Robust model selection for positive and unlabeled learning with constraints
SCIENCE CHINA-INFORMATION SCIENCES(2022)
摘要
Positive and unlabeled (PU) learning is the problem in which training data contains only PU samples. Although PU learning is widely used in real-world applications, its model selection remains challenging. Specifically, traditional model selection methods are often highly sensitive to the class prior as well as the data size, resulting in human overhead in hyperparameter optimization. In this paper, we present a method called ODE (robust model selection) for robust model selection in PU learning. Two novel model evaluators based on the integral probability metric and area under the curve, which are free of the class prior, are introduced, and a variance reduction method is further employed to improve the quality of model selection. In addition, we perform model selection under user-defined constraints and propose a fast halving-style searching algorithm to efficiently identify the most promising model configuration. Extensive empirical studies demonstrate that our proposed method performs more robustly and is more computationally efficient than many state-of-the-art methods.
更多查看译文
关键词
positive and unlabeled learning,model selection,automated machine learning
AI 理解论文
溯源树
样例
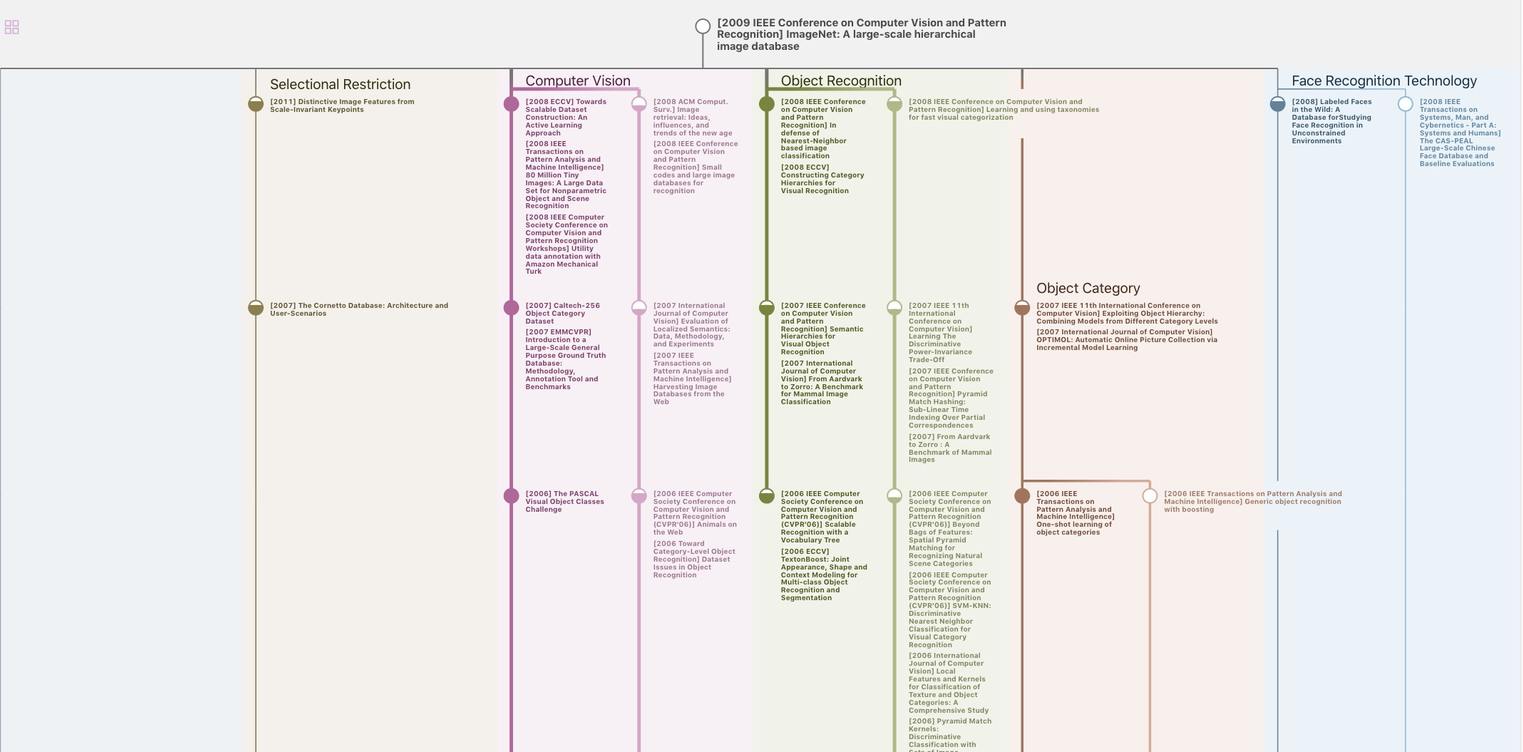
生成溯源树,研究论文发展脉络
Chat Paper
正在生成论文摘要