Stochastic Optimization Workflow for Non-Uniform Coarsening of Simulation Grids and Location and Sequencing of Wells
Day 2 Tue, November 01, 2022(2022)
摘要
Abstract An adaptive differential evolution (DE) optimization algorithm and workflow are presented for non-uniform coarsening (NUC) of simulation grids resulting in smaller and faster models that maintain the key physics of the original model. The workflow presented here fully automates the grid generation process and results in a better NUC grid. The algorithm presented can handle discrete and continuous NUC grid generation parameters. We also use the DE optimization method for location and sequencing of wells. A typical NUC grid generation process splits the reservoir into several regions: property preservation, near-well, far-well, etc. Several techniques for region splitting, e.g. time-of-flight (TOF), distance from wells, mixture of the two, etc. are used. The coarsening process has several types of parameters: continuous, binary, and integer. The resultant optimization problem is a mixed-integer nonlinear optimization problem which is solved using the modified adaptive differential evolution method. Two methods are used to evaluate coarse grid quality. They are flow diagnostics, based on single-phase steady-state flow, and multiphase flow simulation. Well allocation factor differences computed from flow diagnostics and three phase production data differences computed from multiphase simulation are used in the objective function. These two objective functions are used in a two-stage optimization - in the first stage flow diagnostic differences drive the coarse grid generation process. A technique to prescreen NUC grids is presented for the second stage. It significantly reduced the time used in getting optimal NUC grids for all the cases that were studied. The NUC grid results clearly show the convergence of coarse grid results towards the fine grid results. Preserving fluid contacts in the coarse grid is found to be critical in getting an optimal NUC grid. A real field example with dozens of wells are presented that show the effectiveness of the adaptive differential evolution optimization workflow in achieving an optimal drilling schedule. Another set of examples demonstrate how the ability to move well locations within a user defined region helps us to further maximize the objective function. The novelty of this adaptive differential evolution optimization workflow is the ability to automate the NUC grid parameter selection and the grid generation process; thus saving several days for a practicing engineer. Another novelty of the optimization algorithm is the ability to fully automate the process of scenario generation and simulation, thus saving time from manual exploration of the range of parameters.
更多查看译文
关键词
simulation grids,optimization,wells,non-uniform
AI 理解论文
溯源树
样例
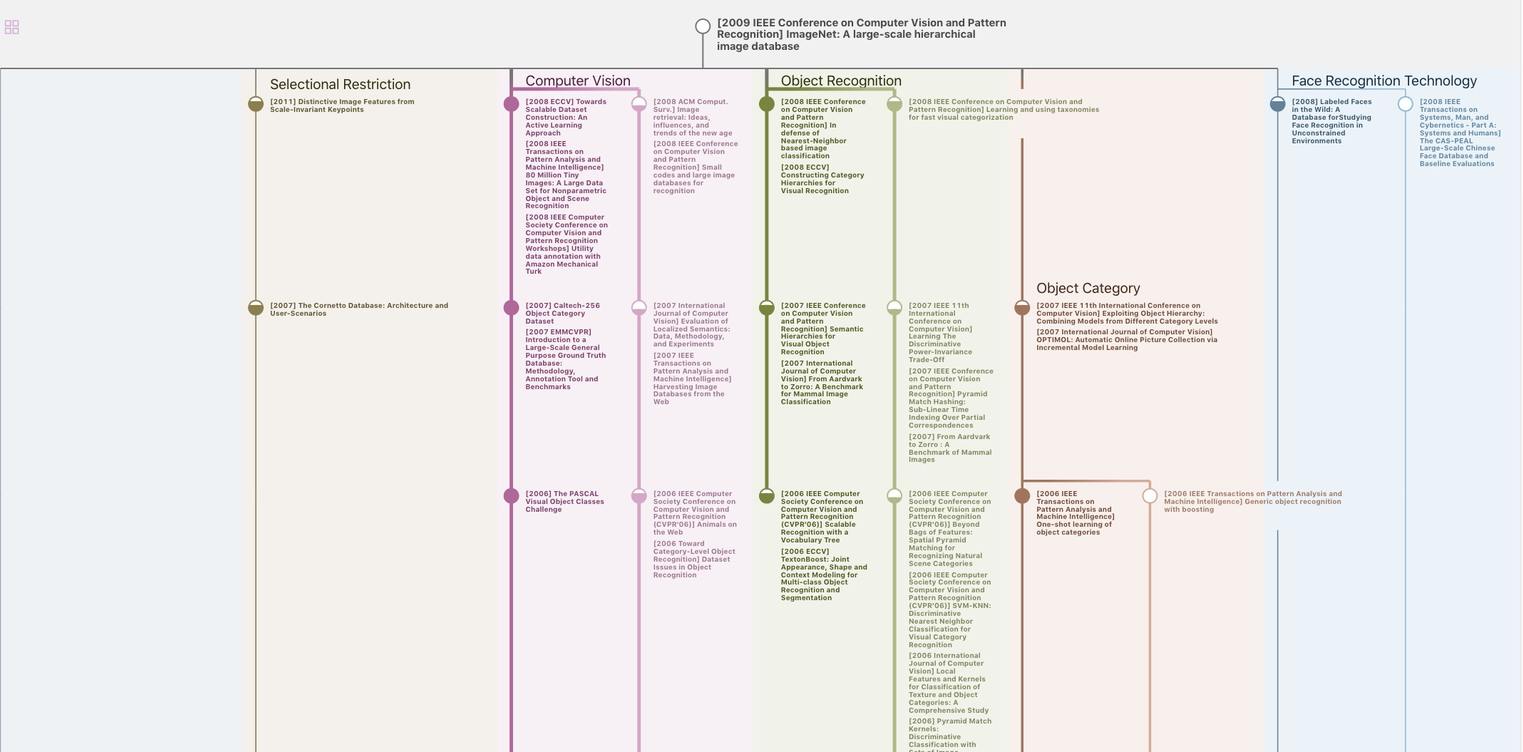
生成溯源树,研究论文发展脉络
Chat Paper
正在生成论文摘要