Semantic SuperPoint: A Deep Semantic Descriptor
2022 Latin American Robotics Symposium (LARS), 2022 Brazilian Symposium on Robotics (SBR), and 2022 Workshop on Robotics in Education (WRE)(2022)
摘要
Several SLAM methods benefit from the use of semantic information. Most integrate photometric methods with high-level semantics such as object detection and semantic segmentation. We propose that adding a semantic segmentation decoder in a shared encoder architecture would help the descriptor decoder learn semantic information, improving the feature extractor. This would be a more robust approach than only using high-level semantic information since it would be intrinsically learned in the descriptor and would not depend on the final quality of the semantic prediction. To add this information, we take advantage of multi-task learning methods to improve accuracy and balance the performance of each task. The proposed models are evaluated according to detection and matching metrics on the HPatches dataset. The results show that the Semantic SuperPoint model performs better than the baseline one.
更多查看译文
关键词
Computer Vision,Visual Odometry
AI 理解论文
溯源树
样例
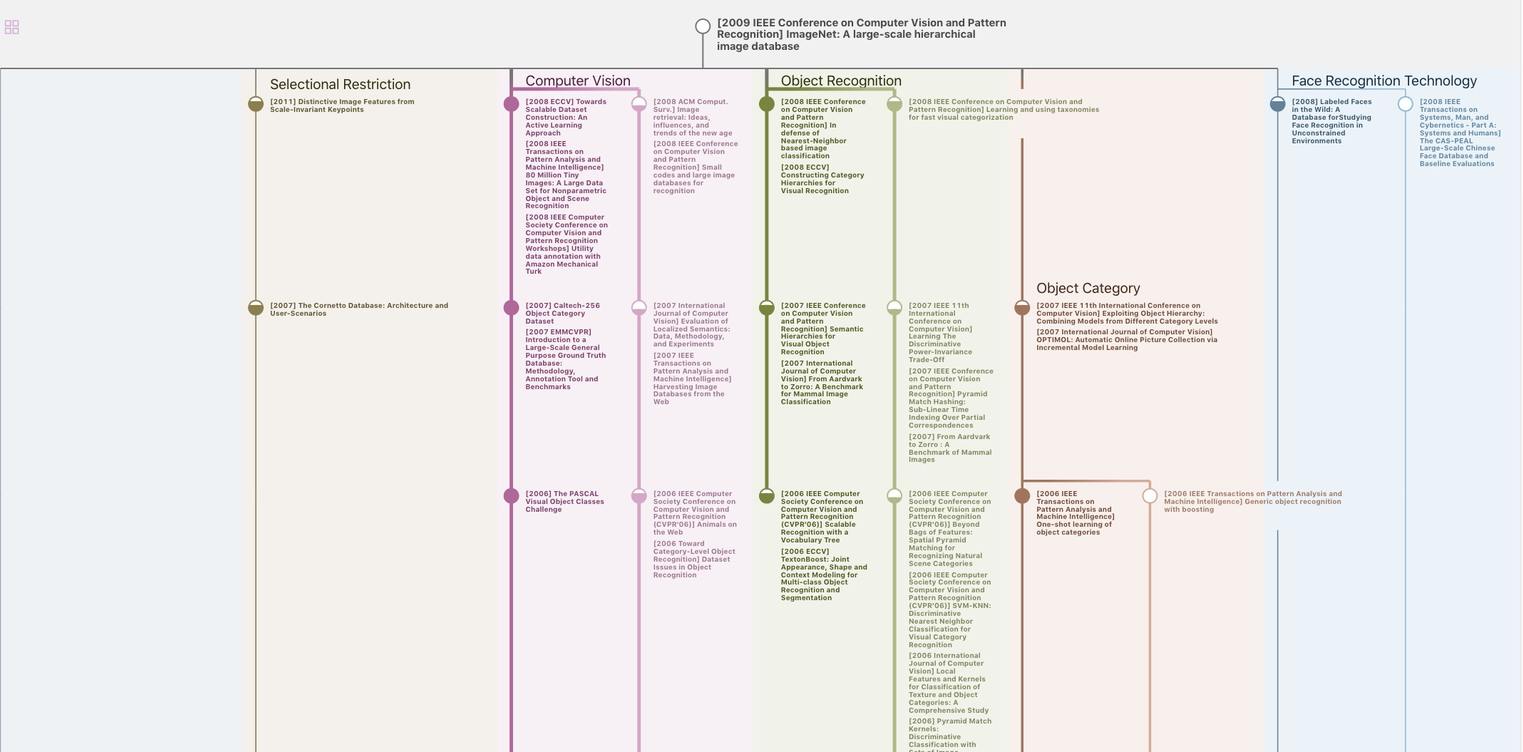
生成溯源树,研究论文发展脉络
Chat Paper
正在生成论文摘要