Improving Multi-generation Robustness of Learned Image Compression
arxiv(2022)
摘要
Benefit from flexible network designs and end-to-end joint optimization approach, learned image compression (LIC) has demonstrated excellent coding performance and practical feasibility in recent years. However, existing compression models suffer from serious multi-generation loss, which always occurs during image editing and transcoding. During the process of repeatedly encoding and decoding, the quality of the image will rapidly degrade, resulting in various types of distortion, which significantly limits the practical application of LIC. In this paper, a thorough analysis is carried out to determine the source of generative loss in successive image compression (SIC). We point out and solve the quantization drift problem that affects SIC, reversibility loss function as well as channel relaxation method are proposed to further reduce the generation loss. Experiments show that by using our proposed solutions, LIC can achieve comparable performance to the first compression of BPG even after 50 times reencoding without any change of the network structure.
更多查看译文
关键词
learned image compression,robustness,multi-generation
AI 理解论文
溯源树
样例
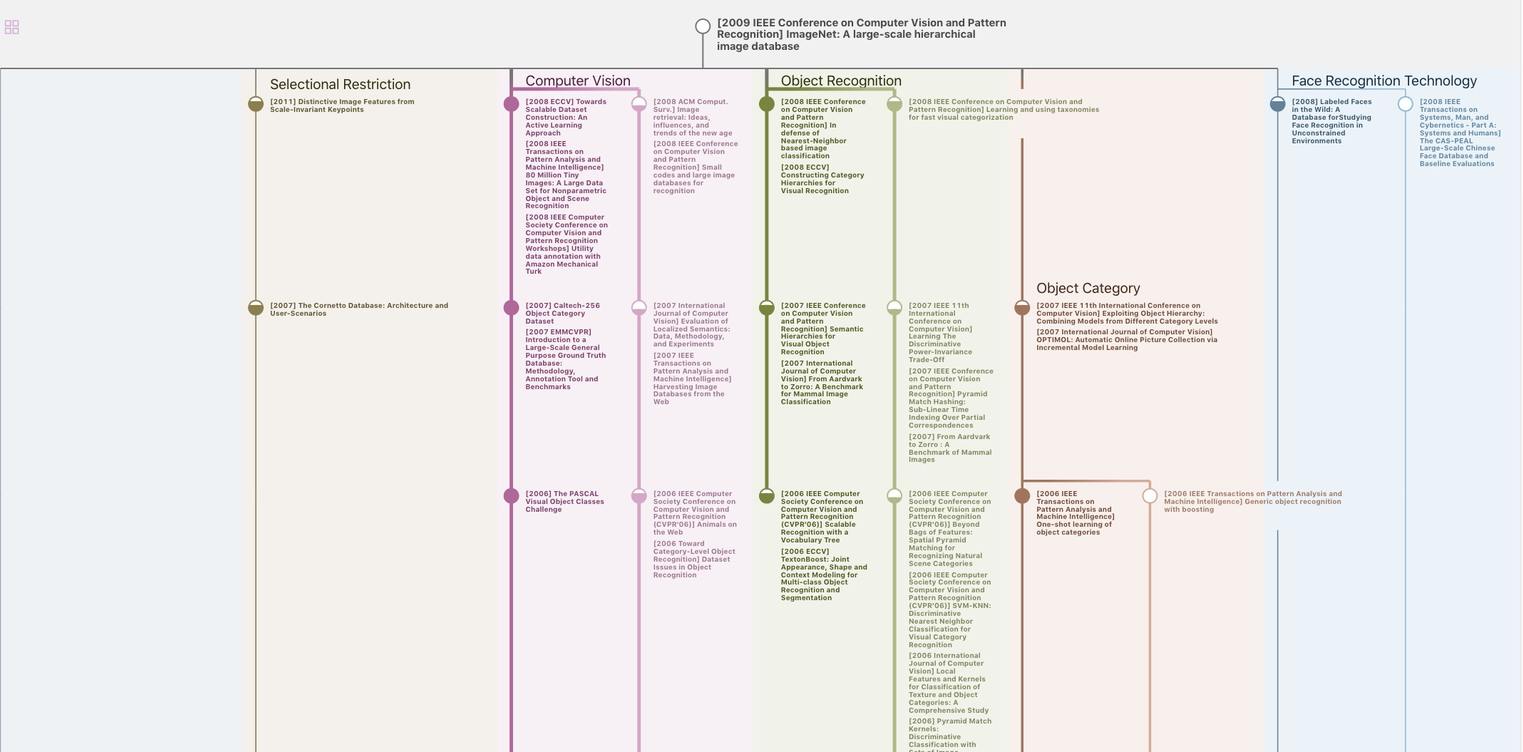
生成溯源树,研究论文发展脉络
Chat Paper
正在生成论文摘要