FedHD: Federated Learning with Hyperdimensional Computing
ACM International Conference on Mobile Computing and Networking(2022)
摘要
Federated Learning (FL) is a widely adopted distributed learning paradigm for to its privacy-preserving and collaborative nature. In FL, each client trains and sends a local model to the central cloud for aggregation. However, FL systems using neural network (NN) models are expensive to deploy on constrained edge devices regarding computation and communication. In this demo, we present FedHD, a FL system using Hyperdimensional Computing (HDC). In contrast to NN, HDC is a brain-inspired and lightweight computing paradigm using high-dimensional vectors and associative memory. Our measurements indicate that FedHD is 3.2x, 3.2x, 5x better on performance, energy and communication efficiency respectively compared to NN-based FL systems whilst maintaining similar accuracy to the state of the art. Our code is available on GitHub(1).
更多查看译文
AI 理解论文
溯源树
样例
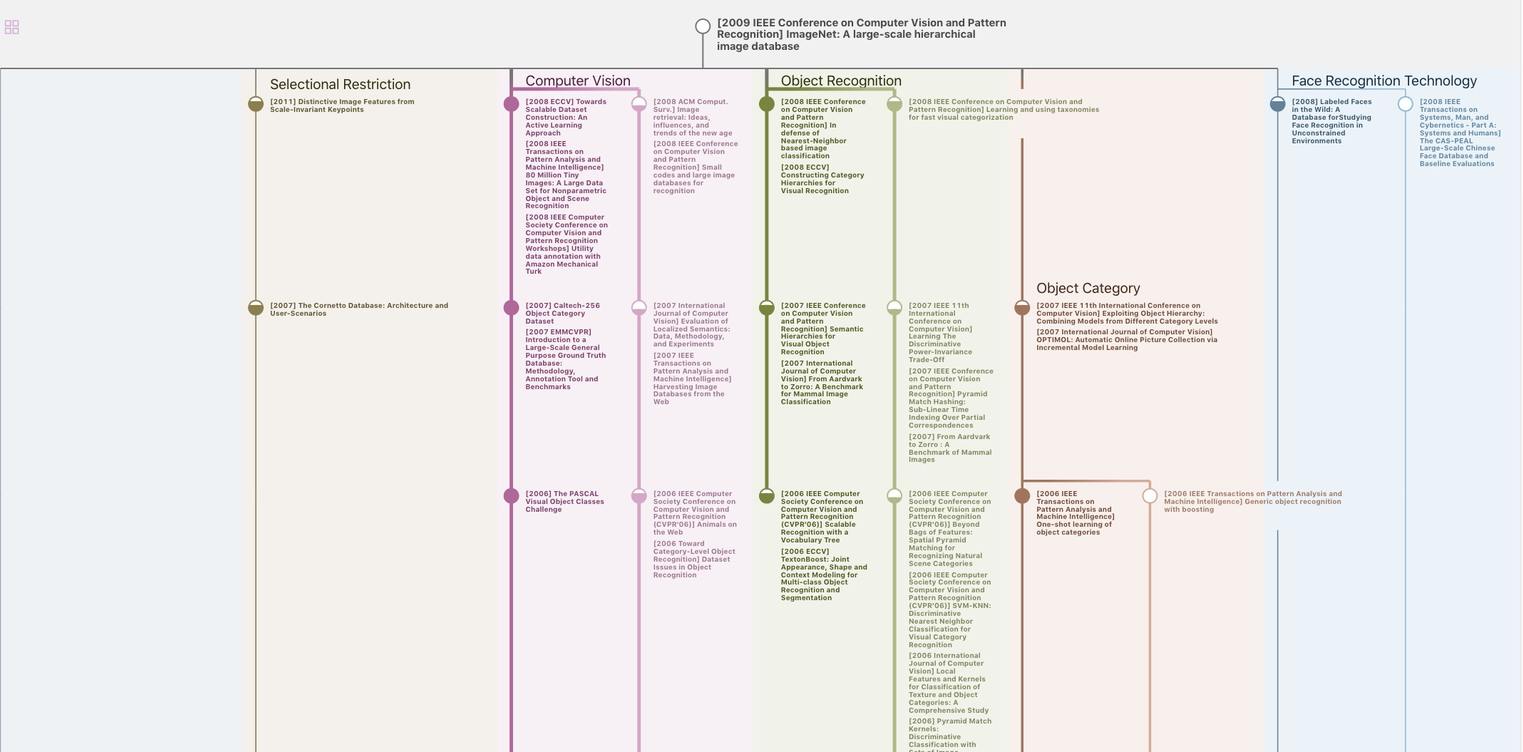
生成溯源树,研究论文发展脉络
Chat Paper
正在生成论文摘要