Feature Necessity & Relevancy in ML Classifier Explanations
arxiv(2023)
摘要
Given a machine learning (ML) model and a prediction, explanations can be defined as sets of features which are sufficient for the prediction. In some applications, and besides asking for an explanation, it is also critical to understand whether sensitive features can occur in some explanation, or whether a non-interesting feature must occur in all explanations. This paper starts by relating such queries respectively with the problems of relevancy and necessity in logic-based abduction. The paper then proves membership and hardness results for several families of ML classifiers. Afterwards the paper proposes concrete algorithms for two classes of classifiers. The experimental results confirm the scalability of the proposed algorithms.
更多查看译文
关键词
ml classifier explanations,feature necessity,relevancy
AI 理解论文
溯源树
样例
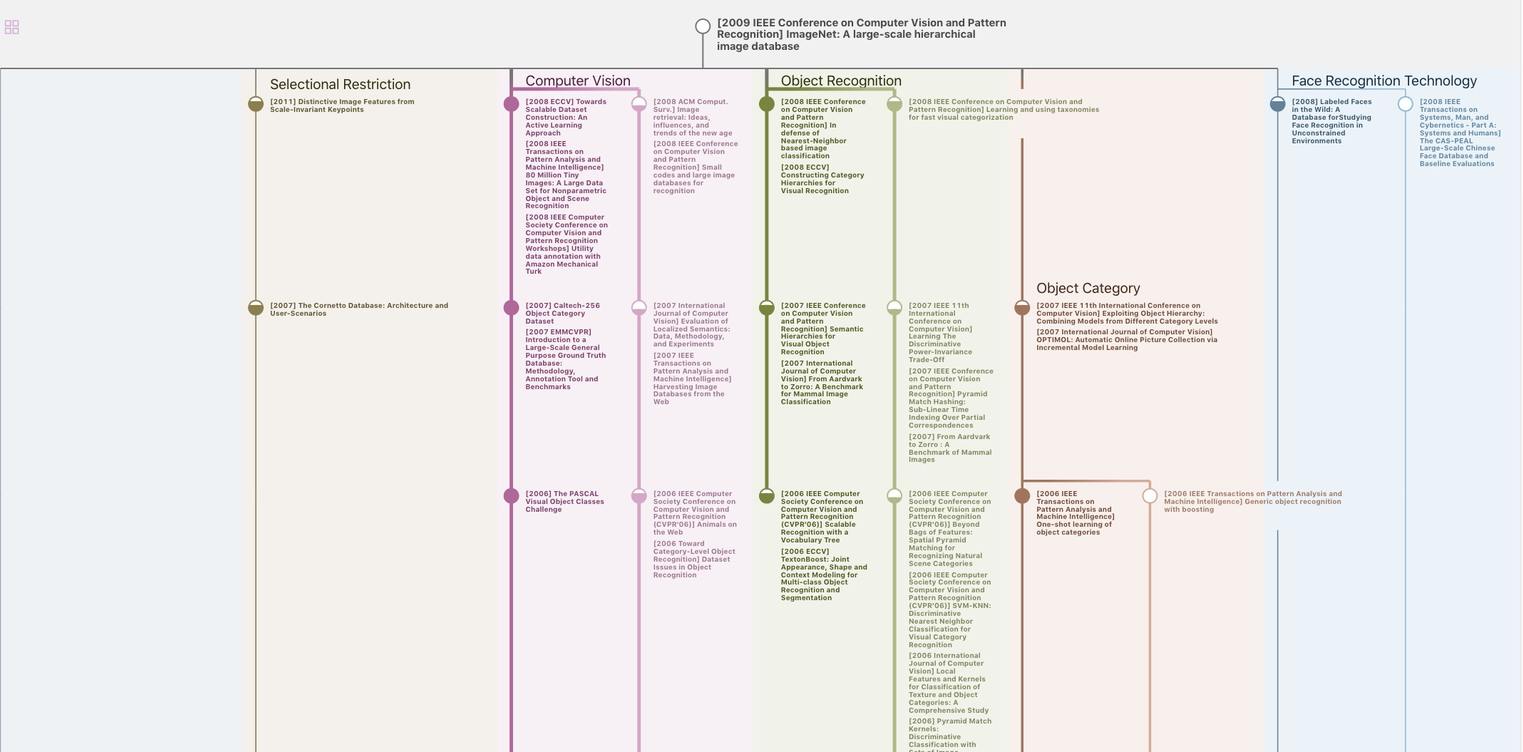
生成溯源树,研究论文发展脉络
Chat Paper
正在生成论文摘要