Diagnostic model optimization method for ADHD based on brain network analysis of resting-state fMRI images and transfer learning neural network.
Frontiers in human neuroscience(2022)
摘要
Attention deficit and hyperactivity disorder (ADHD) is a common inherited disease of the nervous system whose cause(s) and pathogenesis remain unclear. Currently, the diagnosis of ADHD is mainly based on clinical experience and guidelines that have laid out some diagnostic standards. Our study aimed to apply a learning-based classification method to assist the ADHD diagnosis based on high-dimensional resting-state fMRI. Our study selected the ADHD-200 Peking dataset of resting-state fMRI, which has an ADHD patient ( = 142) group and a typically developing control (TDC) healthy control ( = 102) group. We first used Pearson and partial correlation coefficients to perform functional connectivity (FC) analysis between ROIs. Then, the Pearson and partial correlation coefficient matrices were concatenated into a dual-channel feature to build a dual data channel as input to the transfer learning neural network (TLNN) architecture. Finally, we transferred the pretrained model from the auxiliary domain to our target domain and fine-tuned it. Based on the Pearson correlation coefficient, FC between ROIs was detected in 22 brain regions, including the fusiform gyrus, superior frontal gyrus, posterior superior temporal sulcus, inferior parietal lobule, anterior cingulate cortex, and parahippocampal gyrus. Based on the partial correlation coefficient, we found FC in the salient network, default network, sensory-motor network, dorsal attention network, and cerebellum network. With the TLNN architecture, we solved the problem of insufficient training data and improved the sensitivity of the classification method. When the VGG model (fine-tuned transfer strategy, 1,024 fully connected layers) was applied, the accuracy of TLNN classification ultimately reached 82%. Our study suggests that completing the training of the target domain by transferring the prior knowledge of the auxiliary domain is effective in solving the classification problem of small sample datasets. Based on prior knowledge of FC analysis, TLNN classification may assist ADHD diagnosis in a new way.
更多查看译文
关键词
attention deficit and hyperactivity disorder,brain network,classification,resting-state fMRI,transfer learning
AI 理解论文
溯源树
样例
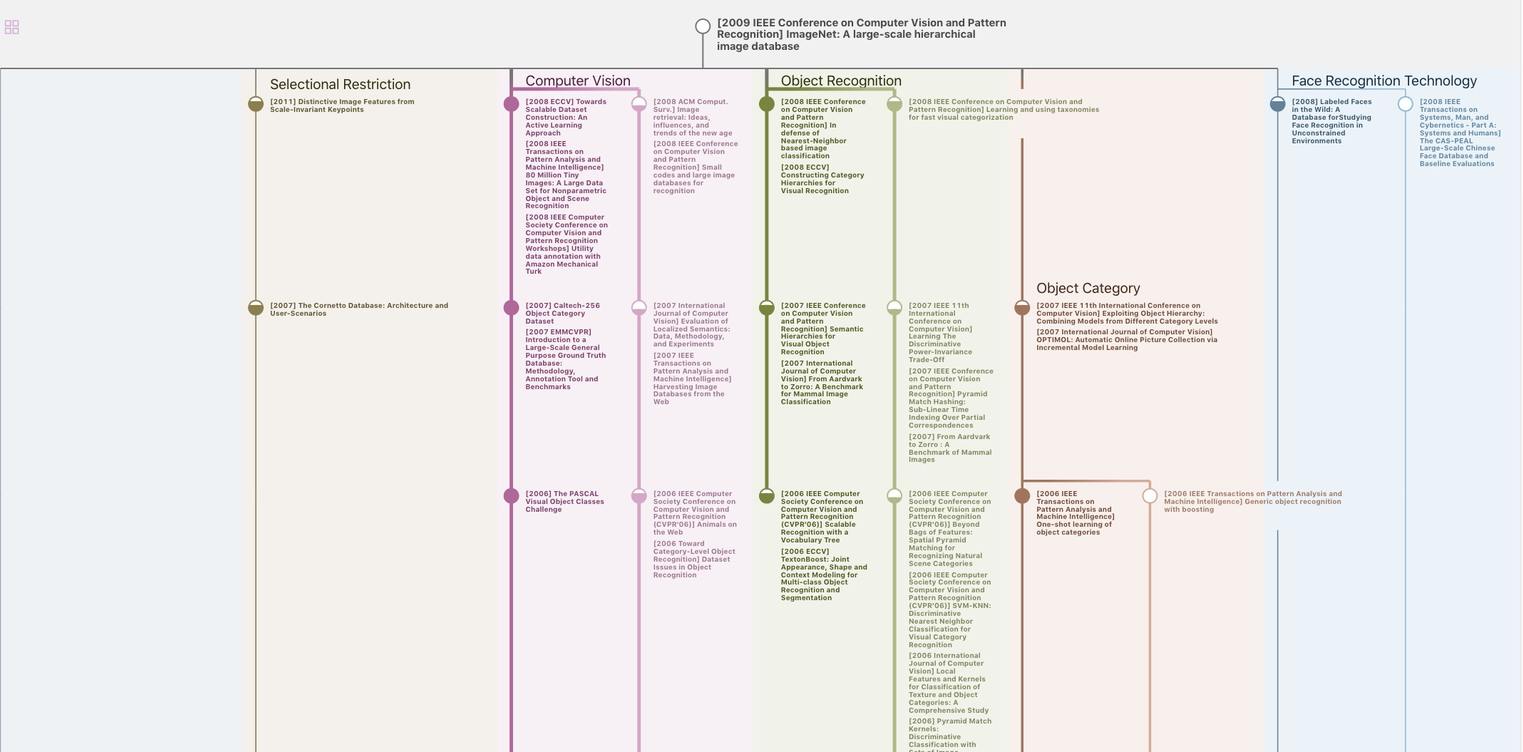
生成溯源树,研究论文发展脉络
Chat Paper
正在生成论文摘要