Ensemble Approach on Deep and Handcrafted Features for Neonatal Bowel Sound Detection
IEEE journal of biomedical and health informatics(2023)
摘要
For the care of neonatal infants, abdominal auscultation is considered a safe, convenient, and inexpensive method to monitor bowel conditions. With the help of early automated detection of bowel dysfunction, neonatologists could create a diagnosis plan for early intervention. In this article, a novel technique is proposed for automated peristalsis sound detection from neonatal abdominal sound recordings and compared to various other machine learning approaches. It adopts an ensemble approach that utilises handcrafted as well as one and two dimensional deep features obtained from Mel Frequency Cepstral Coefficients (MFCCs). The results are then refined with the help of a hierarchical Hidden Semi-Markov Models (HSMM) strategy. We evaluate our method on abdominal sounds collected from 49 newborn infants admitted to our tertiary Neonatal Intensive Care Unit (NICU). The results of leave-one-patient-out cross validation show that our method provides an accuracy of 95.1% and an Area Under Curve (AUC) of 85.6%, outperforming both the baselines and the recent works significantly. These encouraging results show that our proposed Ensemble-based Deep Learning model is helpful for neonatologists to facilitate tele-health applications.
更多查看译文
关键词
Pediatrics,Recording,Mel frequency cepstral coefficient,Feature extraction,Deep learning,Modeling,Data models,Bi-linear feature fusion,bowel sounds,deep learning,ensemble learning,machine learning,MFCC
AI 理解论文
溯源树
样例
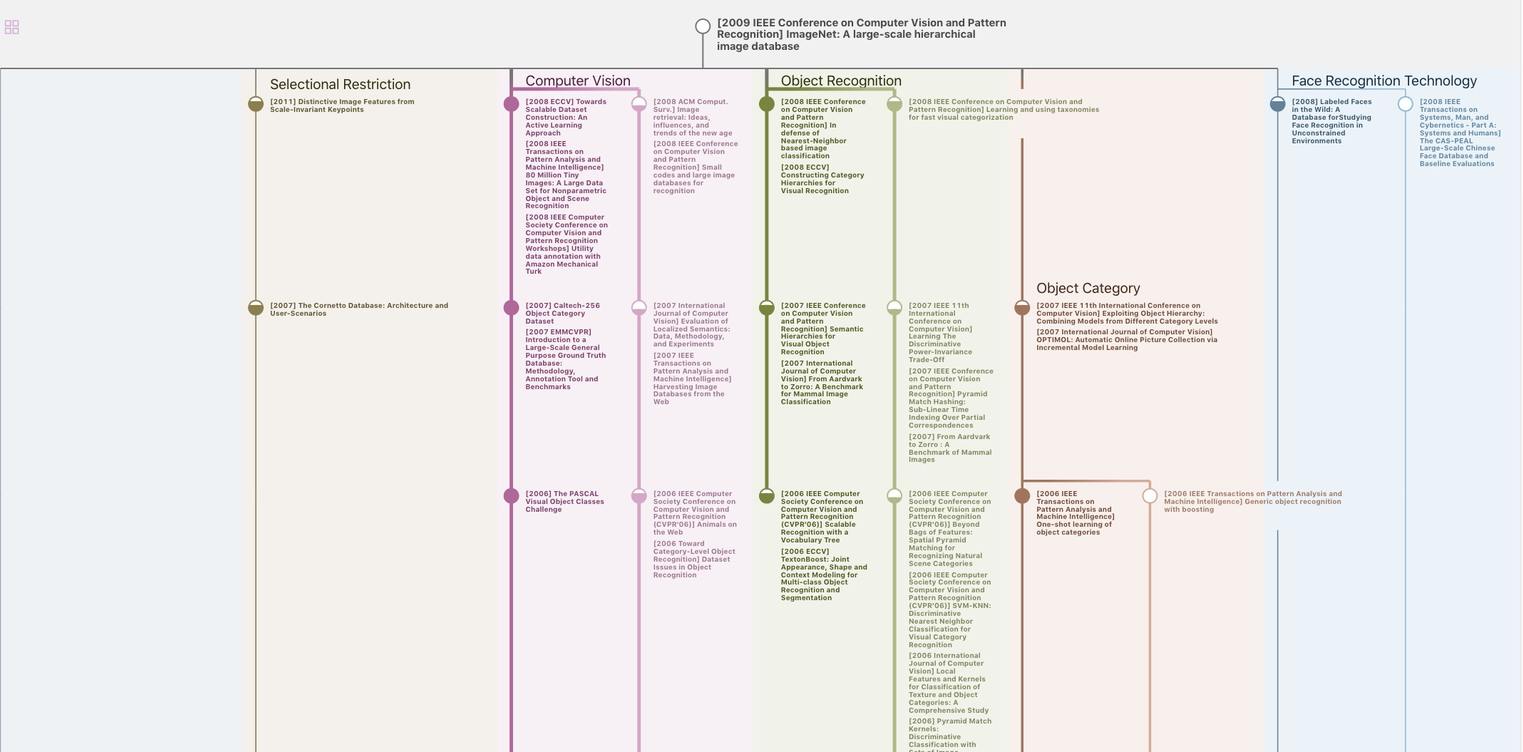
生成溯源树,研究论文发展脉络
Chat Paper
正在生成论文摘要