A Hybrid Preprocessor DE-ABC for Efficient Skin-Lesion Segmentation with Improved Contrast
Diagnostics(2022)
摘要
Rapid advancements and the escalating necessity of autonomous algorithms in medical imaging require efficient models to accomplish tasks such as segmentation and classification. However, there exists a significant dependency on the image quality of datasets when using these models. Appreciable improvements to enhance datasets for efficient image analysis have been noted in the past. In addition, deep learning and machine learning are vastly employed in this field. However, even after the advent of these advanced techniques, a significant space exists for new research. Recent research works indicate the vast applicability of preprocessing techniques in segmentation tasks. Contrast stretching is one of the preprocessing techniques used to enhance a region of interest. We propose a novel hybrid meta-heuristic preprocessor (DE-ABC), which optimises the decision variables used in the contrast-enhancement transformation function. We validated the efficiency of the preprocessor against some state-of-the-art segmentation algorithms. Publicly available skin-lesion datasets such as PH2, ISIC-2016, ISIC-2017, and ISIC-2018 were employed. We used Jaccard and the dice coefficient as performance matrices; at the maximum, the proposed model improved the dice coefficient from 93.56% to 94.09%. Cross-comparisons of segmentation results with the original datasets versus the contrast-stretched datasets validate that DE-ABC enhances the efficiency of segmentation algorithms.
更多查看译文
关键词
deep learning,machine learning,differential evolution,artificial bee colony,computer vision,skin-lesion segmentation
AI 理解论文
溯源树
样例
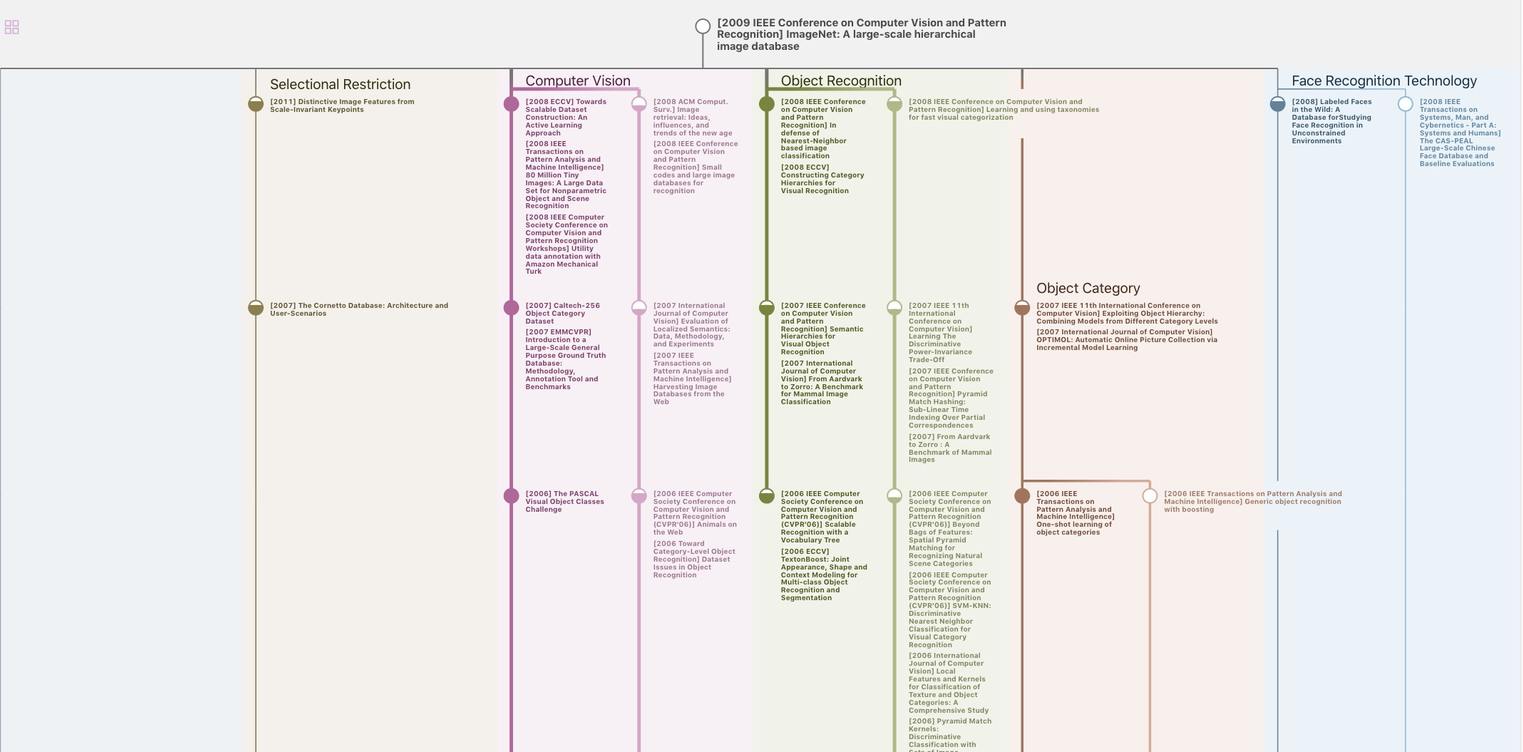
生成溯源树,研究论文发展脉络
Chat Paper
正在生成论文摘要