Cellular traffic prediction via deep state space models with attention mechanism
Computer Communications(2023)
摘要
Cellular traffic prediction is of great importance for operators to manage network resources and make decisions. Traffic is highly dynamic and influenced by many exogenous factors, which would lead to the degradation of traffic prediction accuracy. This paper proposes an end-to-end framework with two variants to explicitly characterize the spatiotemporal patterns of cellular traffic among neighboring cells. It uses convolutional neural networks with an attention mechanism to capture the spatial dynamics and Kalman filter for temporal modeling. Besides, we can fully exploit the auxiliary information such as social activities to improve prediction performance. We conduct extensive experiments on three real-world datasets. The results show that our proposed models outperform the state-of-the-art machine learning techniques in terms of prediction accuracy.
更多查看译文
关键词
Cellular traffic prediction,Kalman filter,Spatiotemporal dependencies,Auxiliary information,Attention mechanism
AI 理解论文
溯源树
样例
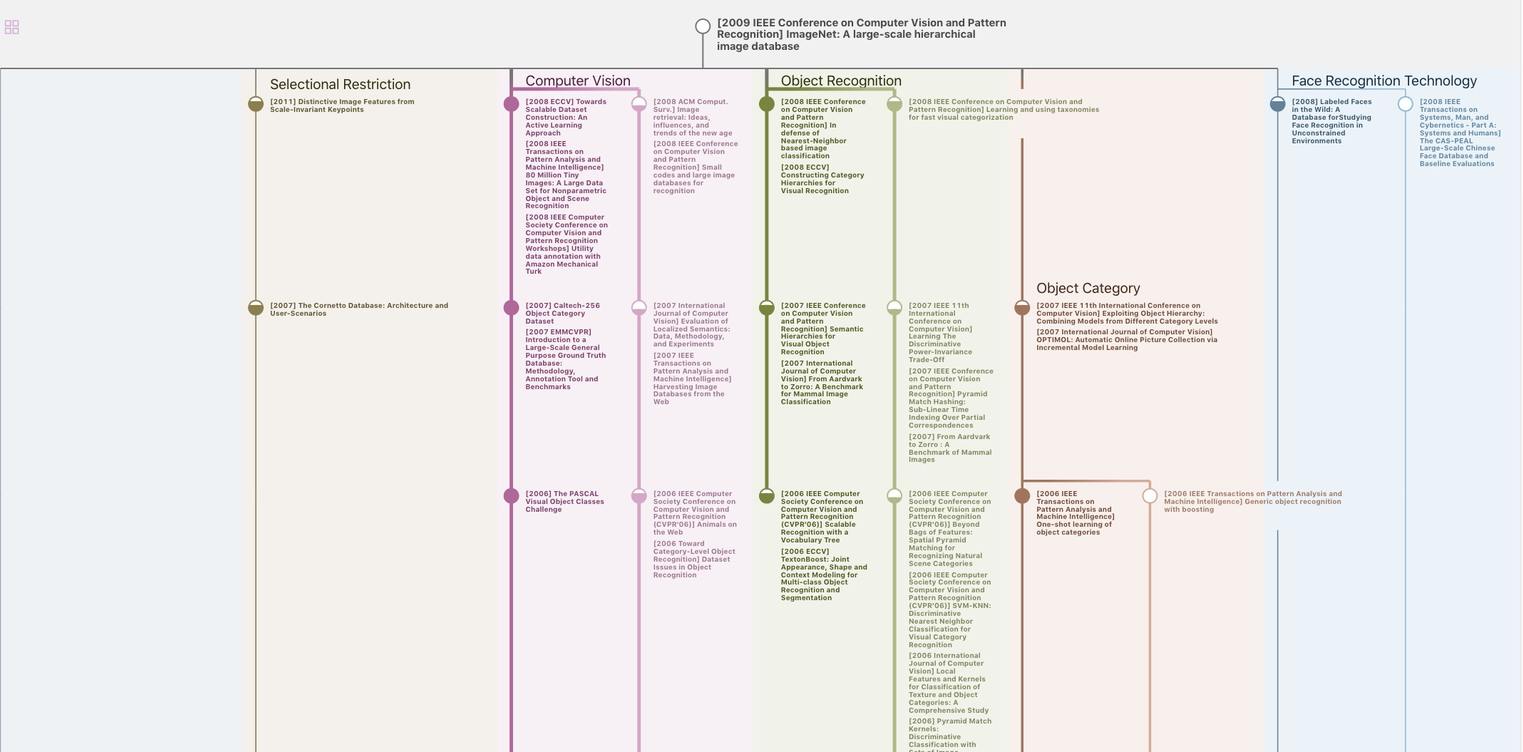
生成溯源树,研究论文发展脉络
Chat Paper
正在生成论文摘要