HFN:Heterogeneous Feature Network for Multivariate Time Series Anomaly Detection
Information Sciences(2024)
摘要
As the key step of anomaly detection for multivariate time-series (MTS) data, learning the relations among different variables has been explored by many approaches. However, most existing approaches overlook the heterogeneity among variables, that is, different types of variables (continuous numerical variables, discrete categorical variables or hybrid variables) may have different edge distributions. In this paper, we propose a novel semi-supervised anomaly detection framework based on a heterogeneous feature network (HFN) for MTS. Specifically, we first combine the embedding similarity subgraph generated by sensor embedding and the feature value similarity subgraph generated by sensor values to construct a time-series heterogeneous graph, which fully utilizes the rich heterogeneous mutual information among variables. Then, a prediction model containing nodes and channel attentions is jointly optimized to obtain better time-series representations. This approach fuses the state-of-the-art technologies of heterogeneous graph structure learning (HGSL) and representation learning. Experimental results on four sensor datasets from real-world applications demonstrate that our approach achieves more accurate anomaly detection compared to baseline methods, laying a foundation for the rapid positioning of anomalies.
更多查看译文
关键词
Heterogeneous neural network,Anomaly detection,Multi-sensor data,Multivariate time series,Deep learning
AI 理解论文
溯源树
样例
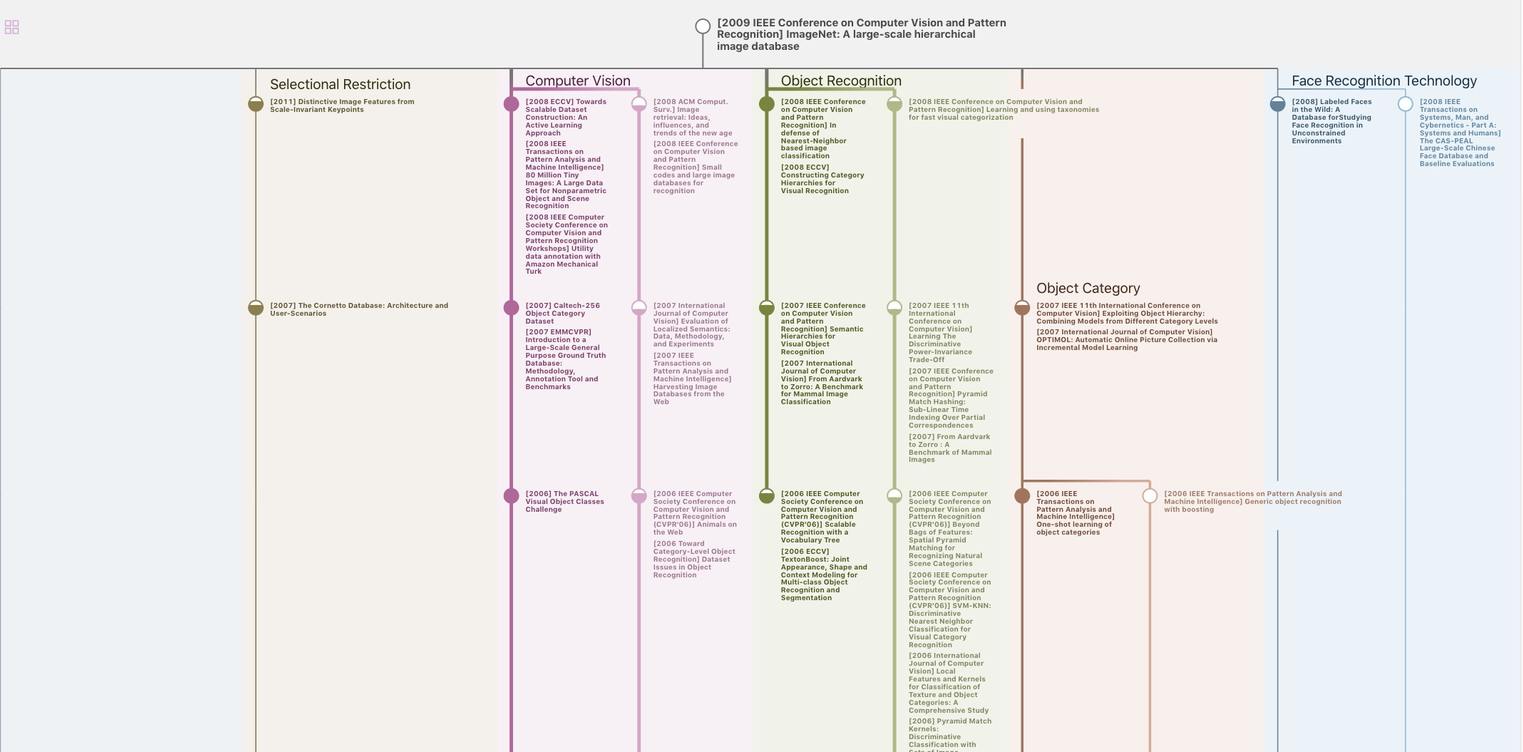
生成溯源树,研究论文发展脉络
Chat Paper
正在生成论文摘要