Model training periods impact estimation of COVID-19 incidence from wastewater viral loads.
The Science of the total environment(2023)
摘要
Wastewater-based epidemiology (WBE) has been deployed broadly as an early warning tool for emerging COVID-19 outbreaks. WBE can inform targeted interventions and identify communities with high transmission, enabling quick and effective responses. As the wastewater (WW) becomes an increasingly important indicator for COVID-19 transmission, more robust methods and metrics are needed to guide public health decision-making. This research aimed to develop and implement a mathematical framework to infer incident cases of COVID-19 from SARS-CoV-2 levels measured in WW. We propose a classification scheme to assess the adequacy of model training periods based on clinical testing rates and assess the sensitivity of model predictions to training periods. A testing period is classified as adequate when the rate of change in testing is greater than the rate of change in cases. We present a Bayesian deconvolution and linear regression model to estimate COVID-19 cases from WW data. The effective reproductive number is estimated from reconstructed cases using WW. The proposed modeling framework was applied to three Northern California communities served by distinct WW treatment plants. The results showed that training periods with adequate testing are essential to provide accurate projections of COVID-19 incidence.
更多查看译文
关键词
Bayesian inference,COVID–19,Public health surveillance: sewage,SARS-CoV-2,Statistical models,Wastewater-based epidemiology (WBE)
AI 理解论文
溯源树
样例
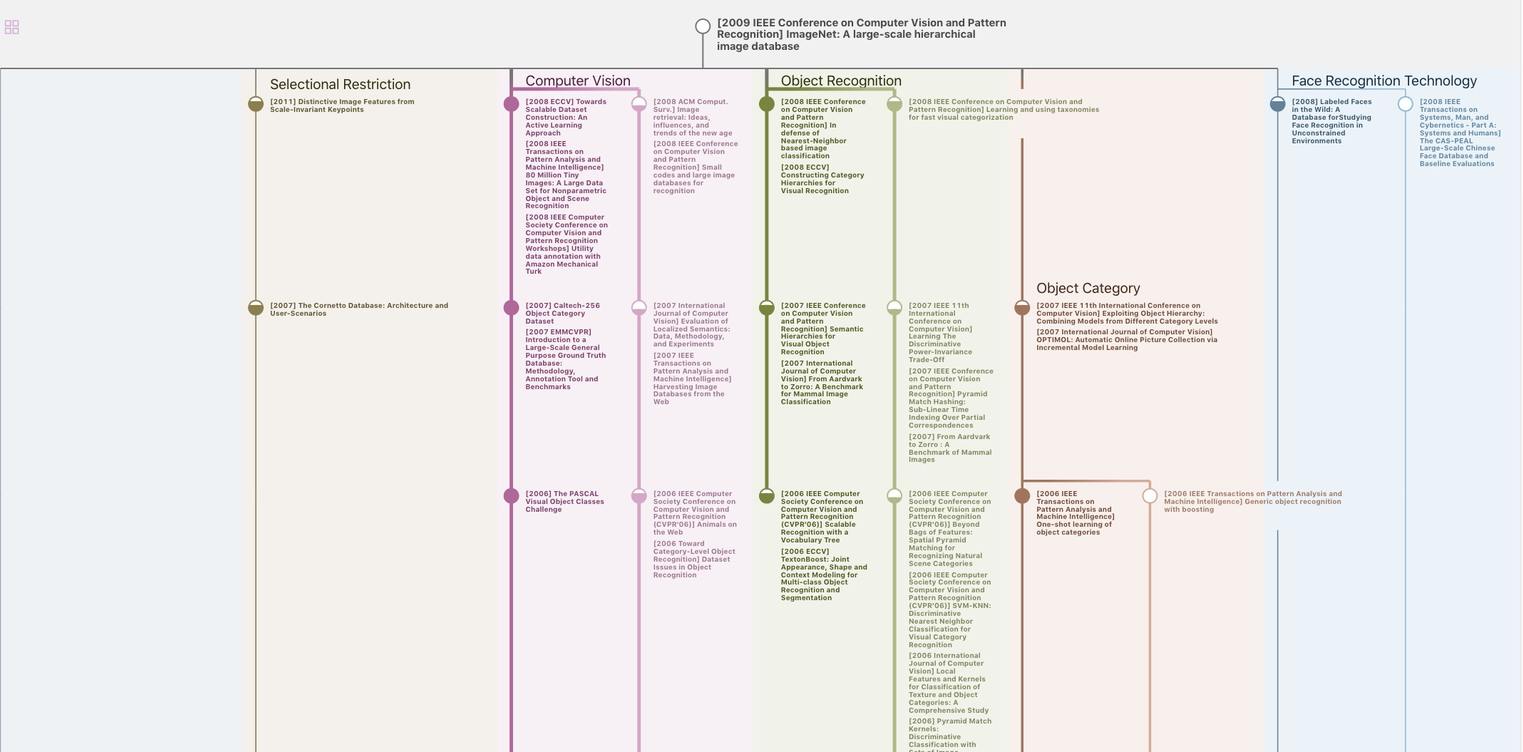
生成溯源树,研究论文发展脉络
Chat Paper
正在生成论文摘要