Provenance-based explanations: Are they useful?
ACM Conference on Management of Data(2022)
摘要
As machine-learning models and data-driven decisions continue to be on the rise, interpreting and explaining their results has recently gained increased interest as well. In this context, provenance has often been advertised as metadata that can be used to provide explanations about some data processing. Advantages of such explanations are possibly many: they may help data engineers get a better sense of their data processing pipelines, offer transparency to auditors or end users, and ultimately, improve the trust one has in the data processing. While today, provenance can be efficiently captured for a large variety of data processing pipelines, systematic research on how useful explanations based on provenance actually is scarce. This paper advocates that there is an urgent need to study in which settings what type of provenance-based explanation is useful, given the somewhat mitigated results that have become available for explanations of machine learning results. This is underlined by a first study that shows that provenance-based explanations of record linkage decisions may not have the desired effect.
更多查看译文
AI 理解论文
溯源树
样例
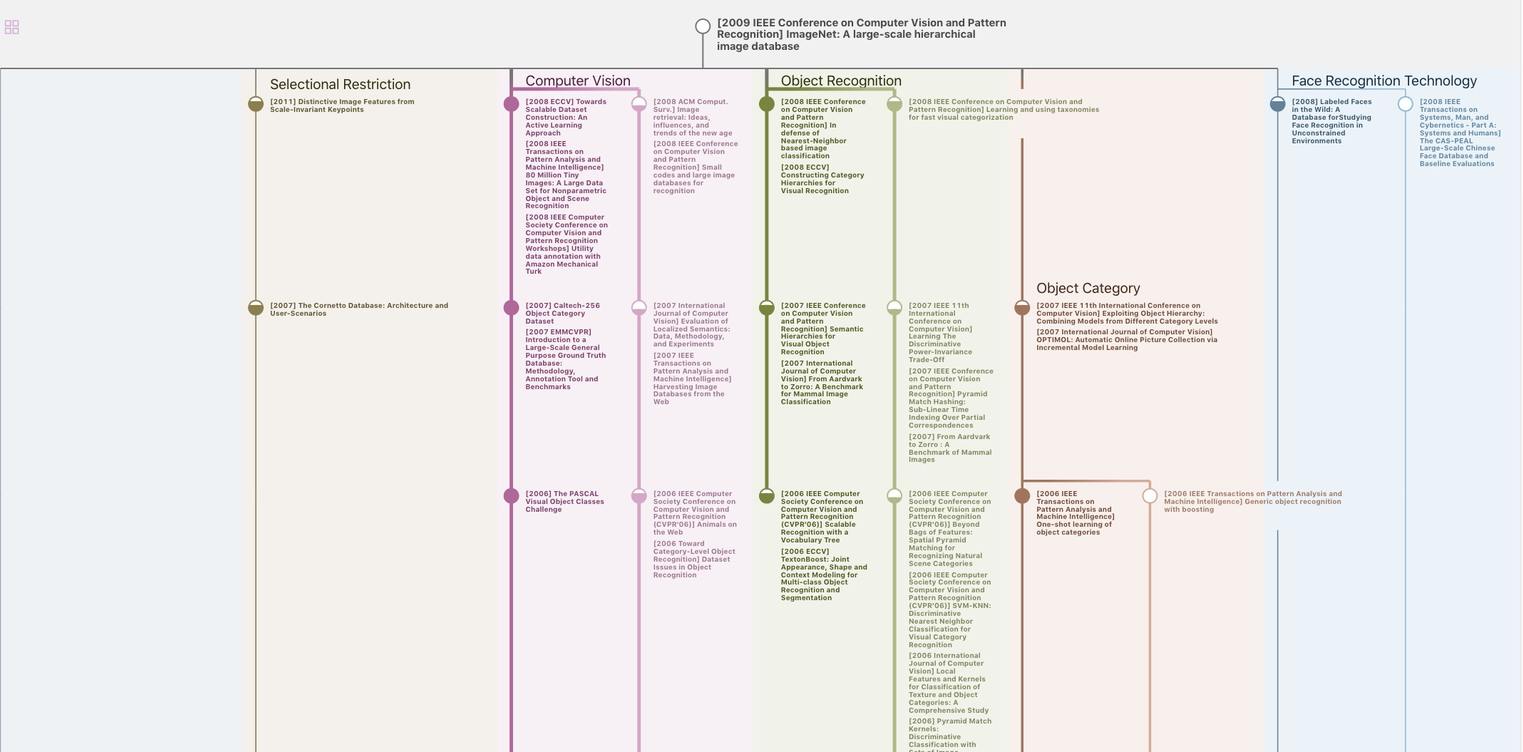
生成溯源树,研究论文发展脉络
Chat Paper
正在生成论文摘要