Generating Sequences by Learning to Self-Correct
ICLR 2023(2022)
摘要
Sequence generation applications require satisfying semantic constraints, such as ensuring that programs are correct, using certain keywords, or avoiding undesirable content. Language models, whether fine-tuned or prompted with few-shot demonstrations, frequently violate these constraints, and lack a mechanism to iteratively revise their outputs. Moreover, some powerful language models are of extreme scale or inaccessible, making it inefficient, if not infeasible, to update their parameters for task-specific adaptation. We present Self-Correction, an approach that decouples an imperfect base generator (an off-the-shelf language model or supervised sequence-to-sequence model) from a separate corrector that learns to iteratively correct imperfect generations. To train the corrector, we propose an online training procedure that can use either scalar or natural language feedback on intermediate imperfect generations. We show that Self-Correction improves upon the base generator in three diverse generation tasks - mathematical program synthesis, lexically-constrained generation, and toxicity control - even when the corrector is much smaller than the base generator.
更多查看译文
关键词
Language models,text generation
AI 理解论文
溯源树
样例
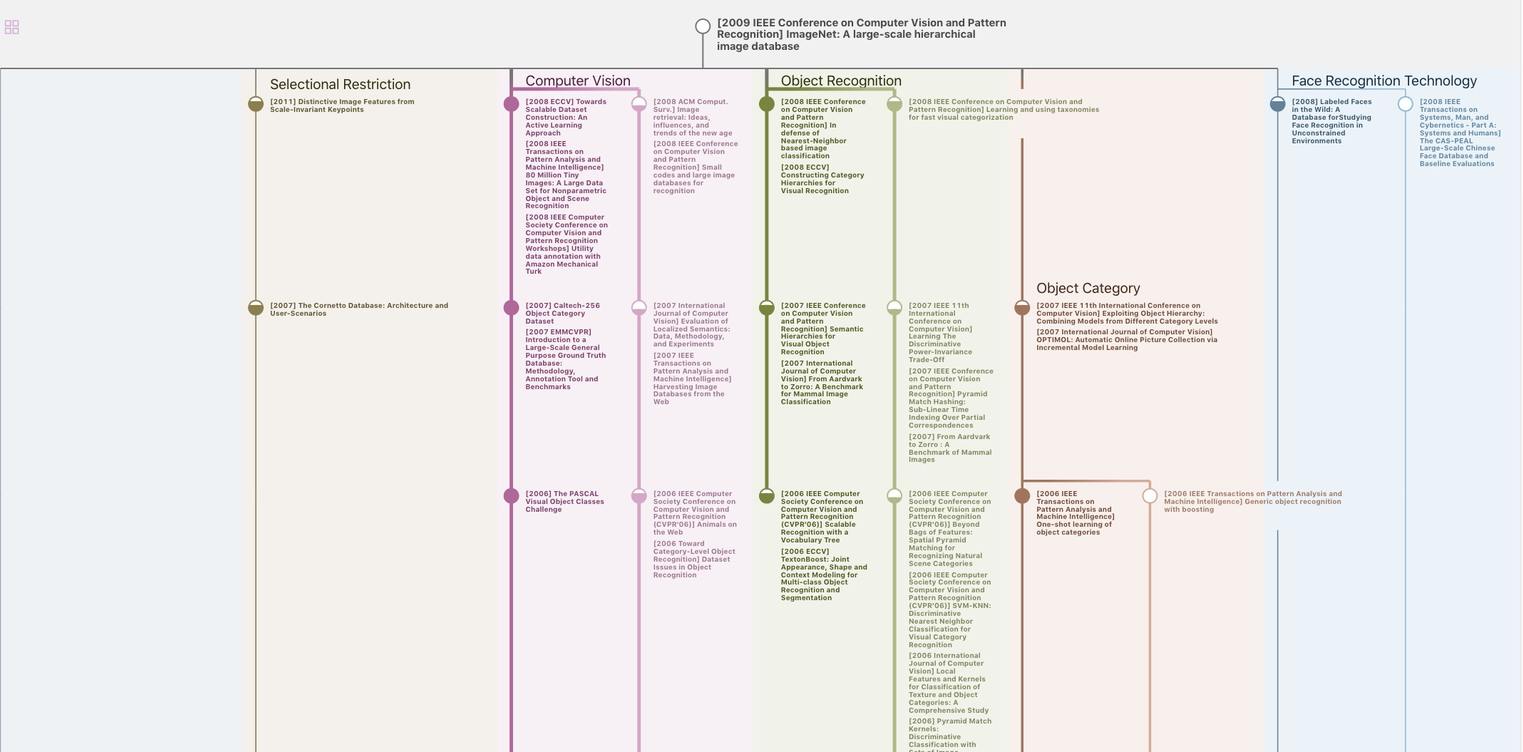
生成溯源树,研究论文发展脉络
Chat Paper
正在生成论文摘要