Knowledge Graph Augmentation Based on Tabular Data: A Case Study for Industrial Safety Inspection
Proceedings of the Sixth International Scientific Conference “Intelligent Information Technologies for Industry” (IITI’22)(2022)
摘要
The use of Semantic Web technologies, including knowledge graphs, is a widespread practice in the development of modern intelligent systems, information retrieval, and question answering. Knowledge graph engineering requires automation and improvement, including the use of various sources of information (e.g., databases, documents, conceptual models). Tables are one of the most accessible and common ways of storing and presenting information, as well as a valuable source of structured domain knowledge. In this paper, we propose to automate the process of extracting specific entities (facts) from tabular data for subsequent filling and augmentation of a target knowledge graph. A new approach is proposed for this purpose. The key feature of our approach is the semantic interpretation (annotation) of separate table elements. We present the main stages of this approach and a description of its implementation. We also conducted a case study on the task of designing a domain knowledge graph using the proposed approach in the field of industrial safety inspection of petrochemical equipment and technological complexes. The results obtained show the advisability of using our approach and software for this task.
更多查看译文
关键词
Semantic web, Knowledge graph, Semantic table interpretation, Table annotation, Entity extraction, Knowledge graph augmentation, Tabular data
AI 理解论文
溯源树
样例
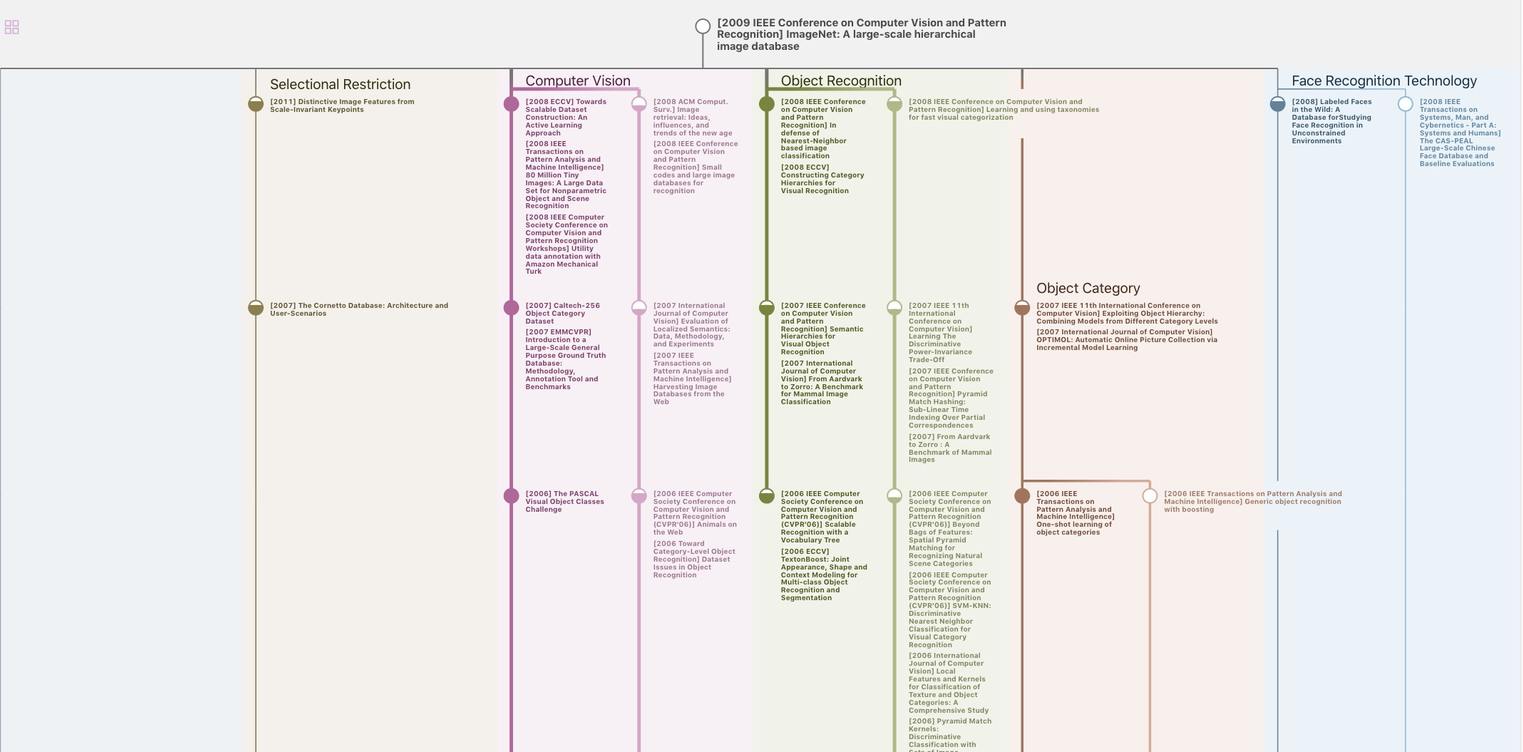
生成溯源树,研究论文发展脉络
Chat Paper
正在生成论文摘要