Multi-Mode Fiber-Based Speckle Contrast Optical Spectroscopy: Analysis of Speckle Statistics
biorxiv(2022)
摘要
Speckle contrast optical spectroscopy/tomography (SCOS/T) provides a real-time, non-invasive, and cost-efficient optical imaging approach to mapping of cerebral blood flow. By measuring many speckles (n>>10), SCOS/T has increased signal-to-noise ratio relative to diffuse correlation spectroscopy, which measures one or a few speckles. However, the current free-space SCOS/T designs are not ideal for large field-of-view imaging in humans because the curved head contour cannot be readily imaged with a single flat sensor and hair obstructs optical access. Herein we evaluate the feasibility of using cost-efficient multi-mode fiber (MMF) bundles for use in SCOS/T systems. One challenge with speckle contrast measurements is the potential for confounding noise sources (e.g., shot noise, readout noise) to contribute to the standard deviation measure and corrupt the speckle contrast measure that is central to the SCOS/T systems. However, for true speckle measurements, the histogram of pixel intensities from light interference follows a non-Gaussian distribution, specifically a gamma distribution with non-zero skew, whereas most noise sources have pixel intensity distributions that are Gaussian. By evaluating speckle data from static and dynamic targets imaged through MMF, we use histograms and statistical analysis of pixel histograms to evaluate whether the statistical properties of the speckles are retained. We show that flow-based speckle can be distinguished from static speckle and from sources of system noise through measures of skew in the pixel intensity histograms. Finally, we illustrate in humans that MMF bundles relay blood flow information. © 2022 Optica Publishing Group
### Competing Interest Statement
The authors have declared no competing interest.
更多查看译文
AI 理解论文
溯源树
样例
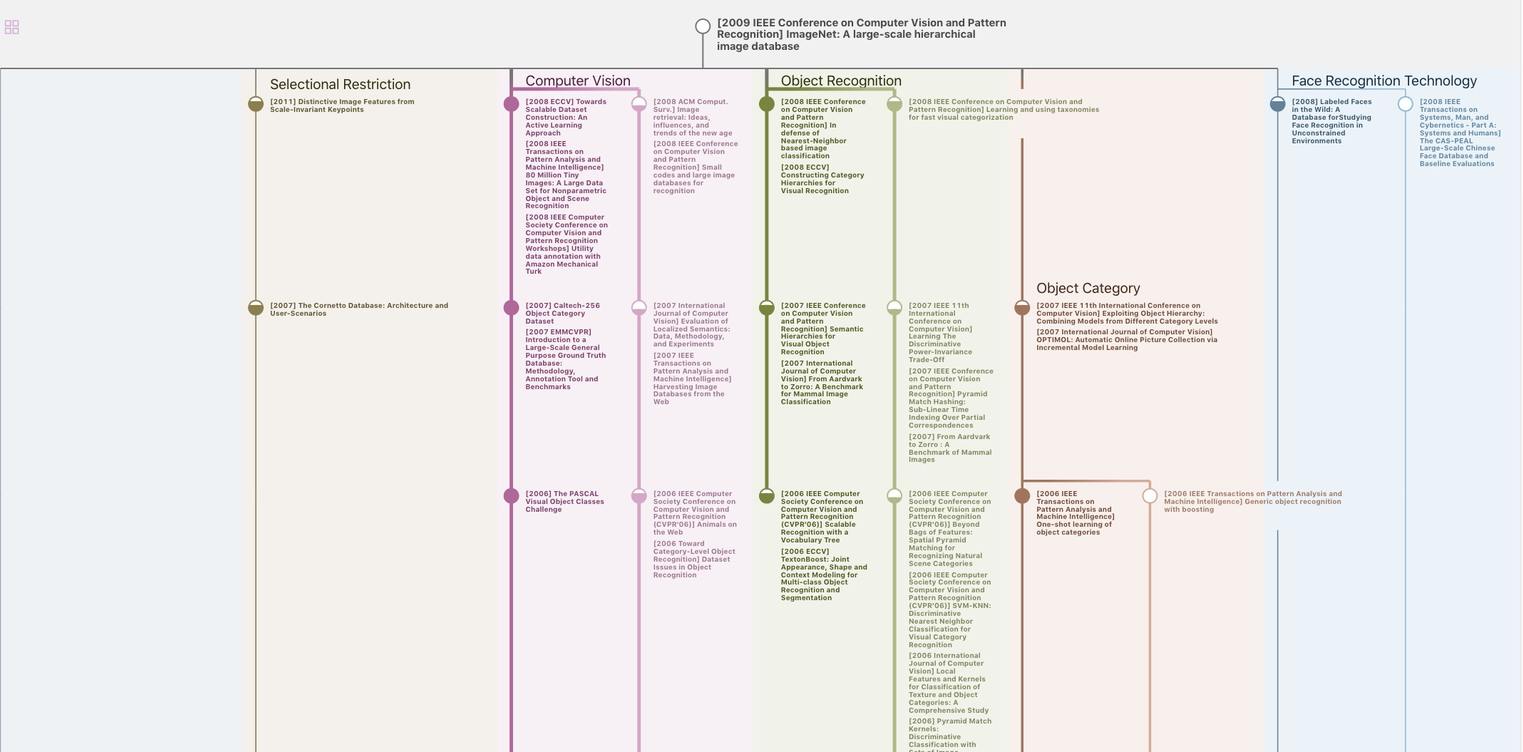
生成溯源树,研究论文发展脉络
Chat Paper
正在生成论文摘要