Variable Attention Masking for Configurable Transformer Transducer Speech Recognition
arxiv(2023)
摘要
This work studies the use of attention masking in transformer transducer based speech recognition for building a single configurable model for different deployment scenarios. We present a comprehensive set of experiments comparing fixed masking, where the same attention mask is applied at every frame, with chunked masking, where the attention mask for each frame is determined by chunk boundaries, in terms of recognition accuracy and latency. We then explore the use of variable masking, where the attention masks are sampled from a target distribution at training time, to build models that can work in different configurations. Finally, we investigate how a single configurable model can be used to perform both first pass streaming recognition and second pass acoustic rescoring. Experiments show that chunked masking achieves a better accuracy vs latency trade-off compared to fixed masking, both with and without FastEmit. We also show that variable masking improves the accuracy by up to 8% relative in the acoustic re-scoring scenario.
更多查看译文
关键词
Neural Transducer,Transformer Transducer,Conformer,Attention Masking,Acoustic Rescoring
AI 理解论文
溯源树
样例
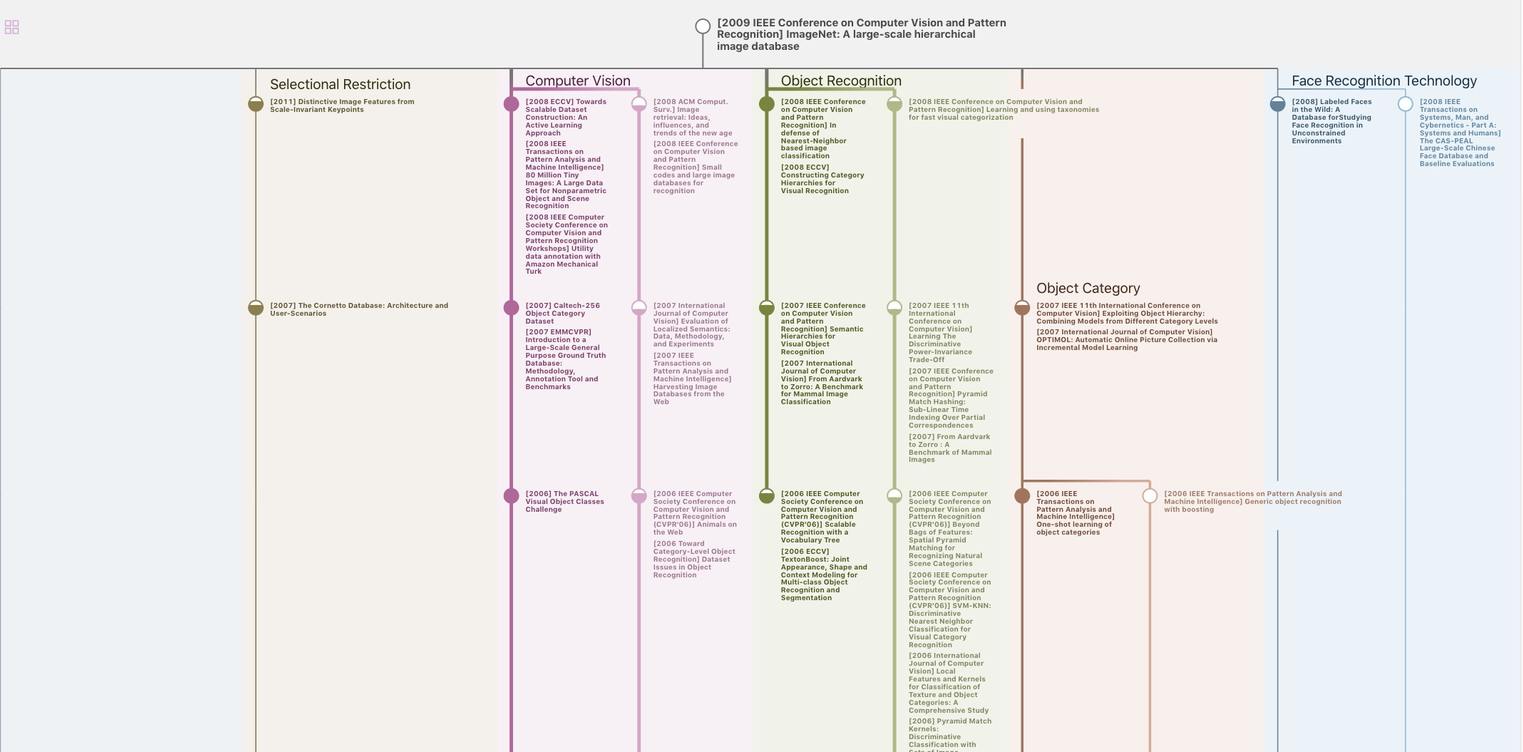
生成溯源树,研究论文发展脉络
Chat Paper
正在生成论文摘要