BayesianPUFNet: Training Sample Efficient Modeling Attack for Physically Unclonable Functions
IEICE Transactions on Fundamentals of Electronics, Communications and Computer Sciences(2023)
摘要
This paper proposes a deep neural network named BayesianPUFNet that can achieve high prediction accuracy even with few challenge-response pairs (CRPs) available for training. Generally, modeling attacks are a vulnerability that could compromise the authenticity of physi-cally unclonable functions (PUFs); thus, various machine learning methods including deep neural networks have been proposed to assess the vulnerabil-ity of PUFs. However, conventional modeling attacks have not considered the cost of CRP collection and analyzed attacks based on the assumption that sufficient CRPs were available for training; therefore, previous studies may have underestimated the vulnerability of PUFs. Herein, we show that the application of Bayesian deep neural networks that incorporate Bayesian statistics can provide accurate response prediction even in situations where sufficient CRPs are not available for learning. Numerical experiments show that the proposed model uses only half the CRP to achieve the same re-sponse prediction as that of the conventional methods. Our code is openly available on https://github.com/bayesian-puf-net/bayesian-puf-net.git.
更多查看译文
关键词
physically unclonable functions,attack
AI 理解论文
溯源树
样例
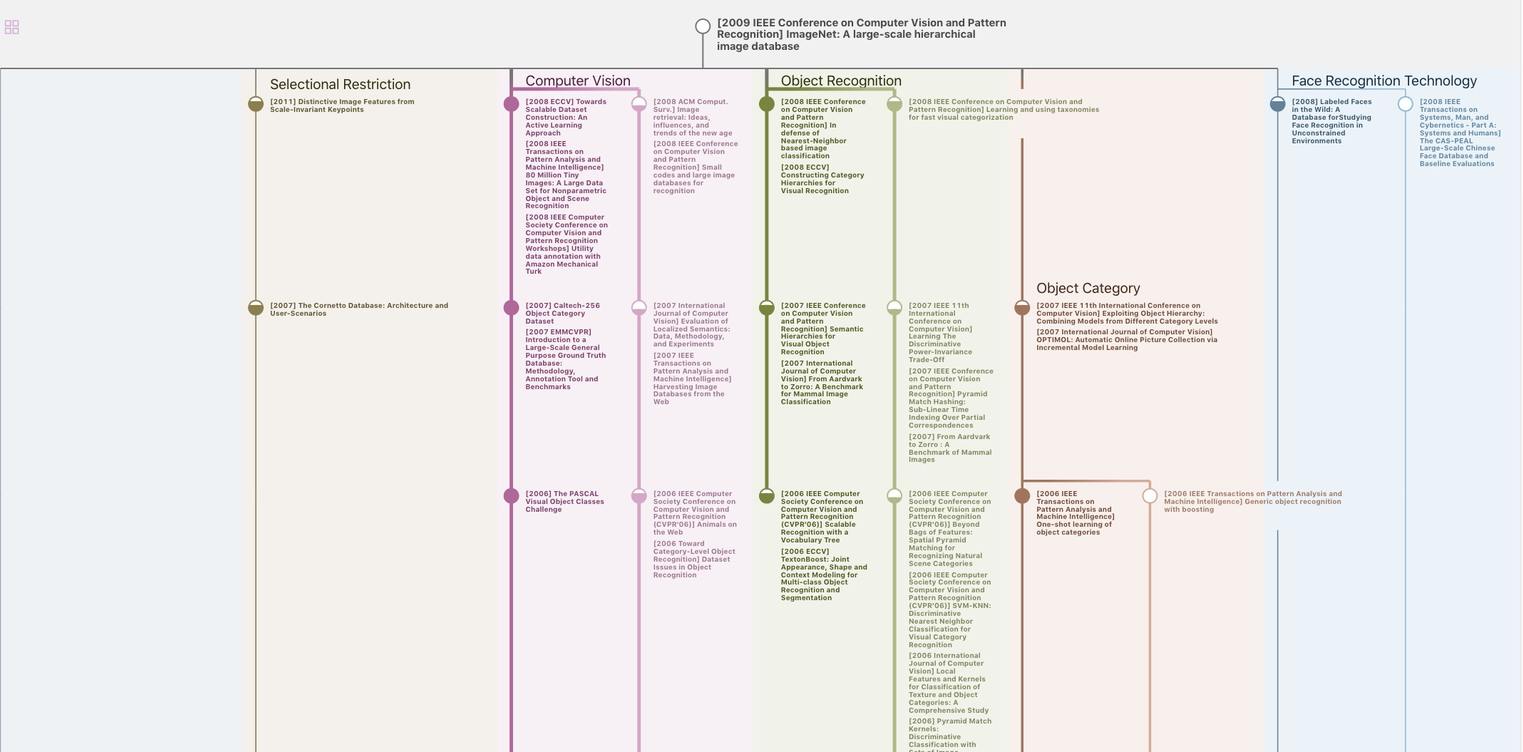
生成溯源树,研究论文发展脉络
Chat Paper
正在生成论文摘要