Instance-Optimal Differentially Private Estimation
arxiv(2022)
摘要
In this work, we study local minimax convergence estimation rates subject to $\epsilon$-differential privacy. Unlike worst-case rates, which may be conservative, algorithms that are locally minimax optimal must adapt to easy instances of the problem. We construct locally minimax differentially private estimators for one-parameter exponential families and estimating the tail rate of a distribution. In these cases, we show that optimal algorithms for simple hypothesis testing, namely the recent optimal private testers of Canonne et al. (2019), directly inform the design of locally minimax estimation algorithms.
更多查看译文
关键词
estimation,instance-optimal
AI 理解论文
溯源树
样例
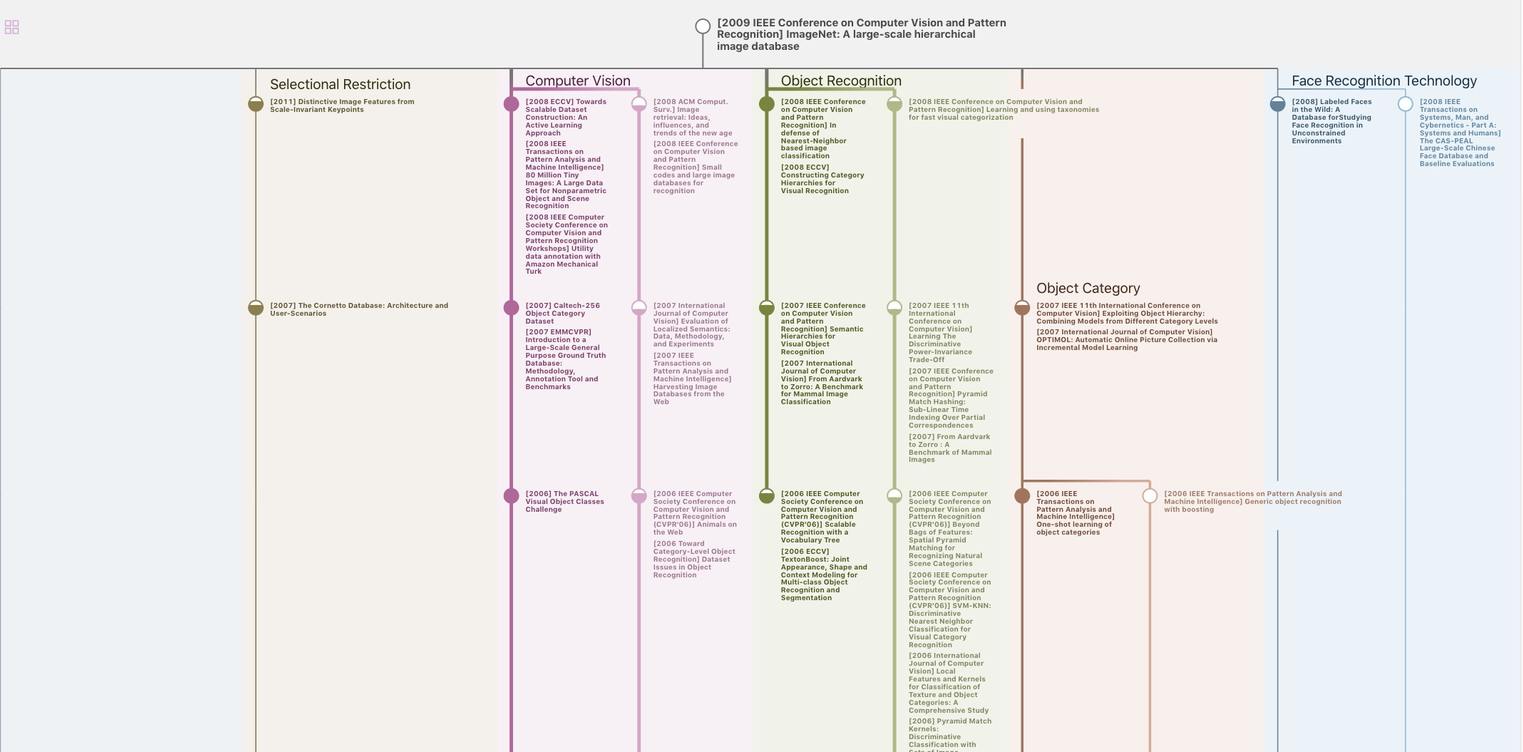
生成溯源树,研究论文发展脉络
Chat Paper
正在生成论文摘要