Automated Analysis of Sleep Study Parameters Using Signal Processing and Artificial Intelligence.
International journal of environmental research and public health(2022)
摘要
An automated sleep stage categorization can readily face noise-contaminated EEG recordings, just as other signal processing applications. Therefore, the denoising of the contaminated signals is inevitable to ensure a reliable analysis of the EEG signals. In this research work, an empirical mode decomposition is used in combination with stacked autoencoders to conduct automatic sleep stage classification with reliable analytical performance. Due to the decomposition of the composite signal into several intrinsic mode functions, empirical mode decomposition offers an effective solution for denoising non-stationary signals such as EEG. Preliminary results showed that through these intrinsic modes, a signal with a high signal-to-noise ratio can be obtained, which can be used for further analysis with confidence. Therefore, later, when statistical features were extracted from the denoised signals and were classified using stacked autoencoders, improved results were obtained for Stage 1, Stage 2, Stage 3, Stage 4, and REM stage EEG signals using this combination.
更多查看译文
关键词
EEG signals,autoencoders,biomedical signals,deep learning,sleep stage classification,sleep study
AI 理解论文
溯源树
样例
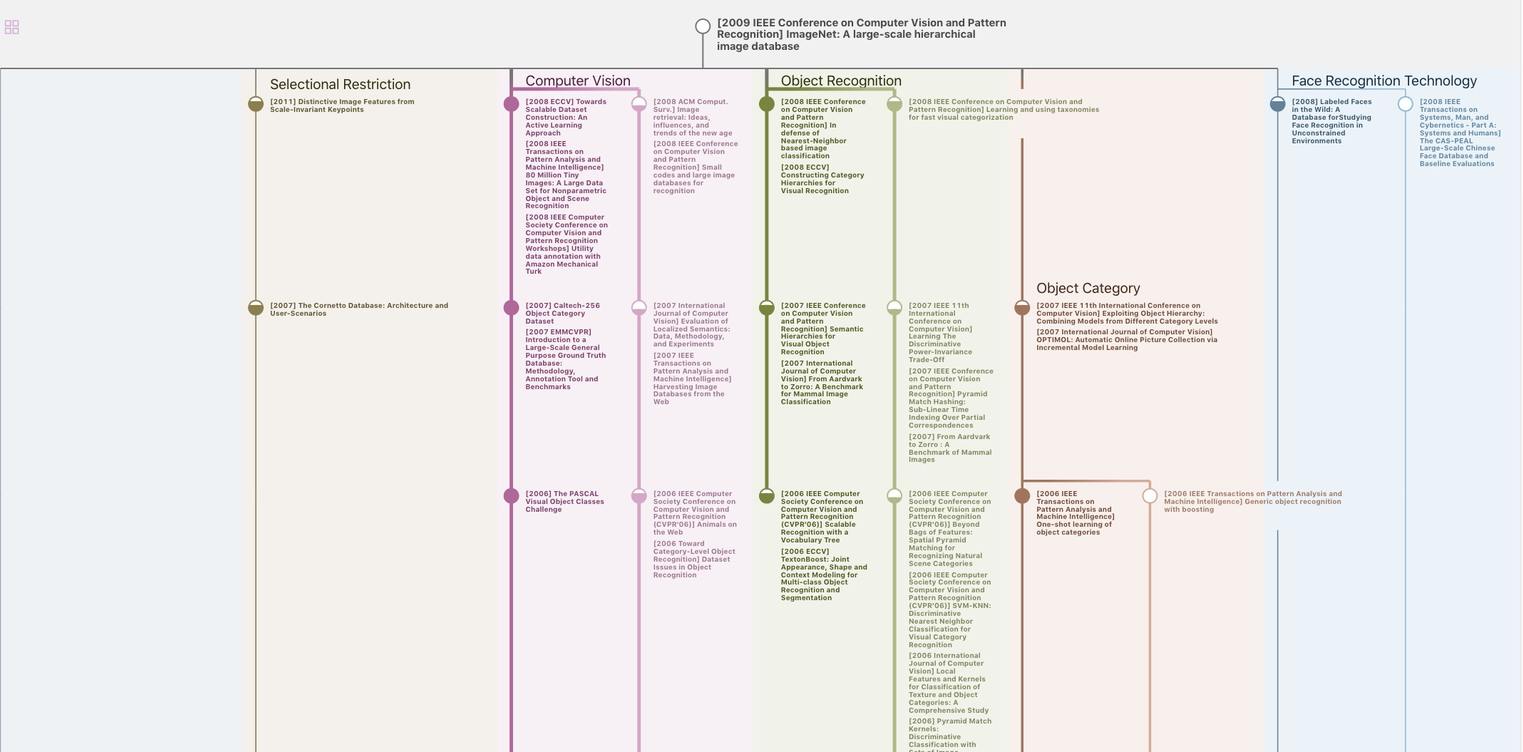
生成溯源树,研究论文发展脉络
Chat Paper
正在生成论文摘要