Semi-supervised transformable architecture search for feature distillation
PATTERN ANALYSIS AND APPLICATIONS(2022)
摘要
The designed method aims to perform image classification tasks efficiently and accurately. Different from the traditional CNN-based image classification methods, which are greatly affected by the number of labels and the depth of the network. Although the deep network can improve the accuracy of the model, the training process is usually time-consuming and laborious. We explained how to use only a few of labels, design a more flexible network architecture and combine feature distillation method to improve model efficiency while ensuring high accuracy. Specifically, we integrate different network structures into independent individuals to make the use of network structures more flexible. Based on knowledge distillation, we extract the channel features and establish a feature distillation connection from the teacher network to the student network. By comparing the experimental results with other related popular methods on commonly used data sets, the effectiveness of the method is proved. The code can be found at https://github.com/ZhangXinba/Semi_FD.
更多查看译文
关键词
Semi-supervised,Feature distillation,Transformable architecture search,Joint loss
AI 理解论文
溯源树
样例
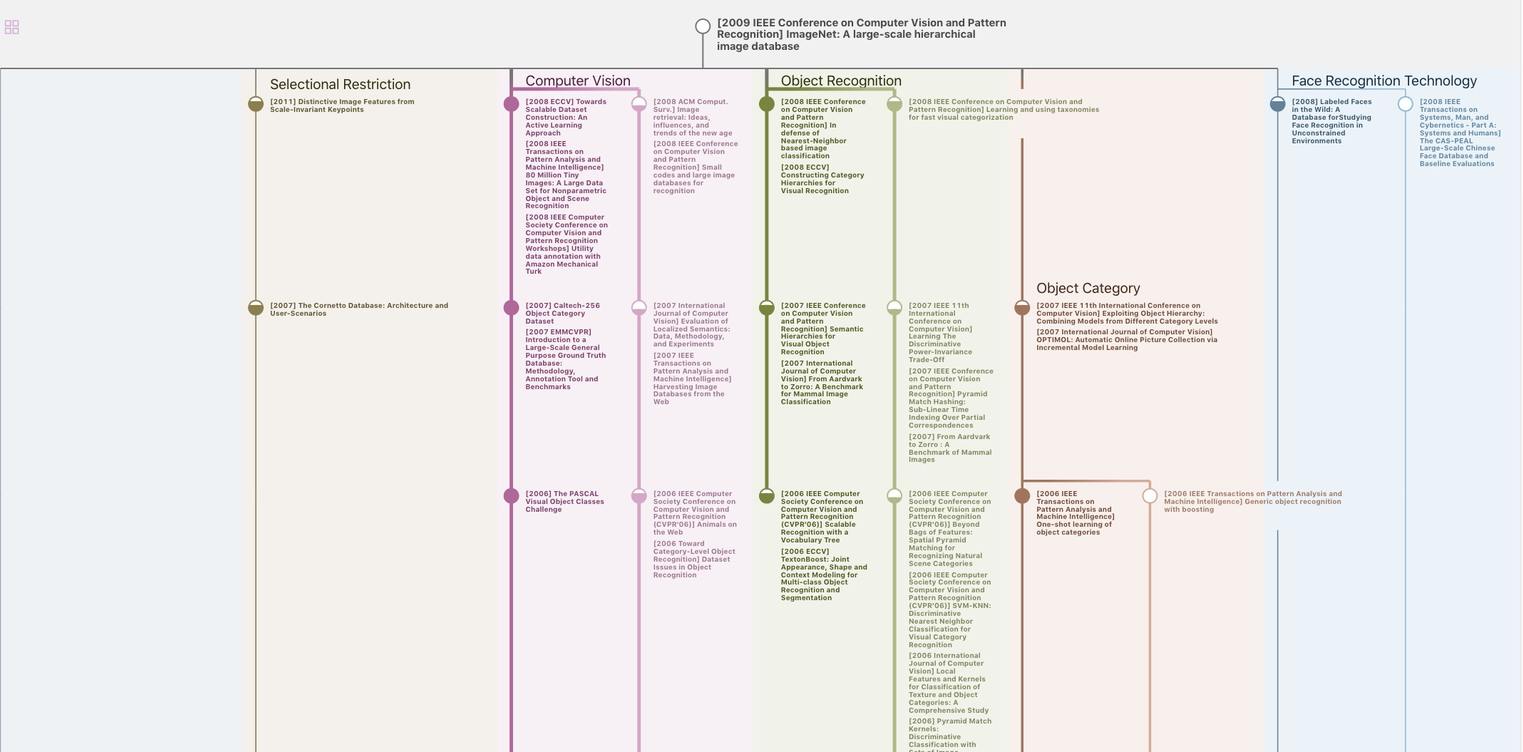
生成溯源树,研究论文发展脉络
Chat Paper
正在生成论文摘要