Unsupervised and Automatic Training Samples Selection Method
IGARSS 2022 - 2022 IEEE International Geoscience and Remote Sensing Symposium(2022)
摘要
In this paper, we propose a new unsupervised and automatic method for the selection of training samples. Thanks to this completely unsupervised method, the samples to be used in the learning task are selected according to objective criteria. Using biased or simplified training samples does not allow a rigorous explanation of the physical phenomena represented by the acquired data, especially in hyperspectral imaging. Furthermore, the use of training samples in learning task is of great importance and essential because they strongly affect the obtained results of any algorithm, when they are simplified or biased. The proposed method was tested on the public IRIS database and on synthetic and real hyperspectral images. Results show that the proposed method can not only select the training samples but also correct the biased or simplified ground truth.
更多查看译文
关键词
Unsupervised partitioning,training samples,bias,objective decision making,learning
AI 理解论文
溯源树
样例
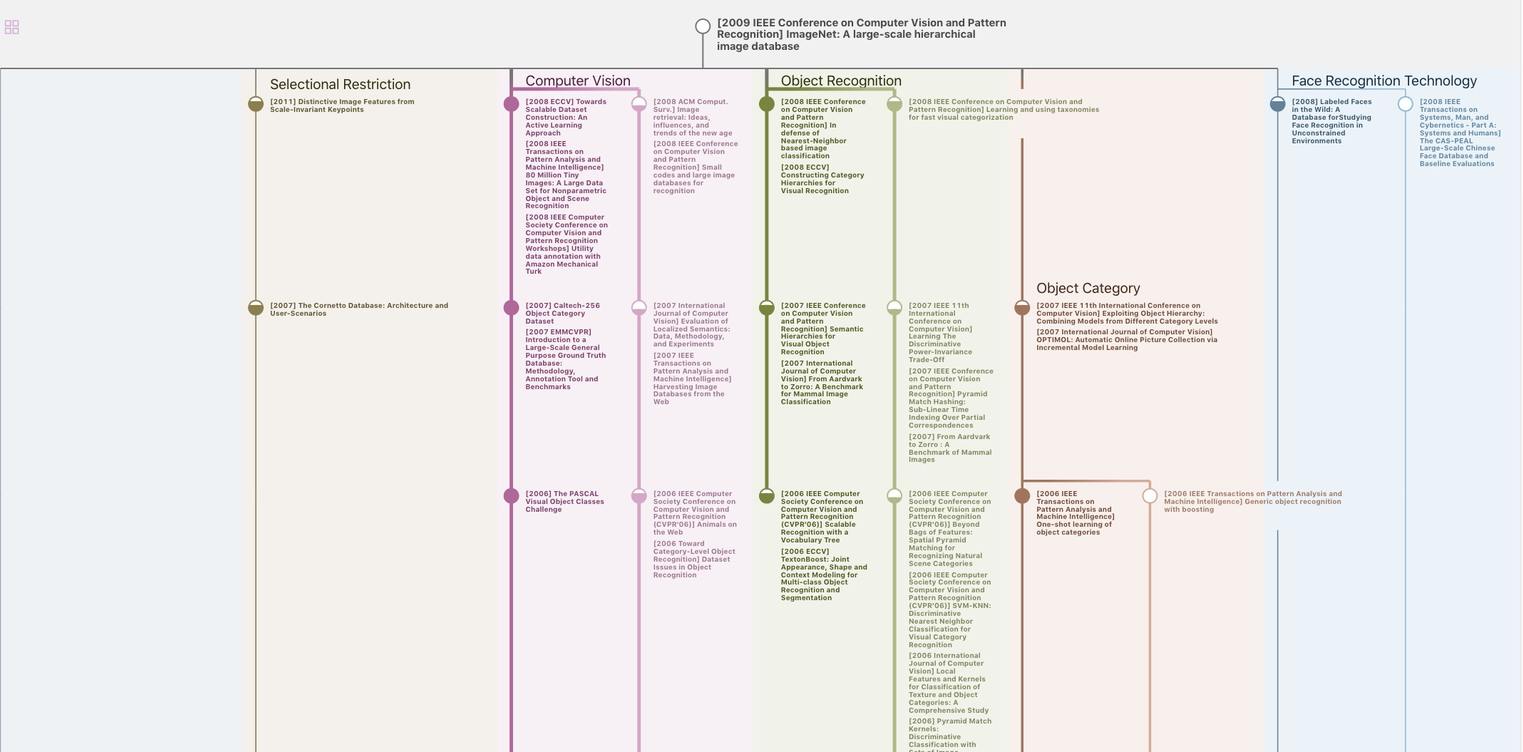
生成溯源树,研究论文发展脉络
Chat Paper
正在生成论文摘要