Addressing the Challenges and Barriers to the Integration of Machine Learning into Clinical Practice: An Innovative Method to Hybrid Human-Machine Intelligence
SENSORS(2022)
摘要
Machine learning (ML) models have proven their potential in acquiring and analyzing large amounts of data to help solve real-world, complex problems. Their use in healthcare is expected to help physicians make diagnoses, prognoses, treatment decisions, and disease outcome predictions. However, ML solutions are not currently deployed in most healthcare systems. One of the main reasons for this is the provenance, transparency, and clinical utility of the training data. Physicians reject ML solutions if they are not at least based on accurate data and do not clearly include the decision-making process used in clinical practice. In this paper, we present a hybrid human-machine intelligence method to create predictive models driven by clinical practice. We promote the use of quality-approved data and the inclusion of physician reasoning in the ML process. Instead of training the ML algorithms on the given data to create predictive models (conventional method), we propose to pre-categorize the data according to the expert physicians' knowledge and experience. Comparing the results of the conventional method of ML learning versus the hybrid physician-algorithm method showed that the models based on the latter can perform better. Physicians' engagement is the most promising condition for the safe and innovative use of ML in healthcare.
更多查看译文
关键词
human-machine collaboration,machine learning,physician-algorithm collaboration,clinical decision-making,personalized medicine,multiple sclerosis
AI 理解论文
溯源树
样例
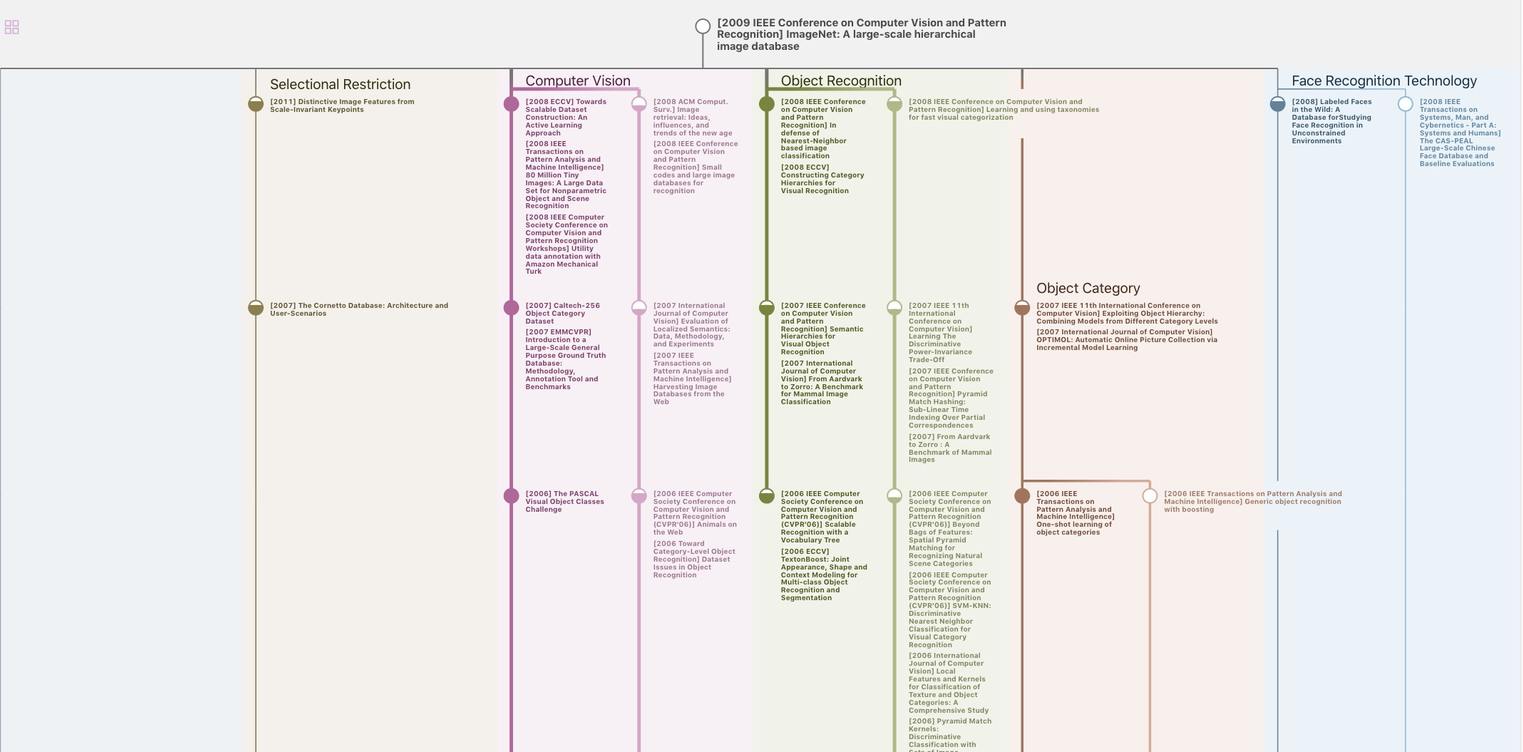
生成溯源树,研究论文发展脉络
Chat Paper
正在生成论文摘要