Local Model Reconstruction Attacks in Federated Learning and their Uses
arxiv(2022)
摘要
In this paper, we initiate the study of local model reconstruction attacks for federated learning, where a honest-but-curious adversary eavesdrops the messages exchanged between a targeted client and the server, and then reconstructs the local/personalized model of the victim. The local model reconstruction attack allows the adversary to trigger other classical attacks in a more effective way, since the local model only depends on the client's data and can leak more private information than the global model learned by the server. Additionally, we propose a novel model-based attribute inference attack in federated learning leveraging the local model reconstruction attack. We provide an analytical lower-bound for this attribute inference attack. Empirical results using real world datasets confirm that our local reconstruction attack works well for both regression and classification tasks. Moreover, we benchmark our novel attribute inference attack against the state-of-the-art attacks in federated learning. Our attack results in higher reconstruction accuracy especially when the clients' datasets are heterogeneous. Our work provides a new angle for designing powerful and explainable attacks to effectively quantify the privacy risk in FL.
更多查看译文
关键词
federated learning,attacks,model
AI 理解论文
溯源树
样例
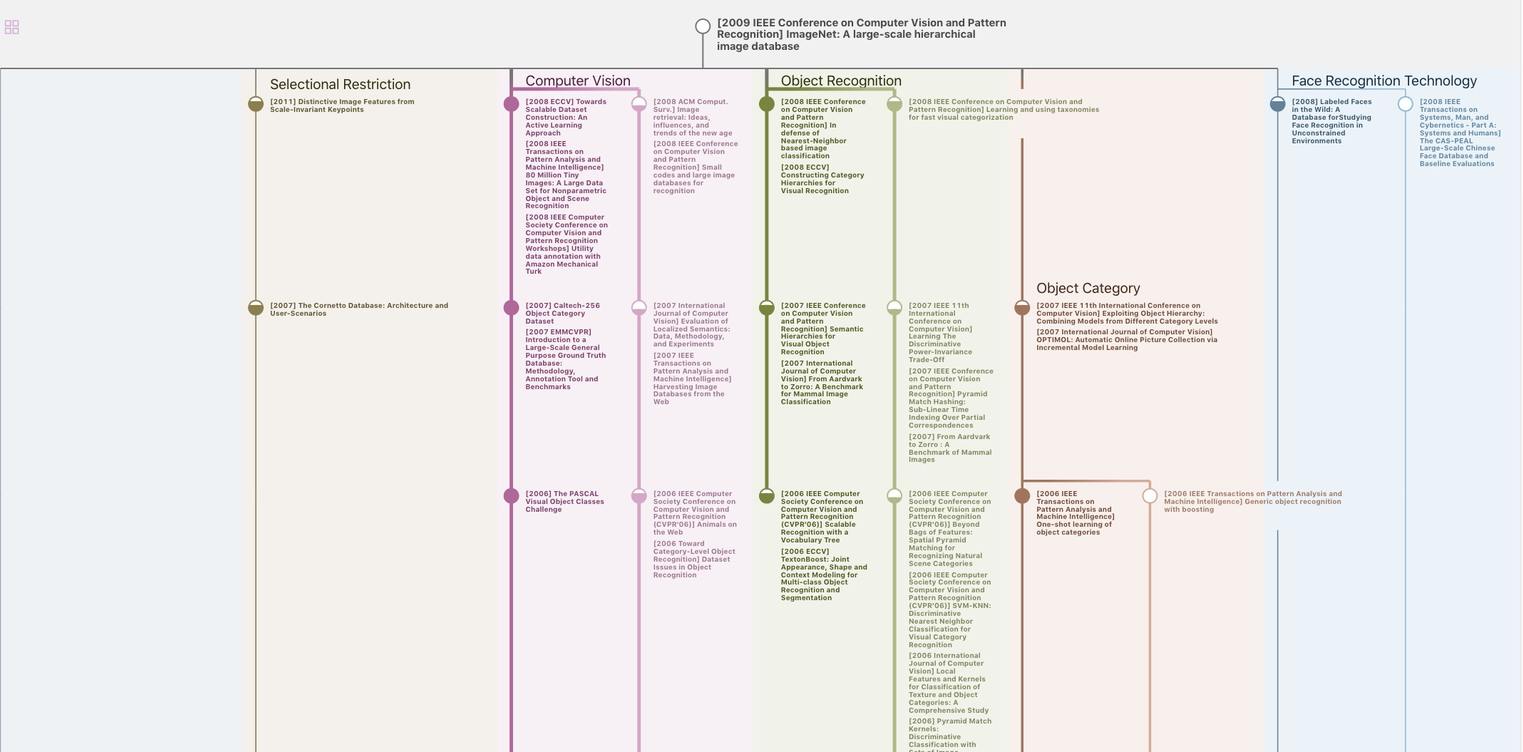
生成溯源树,研究论文发展脉络
Chat Paper
正在生成论文摘要