A Multi-Task Deep Learning Model for Population and LULC (M2PL-NET) Prediction with Scaling to a People Flow Grid
IGARSS 2022 - 2022 IEEE International Geoscience and Remote Sensing Symposium(2022)
摘要
This study attempts to create a comprehensive understanding of a regional population's residence and movements at high spatio-temporal resolution. Most approaches to estimating people flow focus purely on mobile GPS data, but this represents a relatively small and imbalanced user distribution across geographical regions. Hence, this paper proposes a new approach to address these issues by combining a multi-task deep learning satellite imagery technique with user GPS trajectories to predict dynamic population. Static population results demonstrate that the multi-task deep learning model performs reasonably well on the unseen data with Mean Absolute Error (MAE) of 3.15. Night-time predicted population was most highly correlated to observed static population, depicting the efficacy of the people flow grid.
更多查看译文
关键词
Deep learning,geographic information systems,geospatial analysis,remote sensing
AI 理解论文
溯源树
样例
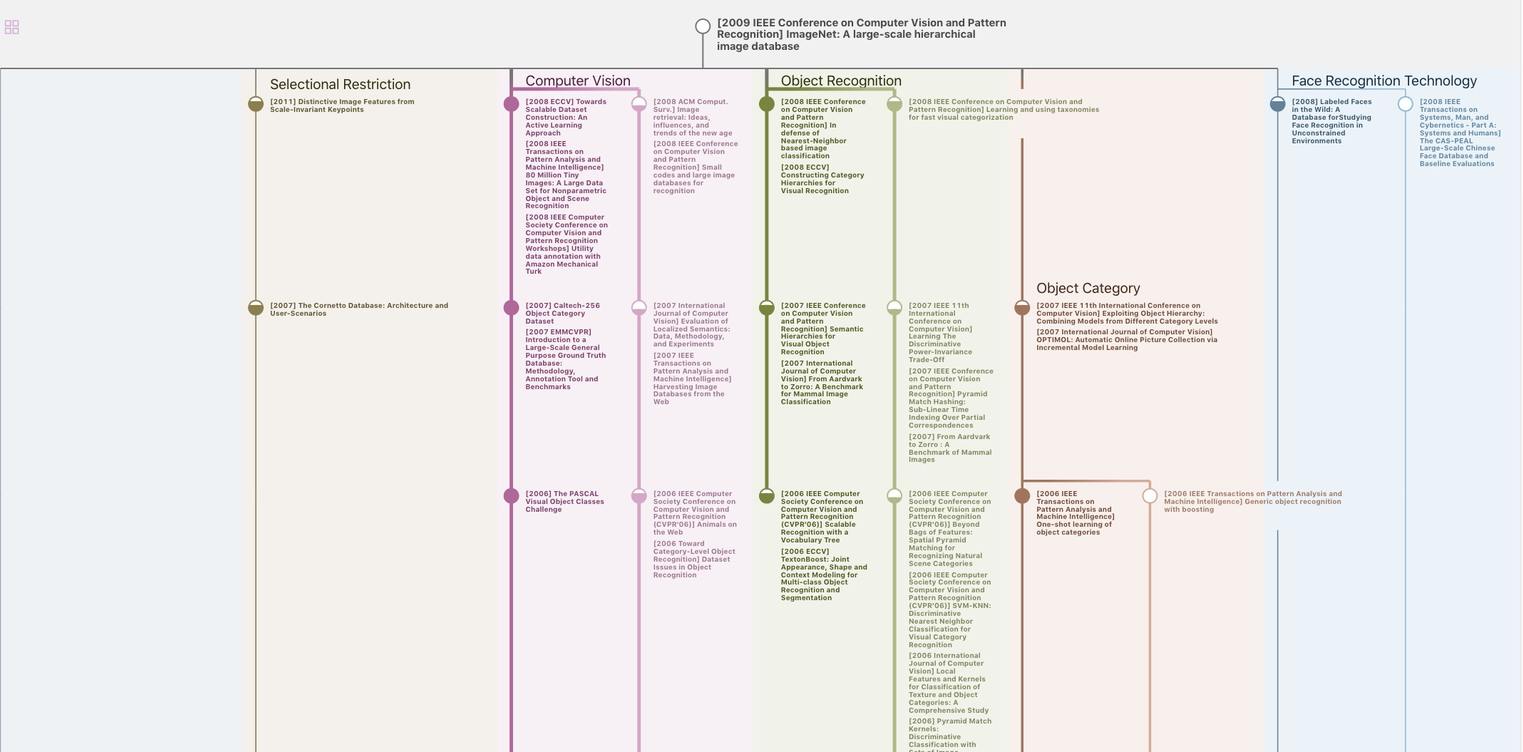
生成溯源树,研究论文发展脉络
Chat Paper
正在生成论文摘要