Non-Intrusive Load Disaggregation of Industrial Cooling Demand with LSTM Neural Network
2022 IEEE International Conference on Environment and Electrical Engineering and 2022 IEEE Industrial and Commercial Power Systems Europe (EEEIC / I&CPS Europe)(2022)
摘要
As the telecommunication industry becomes more and more energy intensive, energy efficiency actions are crucial and urgent measures to achieve energy savings. The main contribution to the energy demand of buildings devoted to the operation of the telecommunication network is cooling. The main issue in order to assess the impact of cooling equipment energy consumption to support energy managers with awareness over the buildings energy outlook is the lack of monitoring devices providing disaggregated load measurements. This work proposes a Non-Intrusive Load Disaggregation (NILD) tool that exploits a literature-based decomposition with an innovative LSTM Neural Network-based decomposition algorithm to assess cooling demand. The proposed methodology has been employed to analyze a real-case dataset containing aggregated load profiles from around sixty telecommunication buildings, resulting in accurate, compliant, and meaningful outcomes.
更多查看译文
关键词
Non-Intrusive Load Disaggregation,Long Short-Term Memory,Cooling Load
AI 理解论文
溯源树
样例
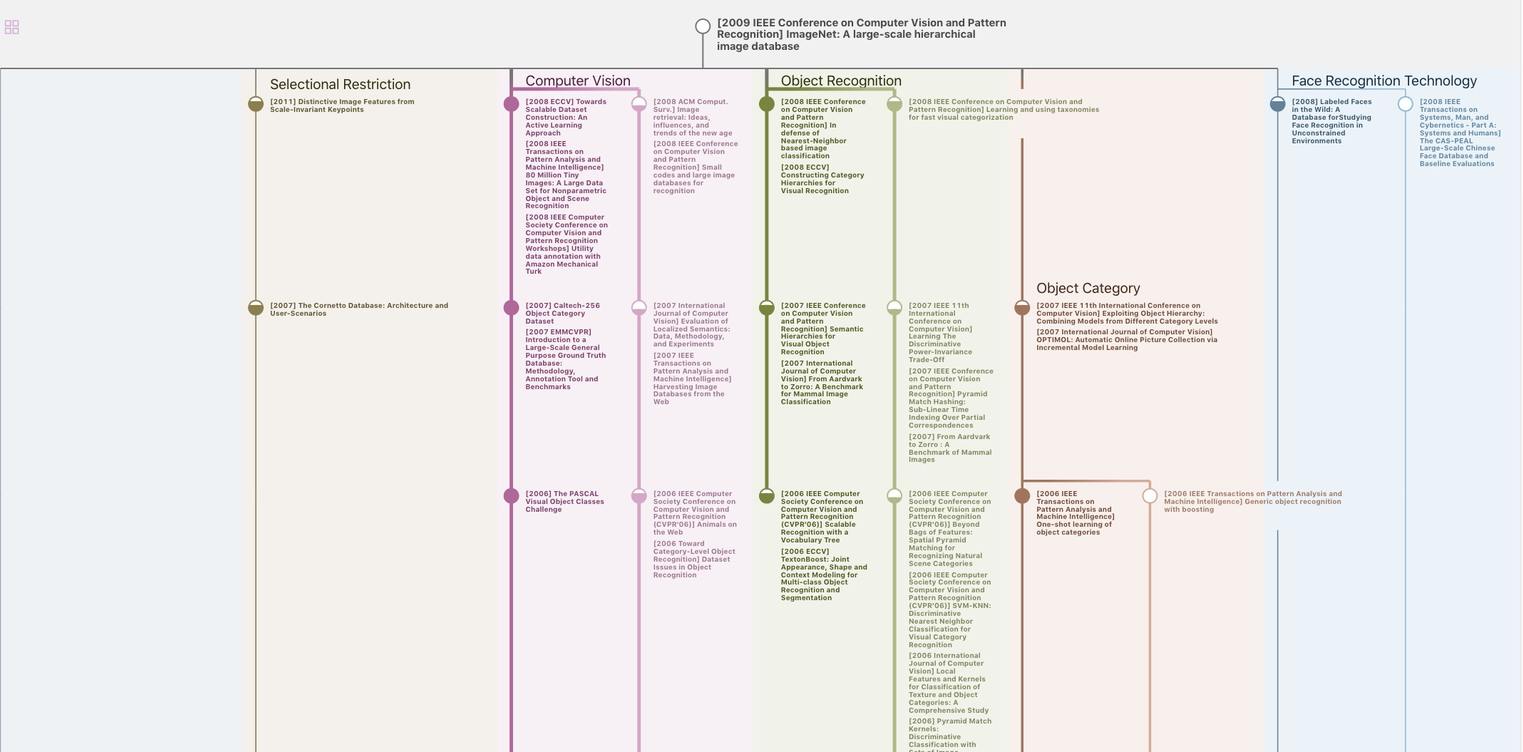
生成溯源树,研究论文发展脉络
Chat Paper
正在生成论文摘要