Combining Soft-Actor Critic with Cross-Entropy Method for Policy Search in Continuous Control
2022 IEEE Congress on Evolutionary Computation (CEC)(2022)
摘要
In this paper, we propose CEM-SAC - a hybridization between the cross-entropy method (CEM), i.e., an estimation-of-distribution algorithm, and the soft-actor critic (SAC), i.e., a state-of-the-art policy gradient algorithm. Our work extends the evolutionary reinforcement learning (ERL) line of research on integrating the robustness of population-based stochastic black-box optimization, that typically assumes little to no problem-specific knowledge, into the training process of policy gradient algorithms, that exploits the sequential decision making nature for efficient gradient estimation. Our hybrid approach, CEM-SAC, exhibits both the stability of CEM and the efficiency of SAC in training policy neural networks of reinforcement learning agents for solving control problems. Experimental result comparisons with the three baselines CEM, SAC, and CEM-TD3, a recently-introduced ERL method that combines CEM and the twin-delayed deep deterministic policy gradient (TD3) algorithm, on a wide range of control tasks in the MuJoCo benchmarks confirm the enhanced performance of our proposed CEM-SAC. The source code is available at https://github.com/ELO-Lab/CEM-SAC.
更多查看译文
关键词
Reinforcement learning,Evolutionary computation,Cross-entropy method,Soft actor-critic,Policy search
AI 理解论文
溯源树
样例
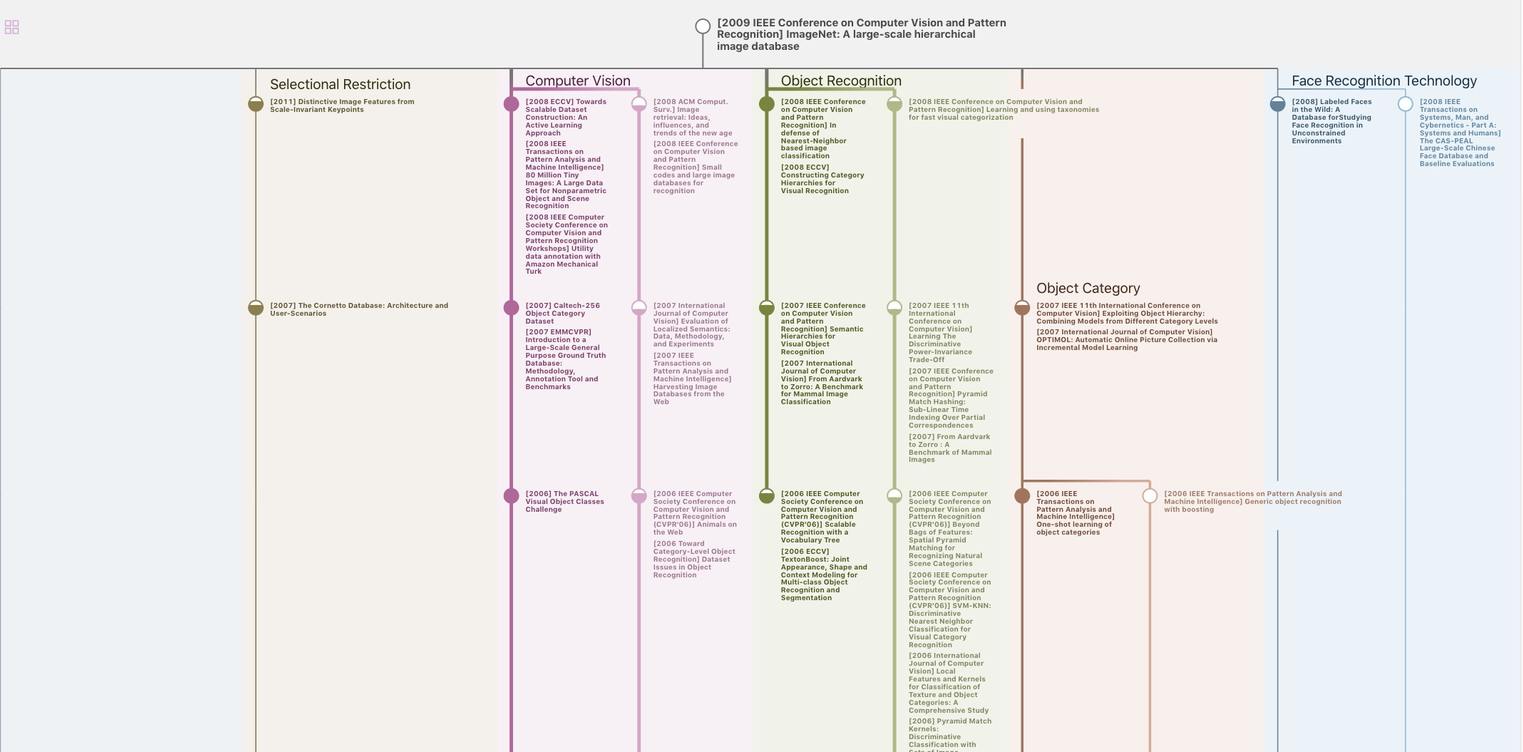
生成溯源树,研究论文发展脉络
Chat Paper
正在生成论文摘要