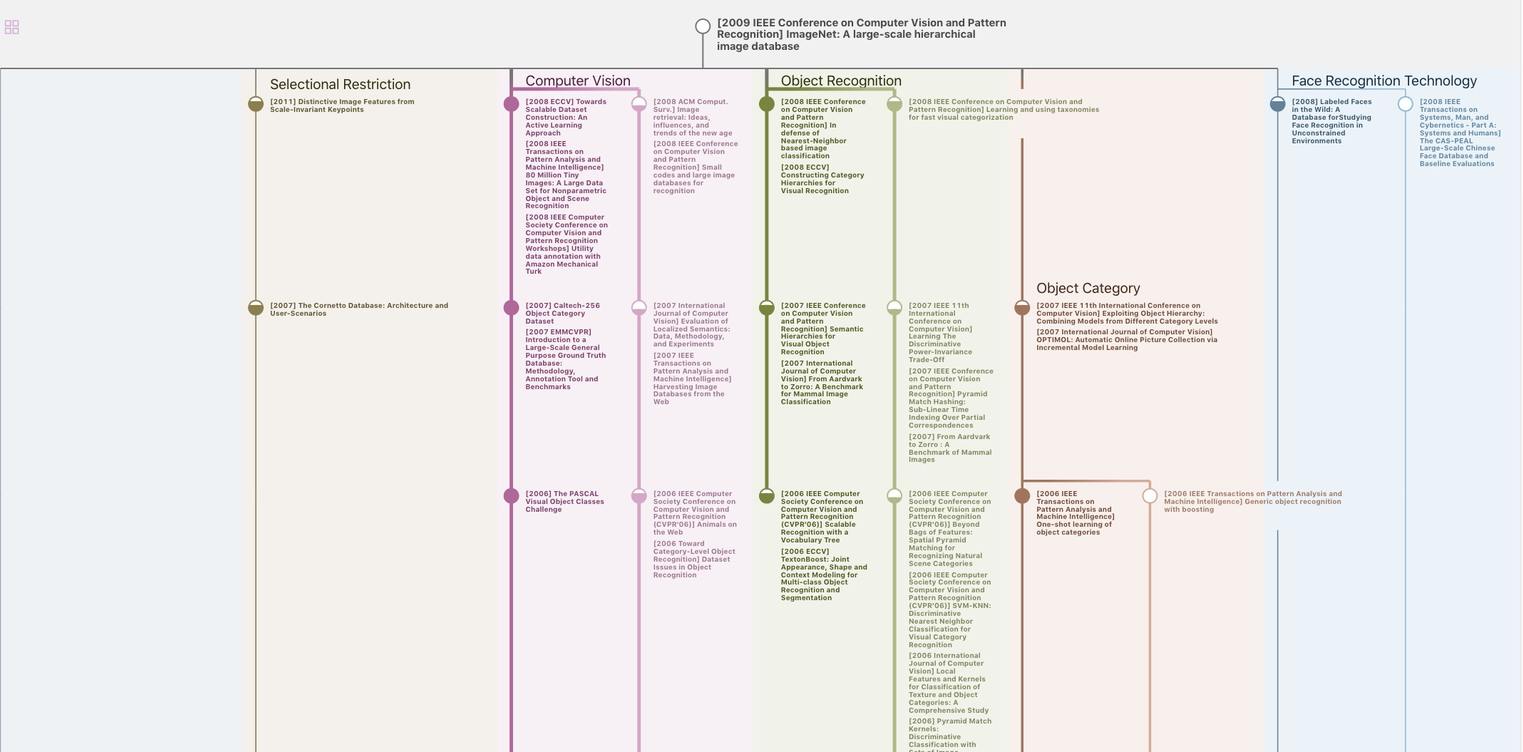
生成溯源树,研究论文发展脉络
Chat Paper
正在生成论文摘要