Evolving Counterfactual Explanations with Particle Swarm Optimization and Differential Evolution
2022 IEEE Congress on Evolutionary Computation (CEC)(2022)
摘要
Counterfactual explanations are a popular eXplainable AI technique, used to provide contrastive answers to “what-if” questions. These explanations are consistent with the way that an everyday person will explain an event, and have been shown to satisfy the ‘right to explanation’ of the European data regulations. Despite this, current work to generate counterfactual explanations either makes assumptions about the model being explained or utlises algorithms that perform suboptimally on continuous data. This work presents two novel algorithms to generate counterfactual explanations using Particle Swarm Optimization (PSO) and Differential Evolution (DE). These are shown to provide effective post-hoc explanations that make no assumptions about the underlying model or data structure. In particular, PSO is shown to generate counterfactual explanations that utilise significantly fewer features to generate sparser explanations when compared to previous related work.
更多查看译文
关键词
explainable AI,counterfactual explanation,particle swarm optimization,differential evolution
AI 理解论文
溯源树
样例
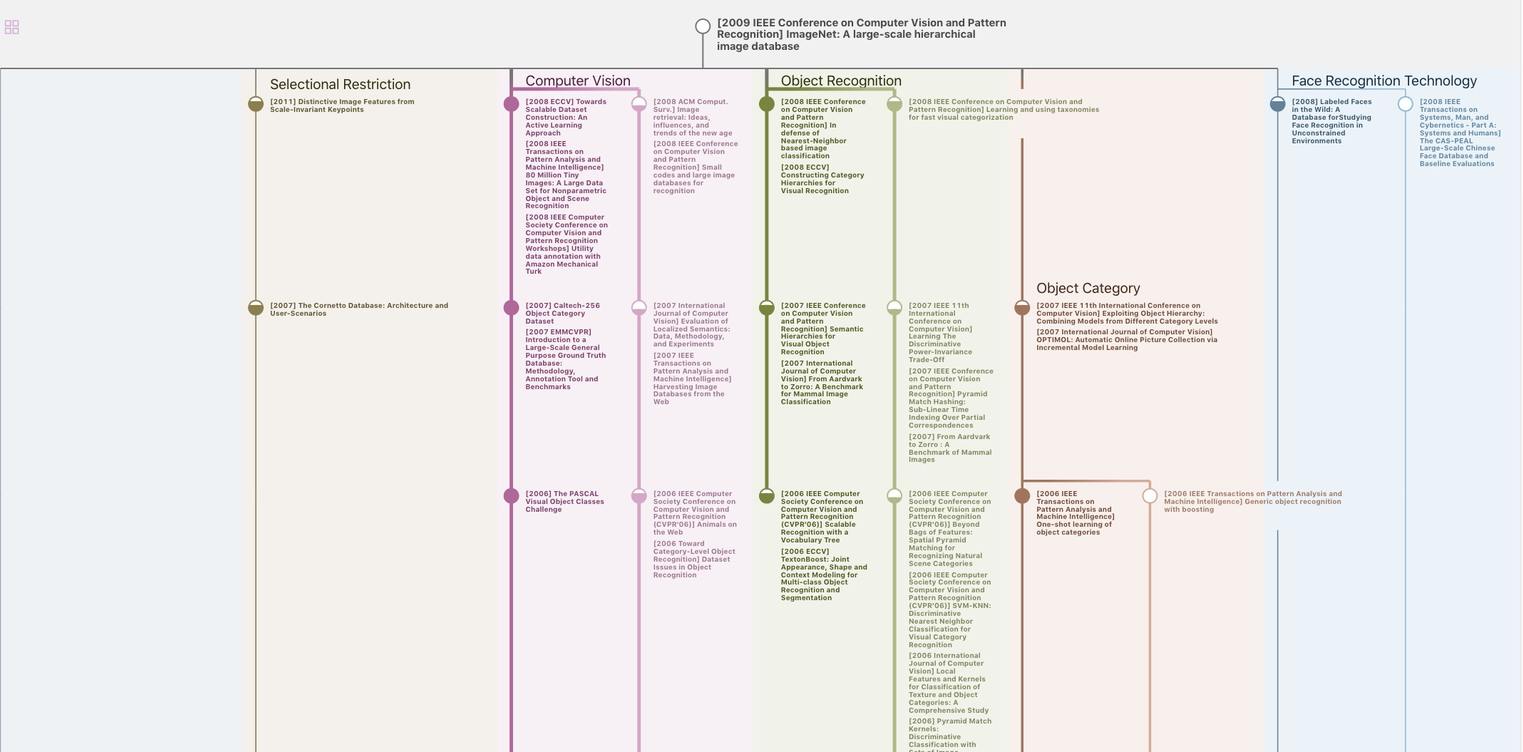
生成溯源树,研究论文发展脉络
Chat Paper
正在生成论文摘要