Salient object detection in low-light images via functional optimization-inspired feature polishing
Knowledge-Based Systems(2022)
摘要
Salient object detection under low-light conditions remains a challenge in many practical applications. Most recent works focus on feature integration without considering filtering out clutter from the darkness, thus limiting the detection performance. To tackle this issue, we propose a low-light image salient object detection network (LLISOD), which generates highly accurate saliency maps by functional optimization-inspired feature polishing strategy. The LLISOD includes: (1) An unfolded implicit nonlinear mapping (UINM) module uniquely designed for polishing feature maps; and (2) the hierarchical feature polishing (HFP) streams proposed for fusing the outputs of the UINM module on the top-down pathway to refine the saliency predictions. Furthermore, we provide a new dataset for benchmarking the investigation of salient object detection in low-light images. Extensive experiments demonstrate that our method outperforms existing state-of-the-art approaches. Code will be available at https://github.com/yuehuihui000/LLISOD.
更多查看译文
关键词
Low-light images,Salient object detection,Features polishing,Implicit nonlinear mapping,Functional optimization
AI 理解论文
溯源树
样例
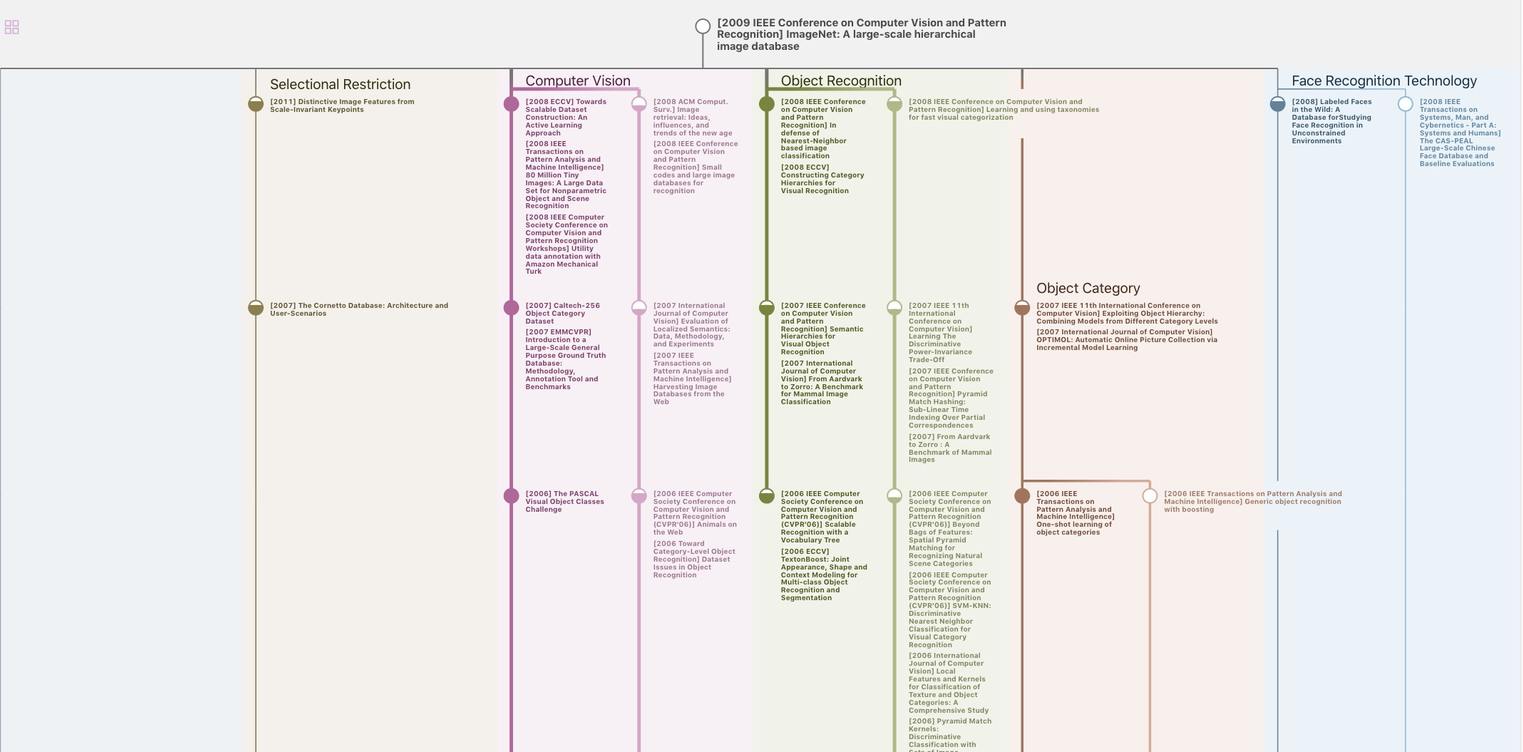
生成溯源树,研究论文发展脉络
Chat Paper
正在生成论文摘要