System Architecture and Software Stack for GDDR6-AiM
2022 IEEE Hot Chips 34 Symposium (HCS)(2022)
摘要
This poster presents system architecture, software stack, and performance analysis for SK hynix’s very first GDDR6-based processing-in-memory (PIM) product sample, called Accelerator-in-Memory (AiM).AiM is designed for the in-memory acceleration of matrix-vector product operations, which are commonly found in machine learning applications. The strength of AiM primarily comes from the two design factors, which are 1) all-bank operation support and 2) extended DRAM command set. All-bank operations allow AiM to fully utilize the abundant internal DRAM bandwidth, which makes it an attractive solution for memory-bound applications. The extended command set allows the host to address these new operations efficiently and provides a clean separation of concerns between the AiM architecture and its software stack design.We present a dedicated FPGA-based reference platform with a software stack, which is used to validate AiM design and evaluate its system-level performance. We also demonstrate FMC-based AiM extension cards that are compatible with the off-the-shelf FPGA boards and serve as an open research platform allowing potential collaborators and academic institutes to access our hardware and software systems.
更多查看译文
关键词
system architecture,GDDR6-based processing-in-memory product sample,accelerator-in-memory,in-memory acceleration,matrix-vector product operations,machine learning applications,all-bank operation support,internal DRAM bandwidth,memory-bound applications,extended command set,software stack design,system-level performance,FMC-based AiM extension cards,software systems,GDDR6-AiM architecture,FPGA-based reference platform
AI 理解论文
溯源树
样例
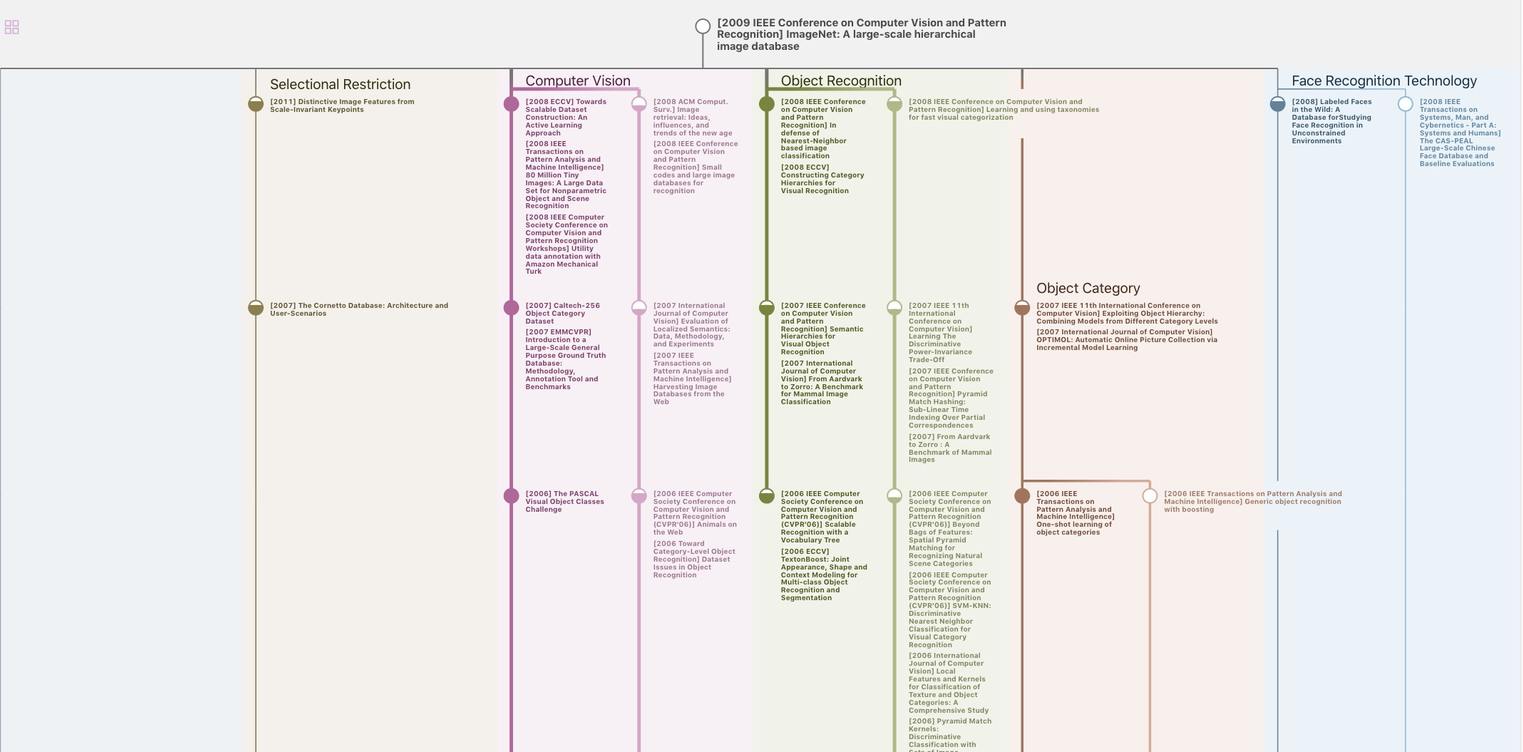
生成溯源树,研究论文发展脉络
Chat Paper
正在生成论文摘要