Decentralized Federated Learning via Non-Coherent Over-the-Air Consensus
ICC 2023 - IEEE INTERNATIONAL CONFERENCE ON COMMUNICATIONS(2023)
摘要
This paper presents NCOTA-DGD, a Decentralized Gradient Descent (DGD) algorithm that combines local gradient descent with a novel Non-Coherent Over-The-Air (NCOTA) consensus scheme to solve distributed machine-learning problems over wirelessly-connected systems. NCOTA-DGD leverages the waveform superposition properties of the wireless channels: it enables simultaneous transmissions under half-duplex constraints, by mapping local optimization signals to a mixture of preamble sequences, and consensus via non-coherent combining at the receivers. NCOTA-DGD operates without channel state information at transmitters and receivers, and leverages the average channel pathloss to mix signals, without explicit knowledge of the mixing weights (typically known in consensus-based optimization algorithms). It is shown both theoretically and numerically that, for smooth and strongly-convex problems with fixed consensus and learning stepsizes, the updates of NCOTA-DGD converge in Euclidean distance to the global optimum with rate O(K) for a target of K iterations. NCOTA-DGD is evaluated numerically over a logistic regression problem, showing faster convergence vis-a-vis running time than implementations of the classical DGD algorithm over digital and analog orthogonal channels.
更多查看译文
关键词
federated learning,non-coherent,over-the-air
AI 理解论文
溯源树
样例
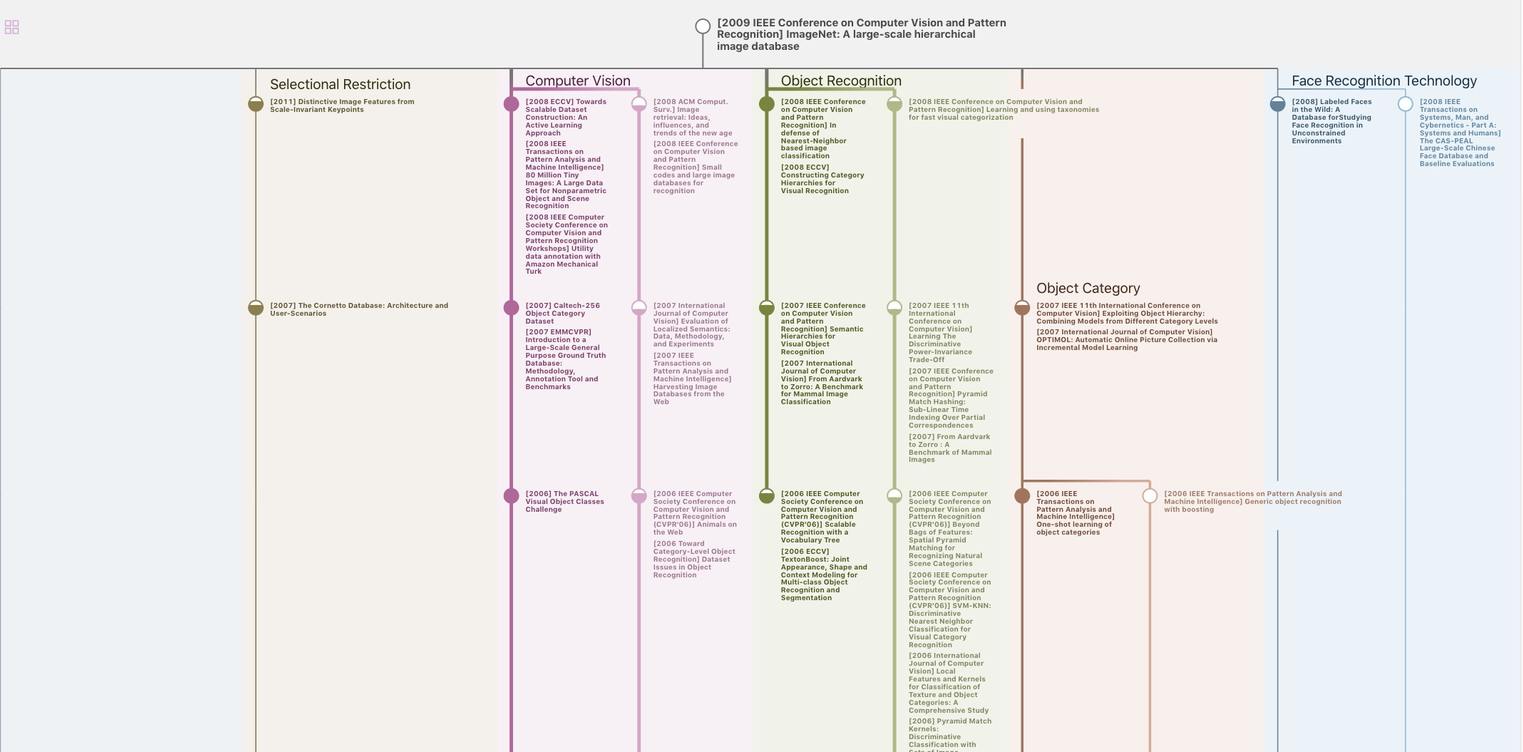
生成溯源树,研究论文发展脉络
Chat Paper
正在生成论文摘要