Multiscale dilated convolutional subdomain adaptation network with attention for unsupervised fault diagnosis of rotating machinery cross operating conditions
MEASUREMENT(2022)
摘要
Unsupervised cross-domain fault diagnosis research of rotating machinery has significant implications. However, some issues remain to be solved. For example, convolutional neural network cannot capture discriminative information in vibration signals from different scales. In addition, the extracted features should be selected to enhance informative features and suppress redundant features. Finally, global domain adaptation methods may cause different subdomains of source and target domains to be too close. To address these challenges, this paper proposes a multiscale dilated convolutional subdomain adaptation network with attention. Firstly, a multiscale dilated convolutional module is developed to extract fault features at different scales. Secondly, a squeeze-andexcitation attention mechanism is built to assign channel-level weights to these features. Finally, local maximum mean discrepancy is constructed to adapt corresponding subdomains of the two domains. The proposed method is applied to perform various unsupervised cross-domain fault diagnosis tasks, and the experimental results demonstrate its superior diagnostic performance.
更多查看译文
关键词
Multiscale features,Attention mechanism,Subdomain adaptation,Unsupervised cross-domain,Rotating machinery fault diagnosis
AI 理解论文
溯源树
样例
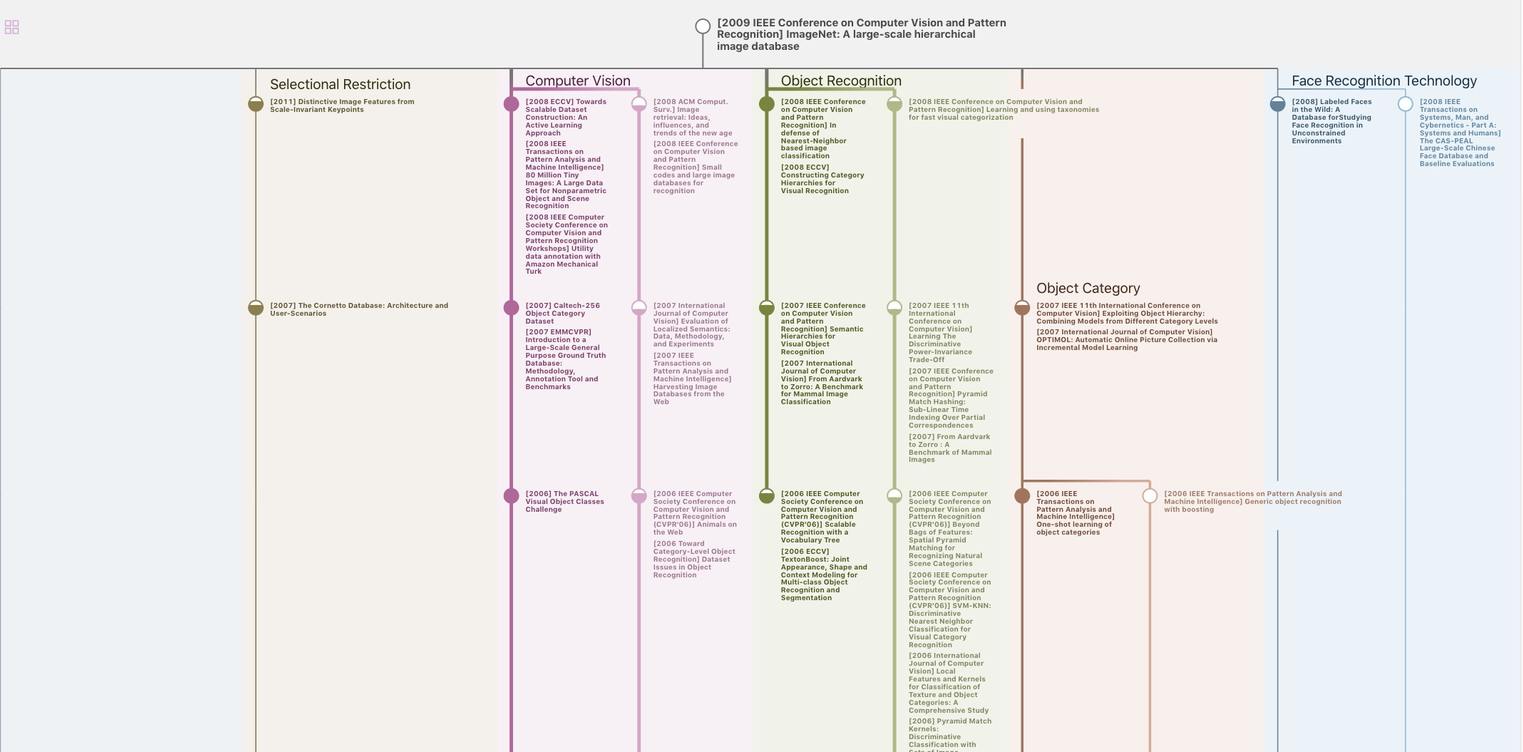
生成溯源树,研究论文发展脉络
Chat Paper
正在生成论文摘要