The Role of Machine Learning and Design of Experiments in the Advancement of Biomaterial and Tissue Engineering Research.
Bioengineering (Basel, Switzerland)(2022)
摘要
Optimisation of tissue engineering (TE) processes requires models that can identify relationships between the parameters to be optimised and predict structural and performance outcomes from both physical and chemical processes. Currently, Design of Experiments (DoE) methods are commonly used for optimisation purposes in addition to playing an important role in statistical quality control and systematic randomisation for experiment planning. DoE is only used for the analysis and optimisation of quantitative data (i.e., number-based, countable or measurable), while it lacks the suitability for imaging and high dimensional data analysis. Machine learning (ML) offers considerable potential for data analysis, providing a greater flexibility in terms of data that can be used for optimisation and predictions. Its application within the fields of biomaterials and TE has recently been explored. This review presents the different types of DoE methodologies and the appropriate methods that have been used in TE applications. Next, ML algorithms that are widely used for optimisation and predictions are introduced and their advantages and disadvantages are presented. The use of different ML algorithms for TE applications is reviewed, with a particular focus on their use in optimising 3D bioprinting processes for tissue-engineered construct fabrication. Finally, the review discusses the future perspectives and presents the possibility of integrating DoE and ML in one system that would provide opportunities for researchers to achieve greater improvements in the TE field.
更多查看译文
关键词
3d printing,Design of Experiment,biomaterials,machine learning,tissue engineering
AI 理解论文
溯源树
样例
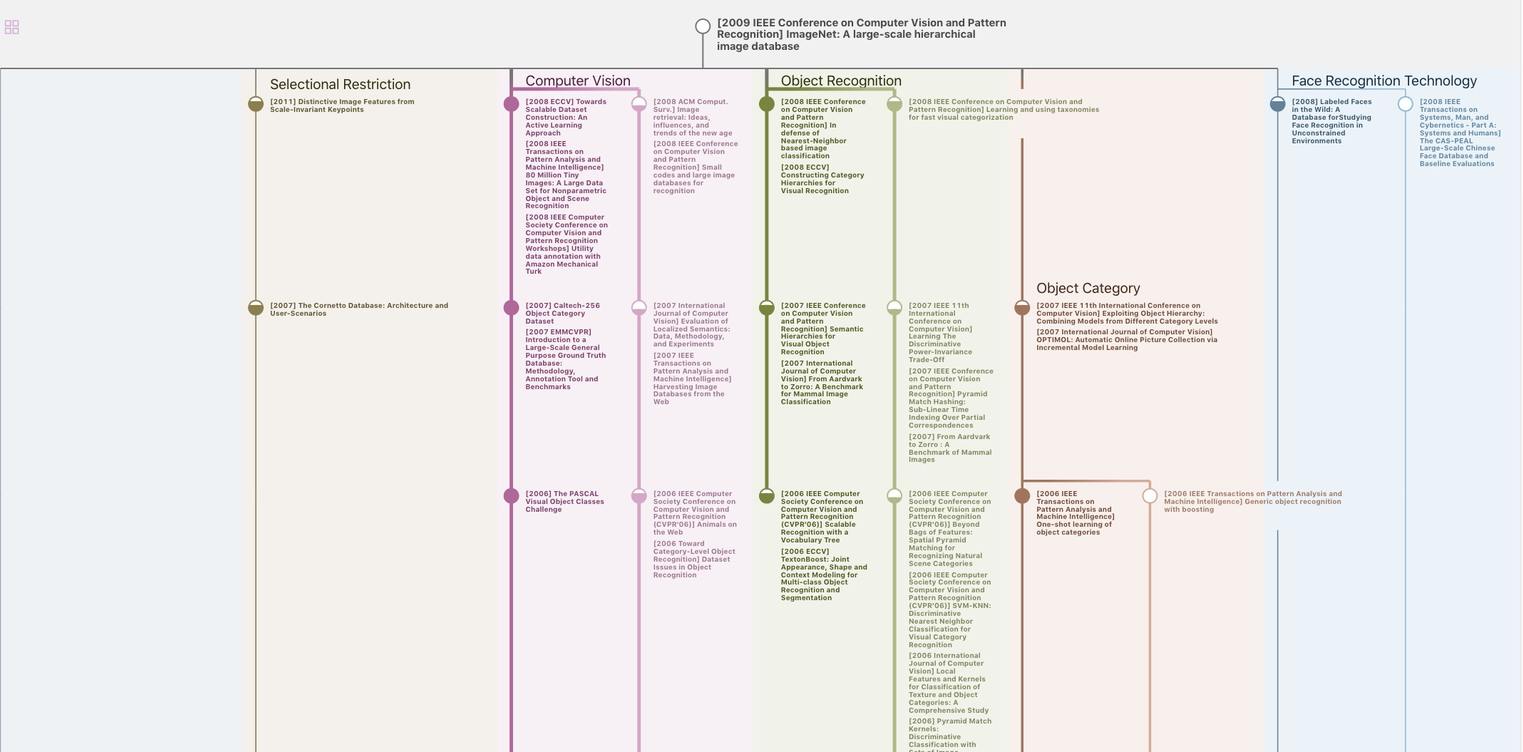
生成溯源树,研究论文发展脉络
Chat Paper
正在生成论文摘要