A neural network approach to solve geometric programs with joint probabilistic constraints
Mathematics and Computers in Simulation(2023)
摘要
We study a dynamical neural network approach to solve nonconvex geometric programs with joint probabilistic constraints (GPPC for short) with normally distributed coefficients and independent matrix row vectors. The main feature of our framework is to solve the biconvex deterministic equivalent problem of GPPC without the use of any convex approximation unlike the state-of-the-art solving methods. The second feature of our approach is to solve the dynamical system without the use of any iterative method but only by solving an ordinary differential equation system. We prove the stability together with the convergence of our neural network in the sense of Lyapunov. We also prove the equivalence between the optimal solution of the deterministic equivalent problem of GPPC and the solution of the dynamical system. We provide numerical experiments to show the performances of our approach compared to the state-of-art.
更多查看译文
关键词
Stochastic geometric programming,Joint probabilistic constraints optimization,Dynamical neural network,Lyapunov theory,ODE systems
AI 理解论文
溯源树
样例
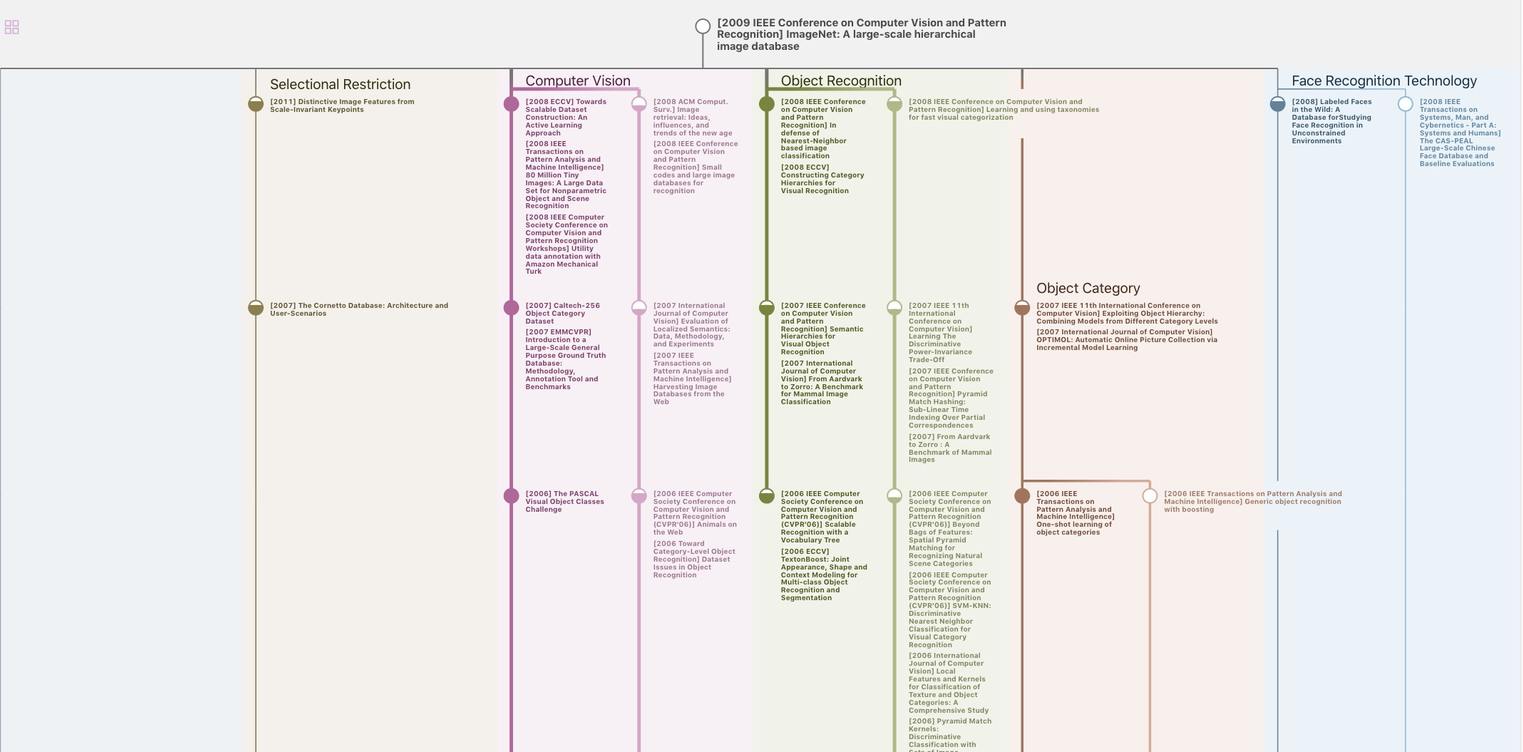
生成溯源树,研究论文发展脉络
Chat Paper
正在生成论文摘要