Tree representations of brain structural connectivity via persistent homology
arxiv(2023)
摘要
The brain structural connectome is generated by a collection of white matter fiber bundles constructed from diffusion weighted MRI (dMRI), acting as highways for neural activity. There has been abundant interest in studying how the structural connectome varies across individuals in relation to their traits, ranging from age and gender to neuropsychiatric outcomes. After applying tractography to dMRI to get white matter fiber bundles, a key question is how to represent the brain connectome to facilitate statistical analyses relating connectomes to traits. The current standard divides the brain into regions of interest (ROIs), and then relies on an adjacency matrix (AM) representation. Each cell in the AM is a measure of connectivity, e.g., number of fiber curves, between a pair of ROIs. Although the AM representation is intuitive, a disadvantage is the high-dimensionality due to the large number of cells in the matrix. This article proposes a simpler tree representation of the brain connectome, which is motivated by ideas in computational topology and takes topological and biological information on the cortical surface into consideration. We demonstrate that our tree representation preserves useful information and interpretability, while reducing dimensionality to improve statistical and computational efficiency. Applications to data from the Human Connectome Project (HCP) are considered and code is provided for reproducing our analyses.
更多查看译文
关键词
adjacency matrix,brain connectome,persistent homology,structural connectivity,tree
AI 理解论文
溯源树
样例
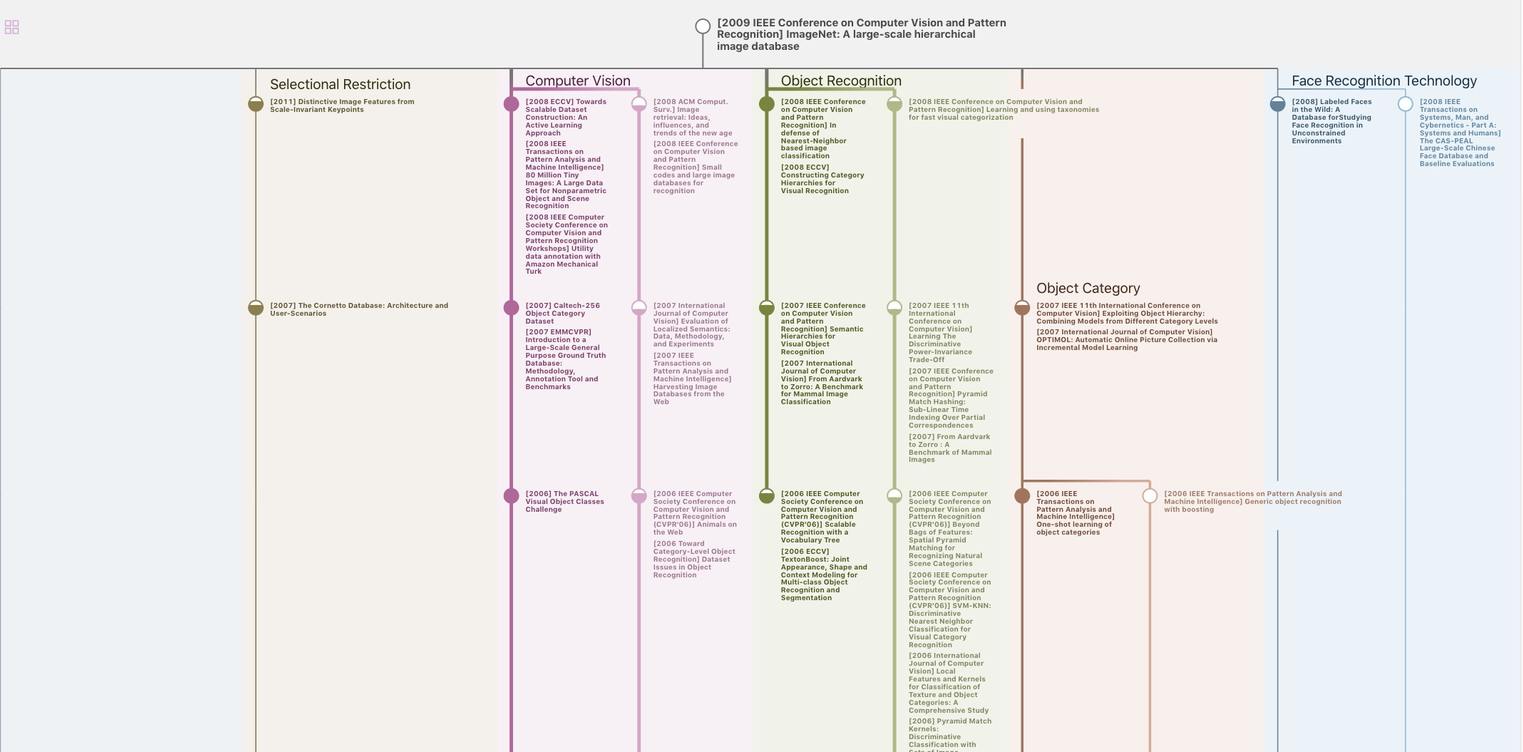
生成溯源树,研究论文发展脉络
Chat Paper
正在生成论文摘要