DSPGAN: A Gan-Based Universal Vocoder for High-Fidelity TTS by Time-Frequency Domain Supervision from DSP
arxiv(2023)
摘要
Recent development of neural vocoders based on the generative adversarial neural network (GAN) has shown obvious advantages of generating raw waveform conditioned on mel-spectrogram with fast inference speed and lightweight networks. Whereas, it is still challenging to train a universal neural vocoder that can synthesize high-fidelity speech from various scenarios with unseen speakers, languages, and speaking styles. In this paper, we propose DSP- GAN, a GAN-based universal vocoder for high-fidelity speech synthesis by applying the time-frequency domain supervision from digital signal processing (DSP). To eliminate the mismatch problem caused by the ground-truth spectrograms in the training phase and the predicted spectrograms in the inference phase, we leverage the mel-spectrogram extracted from the waveform generated by a DSP module, rather than the predicted mel-spectrogram from the Text-to-Speech (TTS) acoustic model, as the time-frequency domain supervision to the GAN-based vocoder. We also utilize sine excitation as the time-domain supervision to improve the harmonic modeling and eliminate various artifacts of the GAN-based vocoder. Experiments show that DSPGAN significantly outperforms the compared approaches and it can generate high-fidelity speech for various TTS models trained using diverse data.
1
更多查看译文
关键词
universal vocoder,generative adversarial network,digital signal processing,source-filter model
AI 理解论文
溯源树
样例
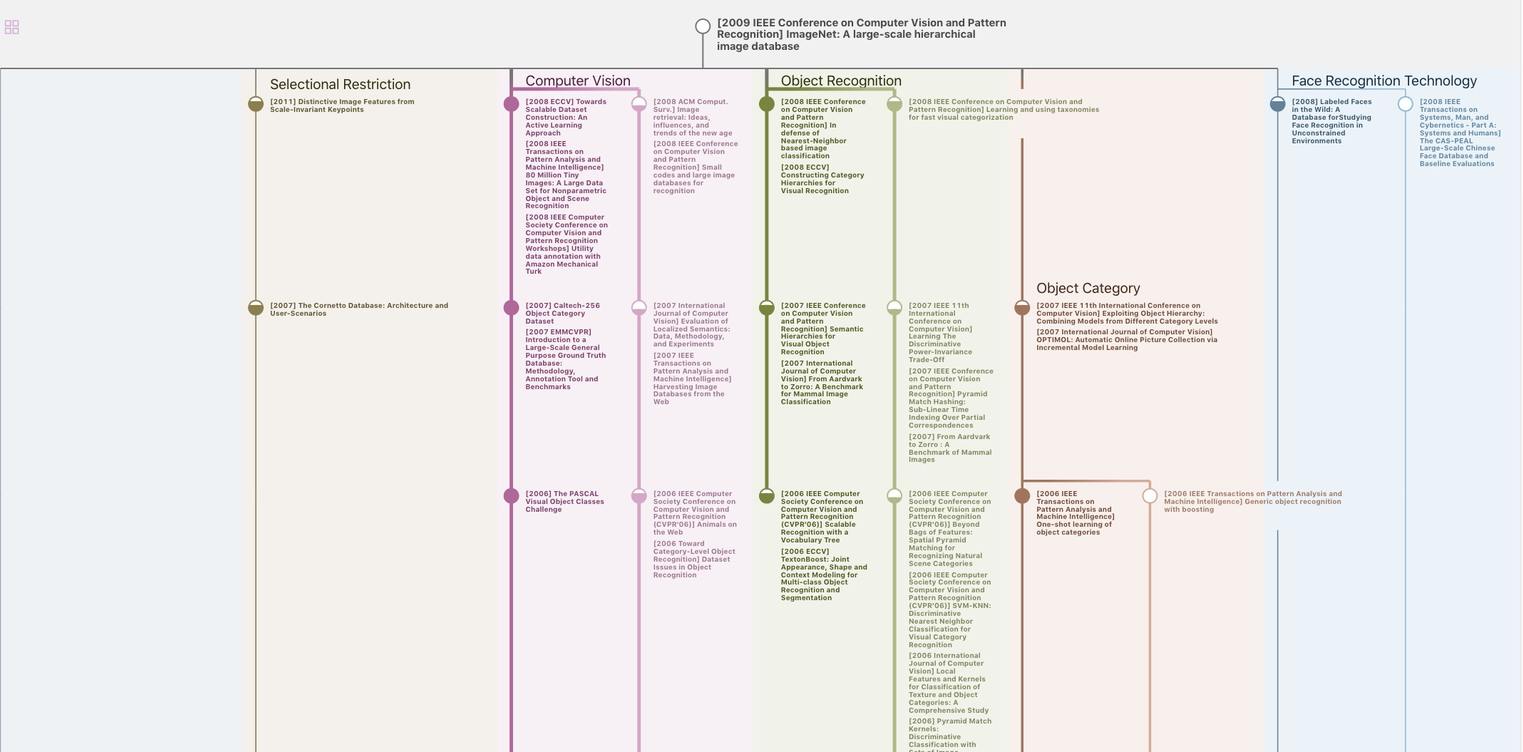
生成溯源树,研究论文发展脉络
Chat Paper
正在生成论文摘要