Detection of changes in the dynamics of thermonuclear plasmas to improve the prediction of disruptions
Nonlinear Dynamics(2022)
摘要
In particular circumstances, nonlinear systems can collapse suddenly and abruptly. Anomalous detection is therefore an important task. Unfortunately, many phenomena occurring in complex systems out of equilibrium, such as disruptions in tokamak thermonuclear plasmas, cannot be modelled from first principles in real-time compatible form and therefore data-driven, machine learning techniques are often deployed. A typical issue, for training these tools, is the choice of the most adequate examples. Determining the intervals, in which the anomalous behaviours manifest themselves, is consequently a challenging but essential objective. In this paper, a series of methods are deployed to determine when the plasma dynamics of the tokamak configuration varies, indicating the onset of drifts towards a form of collapse called disruption. The techniques rely on changes in various quantities derived from the time series of the main signals: from the embedding dimensions to the properties of recurrence plots and to indicators of transition to chaotic dynamics. The methods, being mathematically completely independent, provide quite robust indications about the intervals, in which the various signals manifest a pre-disruptive behaviour. Consequently, the signal samples, to be used for supervised machine learning predictors, can be defined precisely, on the basis of the plasma dynamics. This information can improve significantly not only the performance of machine learning classifiers but also the physical understanding of the phenomenon.
更多查看译文
关键词
Embedding dimension,Recurrence plots,Chaos onset,Nuclear fusion,Plasma disruptions
AI 理解论文
溯源树
样例
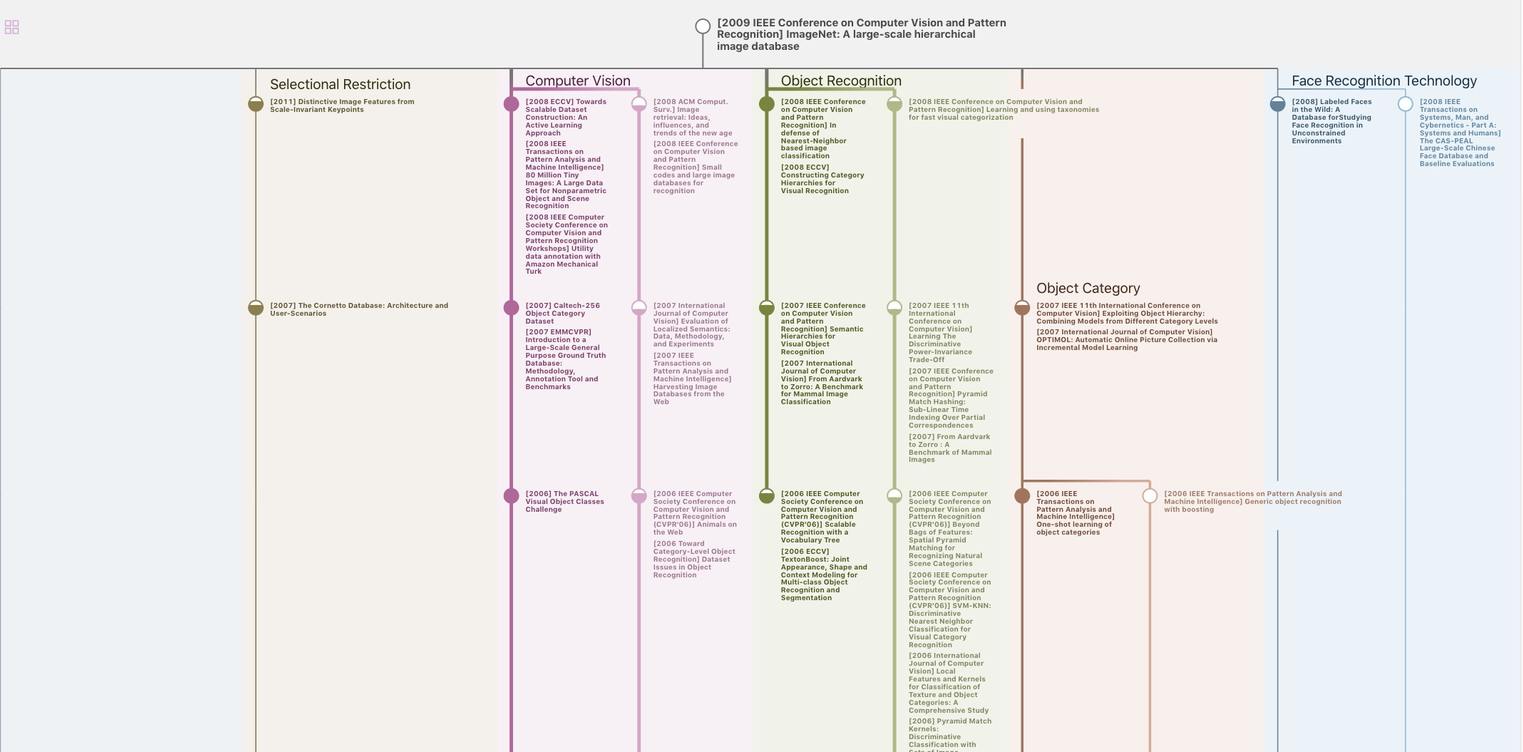
生成溯源树,研究论文发展脉络
Chat Paper
正在生成论文摘要