A Convergence Theory for Federated Average: Beyond Smoothness
arxiv(2022)
摘要
Federated learning enables a large amount of edge computing devices to learn a model without data sharing jointly. As a leading algorithm in this setting, Federated Average (FedAvg), which runs Stochastic Gradient Descent (SGD) in parallel on local devices and averages the sequences only once in a while, have been widely used due to their simplicity and low communication cost. However, despite recent research efforts, it lacks theoretical analysis under assumptions beyond smoothness. In this paper, we analyze the convergence of FedAvg. Different from the existing work, we relax the assumption of strong smoothness. More specifically, we assume the semi-smoothness and semi-Lipschitz properties for the loss function, which have an additional first-order term in assumption definitions. In addition, we also assume bound on the gradient, which is weaker than the commonly used bounded gradient assumption in the convergence analysis scheme. As a solution, this paper provides a theoretical convergence study on Federated Learning.
更多查看译文
关键词
federated average,convergence theory
AI 理解论文
溯源树
样例
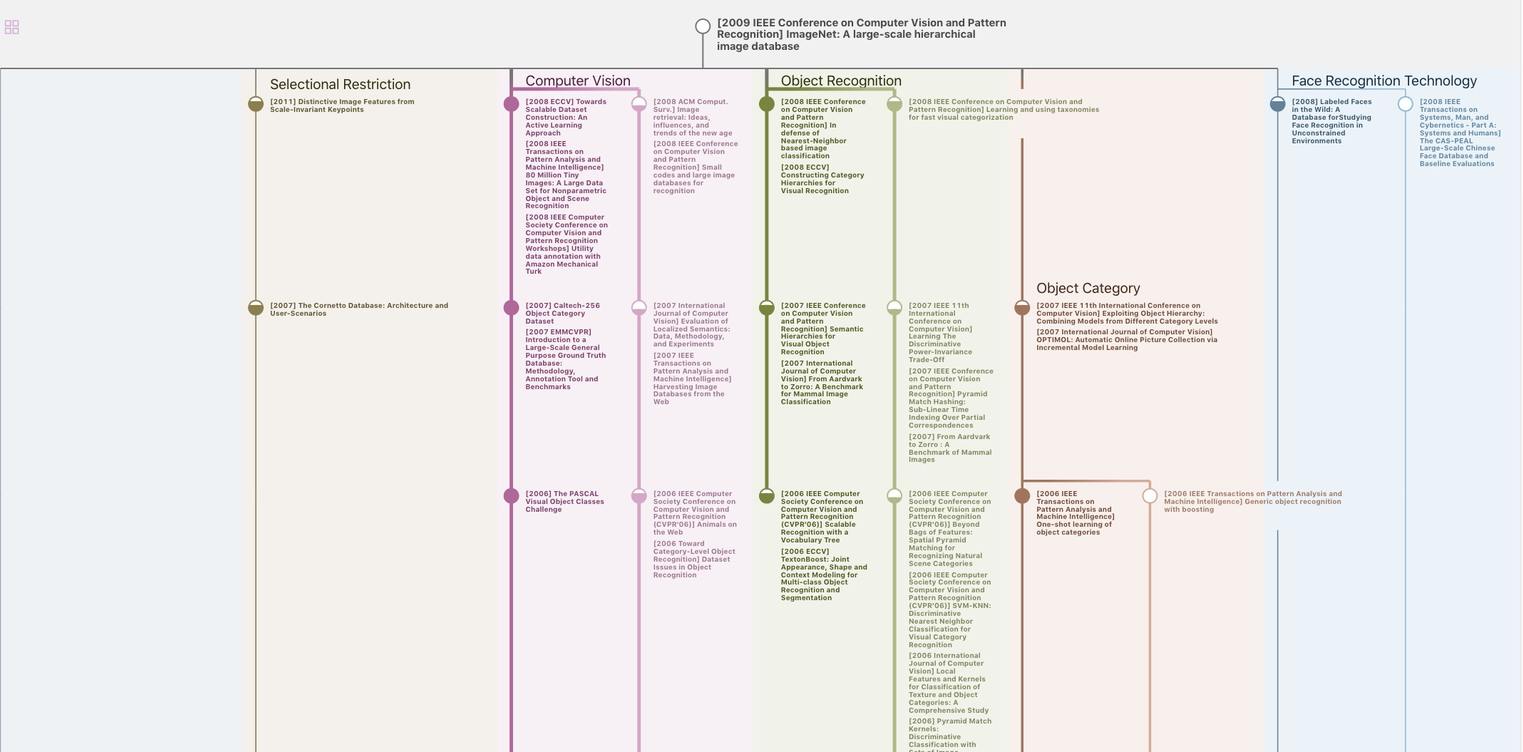
生成溯源树,研究论文发展脉络
Chat Paper
正在生成论文摘要