MSCN-NET: Multi-stage cascade neural network based on attention mechanism for Cerenkov luminescence tomography
JOURNAL OF APPLIED PHYSICS(2022)
摘要
Cerenkov luminescence tomography (CLT) is a highly sensitive and promising technique for three-dimensional non-invasive detection of radiopharmaceuticals in living organisms. However, the severe photon scattering effect causes ill-posedness of the inverse problem, and the results of CLT reconstruction are still unsatisfactory. In this work, a multi-stage cascade neural network is proposed to improve the performance of CLT reconstruction, which is based on the attention mechanism and introduces a special constraint. The network cascades an inverse sub-network (ISN) and a forward sub-network (FSN), where the ISN extrapolates the distribution of internal Cerenkov sources from the surface photon intensity, and the FSN is used to derive the surface photon intensity from the reconstructed Cerenkov source, similar to the transmission process of photons in living organisms. In addition, the FSN further optimizes the reconstruction results of the ISN. To evaluate the performance of our proposed method, numerical simulation experiments and in vivo experiments were carried out. The results show that compared with the existing methods, this method can achieve superior performance in terms of location accuracy and shape recovery capability.
更多查看译文
关键词
attention mechanism,neural network,luminescence,tomography,mscn-net,multi-stage
AI 理解论文
溯源树
样例
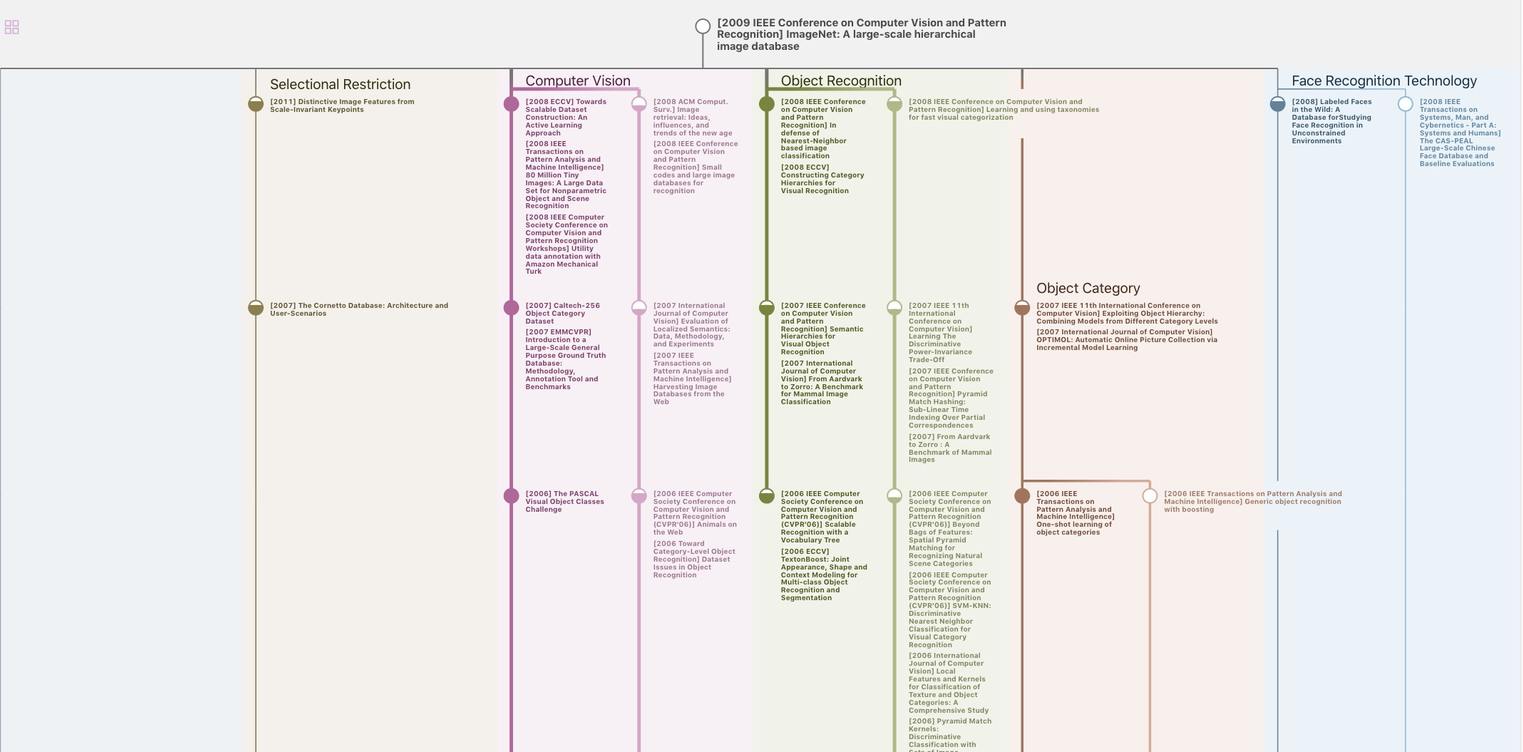
生成溯源树,研究论文发展脉络
Chat Paper
正在生成论文摘要