Two-stage salient object detection based on prior distribution learning and saliency consistency optimization
The Visual Computer(2022)
摘要
Although two-stage methods have favorably improved the accuracy and robustness of saliency detection, obtaining a saliency map with clear foreground boundaries and fine structure is still challenging. In this article, we proposed a novel and effective two-stage method to intelligently detect salient objects, which constitutes the coarse saliency map construction and the fine saliency map generation. Firstly, we develop the prior distribution learning algorithm (PDL) to explore the mapping relationship between input superpixel and its corresponding superpixels in various prior maps. The PDL can calculate the corresponding weights according to the contribution of various priors to each region in the image. Therefore, it can provide more reliable pseudo-labels for training subsequent learning models. Secondly, through learning the implicit representation between reliable samples and multiple priors, the learning model can accurately predict the salient values of those regions that are difficult to judge the saliency, so as to obtain an instructive coarse saliency map. Thirdly, in order to optimize the details of the coarse saliency map, we propose a framework called saliency consistency optimization, which can get clear foreground boundaries and effectively suppress the background noise. We compare the proposed algorithm with other state-of-the-art methods on four datasets. Experimental results adequately demonstrate the effectiveness of our approach over other comparison methods, especially two-stage-based methods.
更多查看译文
关键词
Salient object detection,Two-stage framework,Prior distribution learning,Learning process,Saliency consistency optimization
AI 理解论文
溯源树
样例
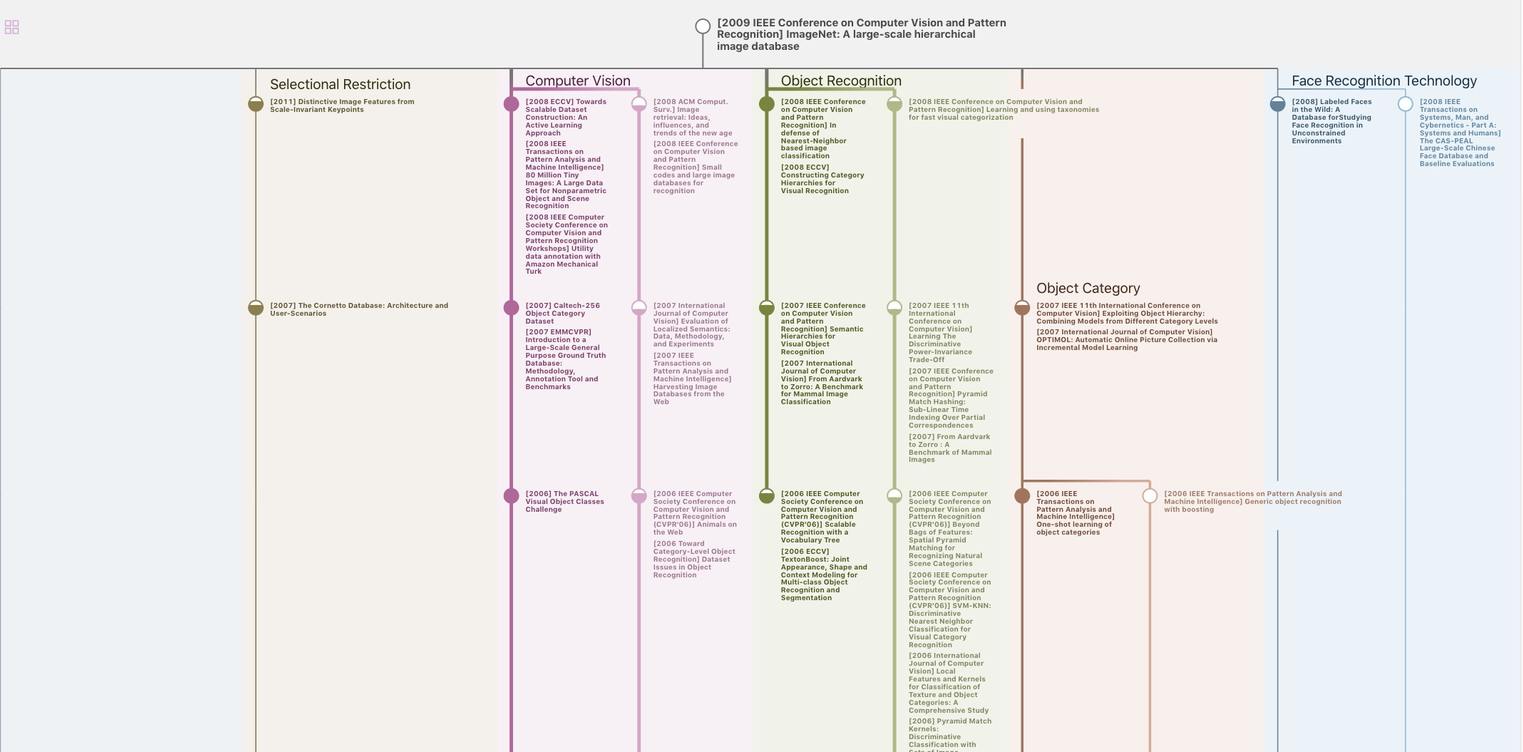
生成溯源树,研究论文发展脉络
Chat Paper
正在生成论文摘要